Claim Your Offer
Unlock a fantastic deal at www.statisticsassignmenthelp.com with our latest offer. Get an incredible 10% off on all statistics assignment, ensuring quality help at a cheap price. Our expert team is ready to assist you, making your academic journey smoother and more affordable. Don't miss out on this opportunity to enhance your skills and save on your studies. Take advantage of our offer now and secure top-notch help for your statistics assignments.
We Accept
- Understanding Descriptive vs. Inferential Statistics
- Descriptive Statistics: A Snapshot of Your Data
- Inferential Statistics: Drawing Conclusions Beyond the Data
- Practical Application: Calculating Descriptive Statistics in Google Sheets
- Step 1: Input Your Data
- Step 2: Calculate the Mean
- Step 3: Find the Median
- Step 4: Determine the Mode
- Step 5: Calculate the Range, Variance, and Standard Deviation
- Step 6: Analyze Your Results
- Calculating Descriptive Statistics for Different Data Types
- Numerical Data
- Categorical Data
- Ordinal Data
- The Importance of Statistics in Education: Enhancing Learning and Decision-Making
- Measuring Student Performance
- Evaluating Teaching Methods
- Informing Policy Decisions
- Promoting Equity in Education
- Enhancing Research and Innovation
- The Mean: Why This Statistical Measure Matters
- A Central Value
- A Basis for Comparison
- Understanding Variability
- Application in Real-World Scenarios
- Potential Pitfalls
- The Importance of Statistics: Ten Reasons to Embrace This Discipline
- Conclusion
Statistics is an essential discipline that plays a crucial role in a wide range of fields, from academic research to business analytics. The ability to understand and apply statistical concepts is invaluable, not only in the academic environment but also in everyday decision-making. This comprehensive guide will explore key statistical concepts, including the distinction between descriptive and inferential statistics, the practicalities of calculating descriptive statistics using tools like Google Sheets, and the significance of statistics in education. Additionally, it will delve into the importance of the mean as a central measure and offer ten compelling reasons why statistics is vital. Throughout this blog, we will also explain how mastering these concepts can help students solve their statistics assignment effectively, ensuring they are well-equipped to analyze data, draw meaningful conclusions, and present their findings with confidence.
Understanding Descriptive vs. Inferential Statistics
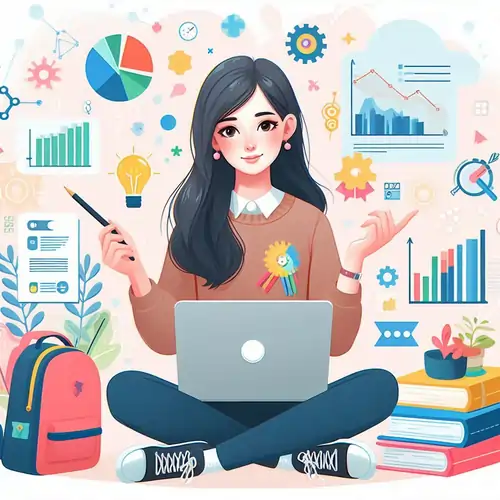
Statistics can broadly be divided into two main categories: descriptive and inferential statistics. Both are essential for data analysis, but they serve different purposes and are used in distinct ways.
Descriptive Statistics: A Snapshot of Your Data
Descriptive statistics provide a way to summarize and organize data in a meaningful way. When faced with a large dataset, descriptive statistics allow you to reduce the data to a simpler form without losing its essential characteristics. The key measures in descriptive statistics include the mean, median, mode, range, variance, and standard deviation.
- Mean: The mean is the average of a set of numbers. It provides a central value that represents the dataset. For example, if you're analyzing students' test scores, the mean score gives you a quick overview of the general performance of the class.
- Median: The median is the middle value when the data points are arranged in ascending or descending order. It is particularly useful when dealing with skewed data, as it is less affected by outliers than the mean.
- Mode: The mode is the most frequently occurring value in a dataset. It is useful in understanding the most common outcomes within the data.
- Range: The range measures the spread of the data by subtracting the smallest value from the largest. It gives a sense of the variability within the dataset.
- Variance and Standard Deviation: Variance measures how much the data points differ from the mean, while the standard deviation is the square root of the variance. These measures provide insights into the distribution and consistency of the data.
In the context of assignments, descriptive statistics are essential when you need to present data in a clear and concise manner. For example, if you are asked to analyze the performance of different student groups based on test scores, you would use descriptive statistics to summarize the data and highlight key trends. This summary helps in communicating complex data in a more understandable form, making it easier to draw preliminary conclusions.
Inferential Statistics: Drawing Conclusions Beyond the Data
While descriptive statistics summarize the data at hand, inferential statistics go a step further by making predictions or inferences about a larger population based on a sample of data. This branch of statistics is concerned with testing hypotheses and determining the probability that a given hypothesis is true.
- Hypothesis Testing: Hypothesis testing involves making an assumption (the null hypothesis) about a population parameter and then using sample data to test this assumption. For example, you might hypothesize that a new teaching method has no effect on students' performance. By analyzing a sample of test scores, you can use inferential statistics to determine whether the data supports or refutes this hypothesis.
- Confidence Intervals: A confidence interval gives a range of values that is likely to contain the population parameter. For instance, if you calculate a 95% confidence interval for the mean test score of a group, you can be 95% certain that the true mean of the population falls within this range.
- Regression Analysis: Regression analysis is used to model the relationship between variables. For example, you might use regression to predict students' final exam scores based on their attendance and participation throughout the semester.
In assignments that require you to make predictions or draw conclusions from data, inferential statistics are indispensable. They allow you to apply your findings from a sample to a larger population, adding depth and rigor to your analysis. By understanding and applying inferential statistics, you can provide evidence-based conclusions that are critical in both academic and professional contexts.
Practical Application: Calculating Descriptive Statistics in Google Sheets
Google Sheets is a versatile tool that offers a range of functions to calculate descriptive statistics. Its accessibility and ease of use make it an excellent choice for students and professionals alike. Here’s a step-by-step guide on how to calculate basic descriptive statistics using Google Sheets:
Step 1: Input Your Data
Start by entering your data into a column in Google Sheets. For example, if you are analyzing test scores, you might input each student’s score in column A.
Step 2: Calculate the Mean
To calculate the mean (average) of your data, use the =AVERAGE(range) function. For example, if your data is in cells A1 through A20, you would enter =AVERAGE(A1:A20) in another cell. Google Sheets will automatically compute the average score.
Step 3: Find the Median
To find the median, use the =MEDIAN(range) function. This will give you the middle value of your data, which is particularly useful if your data is skewed.
Step 4: Determine the Mode
The mode can be calculated using the =MODE(range) function. This function returns the most frequently occurring value in your dataset.
Step 5: Calculate the Range, Variance, and Standard Deviation
- Range: To calculate the range, subtract the minimum value from the maximum value. You can find the minimum using =MIN(range) and the maximum using =MAX(range).
- Variance and Standard Deviation: Google Sheets provides functions for both variance and standard deviation. Use =VAR(range) for variance and =STDEVP(range) for standard deviation. These measures help you understand the spread and consistency of your data.
Step 6: Analyze Your Results
Once you’ve calculated these statistics, take time to analyze them. What do they tell you about your data? Are there any trends or outliers? For example, if the standard deviation is high, it suggests that there is a wide variation in test scores among students. This insight can guide your interpretation and conclusions in your assignment.
By following these steps, you can efficiently calculate and analyze descriptive statistics in Google Sheets. This practical skill is invaluable for assignments that require data analysis, as it allows you to handle large datasets with ease and derive meaningful insights.
Calculating Descriptive Statistics for Different Data Types
While calculating descriptive statistics for simple numerical data is straightforward, things can get more complex when dealing with different data types, such as categorical or ordinal data. Let’s explore how to approach descriptive statistics for various data types:
Numerical Data
Numerical data, whether continuous or discrete, is the most common type of data analyzed using descriptive statistics. The steps outlined above for calculating the mean, median, mode, range, variance, and standard deviation are all applicable here.
Categorical Data
Categorical data refers to variables that can take on one of a limited, fixed number of categories, such as gender (male, female) or type of school (public, private). For categorical data, the most appropriate descriptive statistics are frequency counts and percentages.
- Frequency Counts: A frequency count shows how often each category occurs in your dataset. For example, if you’re analyzing the gender distribution in a class, you might count the number of males and females.
- Percentages: Percentages provide a way to express these frequencies relative to the total number of observations. For instance, if 60% of the students in your dataset are female, this is an important statistic that summarizes the data effectively.
Ordinal Data
Ordinal data represents variables with ordered categories, where the order matters, but the differences between categories are not necessarily uniform. An example is a survey where respondents rate their satisfaction on a scale of 1 to 5.
- Median and Mode: For ordinal data, the median and mode are commonly used. The median gives you the middle category, while the mode shows the most frequently occurring category.
- Range and Percentiles: The range can be calculated by subtracting the lowest category from the highest. Percentiles are also useful, especially when you want to see how a particular observation ranks within the dataset.
By understanding how to calculate descriptive statistics for different types of data, you can apply the appropriate methods in your assignments, ensuring accurate and meaningful analysis.
The Importance of Statistics in Education: Enhancing Learning and Decision-Making
Statistics plays a vital role in education, influencing everything from policy decisions to classroom practices. By analyzing data, educators and administrators can make informed decisions that improve student outcomes and enhance the overall quality of education.
Measuring Student Performance
One of the primary uses of statistics in education is to measure student performance. By analyzing test scores, attendance records, and other data, educators can identify trends, such as which students are excelling and which may need additional support. For example, descriptive statistics can be used to summarize the performance of a class on a particular test, highlighting the average score, the range of scores, and any outliers. This information is crucial for identifying areas where students are struggling and tailoring instruction to meet their needs.
Evaluating Teaching Methods
Statistics is also essential for evaluating the effectiveness of different teaching methods. For instance, if a school implements a new curriculum or instructional strategy, administrators can use inferential statistics to assess its impact on student achievement. By comparing test scores before and after the implementation, and controlling for other variables, educators can determine whether the new approach has led to significant improvements in learning outcomes.
Informing Policy Decisions
At the policy level, statistics is used to guide decisions about resource allocation, curriculum development, and other critical areas. For example, a school district might analyze graduation rates and standardized test scores to determine where additional resources are needed. Similarly, policymakers can use statistical analysis to evaluate the effectiveness of educational programs and initiatives, ensuring that funding is directed toward those that have the greatest impact on student success.
Promoting Equity in Education
Statistics also plays a key role in promoting equity in education. By analyzing data on student demographics, performance, and access to resources, educators and policymakers can identify disparities and work to address them. For example, if data shows that students from low-income backgrounds are underperforming compared to their peers, targeted interventions can be developed to support these students and close the achievement gap.
Enhancing Research and Innovation
Finally, statistics is a cornerstone of educational research, providing the tools needed to test hypotheses, analyze data, and draw conclusions. Research in education often involves complex data sets, and statistical methods are essential for making sense of this data and generating new insights. Whether it's studying the impact of technology in the classroom, exploring factors that influence student motivation, or developing new assessment tools, statistics is integral to advancing our understanding of education and improving practices.
Understanding the importance of statistics in education can enrich your assignments by providing a broader context for your analysis. When writing about educational topics, incorporating statistical evidence not only strengthens your arguments but also demonstrates your ability to apply quantitative methods to real-world problems. This skill is highly valued in both academic and professional settings, making it an essential component of your education.
The Mean: Why This Statistical Measure Matters
The mean, or average, is one of the most commonly used statistical measures, and for good reason. It provides a simple yet powerful way to summarize a set of data and is widely used in both academic research and everyday decision-making.
A Central Value
The mean is often referred to as a "measure of central tendency" because it represents a central value in a dataset. By calculating the mean, you get a single number that summarizes the entire dataset, making it easier to compare different sets of data or track changes over time. For example, if you're analyzing the performance of different student groups, the mean test score provides a quick way to see which group is performing better on average.
A Basis for Comparison
The mean is also useful for making comparisons. For instance, if you have two sets of test scores, calculating the mean for each set allows you to compare the overall performance of the two groups. This is particularly important in assignments that involve comparing different populations or examining the effects of an intervention. By using the mean, you can draw meaningful comparisons and support your conclusions with quantitative evidence.
Understanding Variability
While the mean provides a central value, it's also important to understand the variability in your data. This is where measures like the standard deviation come into play. The standard deviation shows how much the data points differ from the mean. If the standard deviation is small, it means that most of the data points are close to the mean, indicating consistency. If it's large, it suggests that there is a lot of variation in the data. Understanding this variability is crucial for interpreting the mean correctly and making informed decisions based on the data.
Application in Real-World Scenarios
The mean is widely used in various real-world scenarios, from business to healthcare. For example, businesses often use the mean to analyze sales data, track customer satisfaction, or evaluate employee performance. In healthcare, the mean is used to assess the effectiveness of treatments, monitor patient outcomes, and allocate resources. By mastering the concept of the mean, you can apply it to a wide range of contexts, both in your assignments and in your future career.
Potential Pitfalls
While the mean is a valuable tool, it's important to be aware of its limitations. The mean can be affected by outliers, or extreme values, which can skew the results. For example, if you're calculating the mean income of a group of people and one person has an exceptionally high income, this could distort the mean, making it higher than it would be otherwise. In such cases, it might be more appropriate to use the median, which is less sensitive to outliers. Being aware of these potential pitfalls and knowing when to use the mean (and when not to) is essential for accurate data analysis.
By understanding the significance of the mean and its applications, you can effectively incorporate this measure into your assignments. Whether you're analyzing test scores, comparing groups, or summarizing data, the mean provides a powerful tool for drawing meaningful conclusions and presenting your findings in a clear and concise manner.
The Importance of Statistics: Ten Reasons to Embrace This Discipline
Statistics is a fundamental discipline that permeates many aspects of life. From making informed decisions to driving innovation, the applications of statistics are vast and varied. Here are ten reasons why statistics is important and why it should be an integral part of your academic and professional toolkit:
- Informed Decision-Making: Statistics provide the data needed to make informed decisions in various fields. Whether you're a business leader, educator, or policymaker, understanding statistical data allows you to make choices that are backed by evidence rather than intuition.
- Understanding Trends: By analyzing data over time, statistics help identify trends and patterns. This is crucial for everything from predicting market behavior in business to monitoring public health trends.
- Predicting Outcomes: Statistical models can predict future events based on current data. For example, regression analysis can be used to forecast sales, project future growth, or estimate the impact of a new policy.
- Measuring Effectiveness: In education, business, and healthcare, statistics measure the effectiveness of strategies and interventions. By analyzing data before and after an intervention, you can assess its impact and make necessary adjustments.
- Quantifying Uncertainty: Statistics help quantify uncertainty and assess risks. This is especially important in fields like finance and insurance, where understanding risk is essential for making sound decisions.
- Enhancing Communication: Data-driven insights enhance communication by providing evidence-based arguments. Whether you're writing a report, giving a presentation, or engaging in a debate, statistical evidence adds credibility to your claims.
- Improving Processes: Statistical analysis identifies areas for improvement in processes and systems. For example, businesses use statistics to optimize production processes, reduce waste, and increase efficiency.
- Supporting Research: Statistics is the backbone of research, providing the tools for hypothesis testing and data analysis. Whether you're conducting a scientific study, exploring social trends, or developing new technologies, statistics is essential for generating and validating findings.
- Driving Innovation: In fields like technology, medicine, and engineering, statistics drives innovation by analyzing experimental data and identifying new opportunities for development.
- Empowering Individuals: Understanding statistics empowers individuals to critically evaluate information and make informed choices. In an age of data overload, the ability to interpret and analyze statistical information is more important than ever.
These ten reasons highlight the importance of statistics in various aspects of life and demonstrate why it is a critical skill to master. By embracing the discipline of statistics, you not only enhance your ability to complete assignments but also equip yourself with the tools needed to succeed in a data-driven world.
Conclusion
Statistics is more than just a collection of formulas and methods—it's a powerful tool for making sense of the world around us. By mastering the key concepts of descriptive and inferential statistics, learning how to calculate and interpret data using tools like Google Sheets, and understanding the significance of statistics in fields like education, you equip yourself with the skills necessary to excel in both academic and professional settings. The ability to analyze data, draw meaningful conclusions, and communicate your findings effectively is invaluable, not only for completing assignments but also for making informed decisions in everyday life.
This blog has provided you with a comprehensive guide to understanding and applying statistical concepts, ensuring you are well-prepared to tackle any statistics assignment with confidence. Whether you're summarizing data, testing hypotheses, or exploring the importance of statistics in education, the knowledge and skills you've gained here will serve as a strong foundation. By embracing the discipline of statistics, you not only enhance your academic performance but also position yourself as a critical thinker and problem-solver in a data-driven world.