Claim Your Offer
Unlock a fantastic deal at www.statisticsassignmenthelp.com with our latest offer. Get an incredible 20% off on your second statistics assignment, ensuring quality help at a cheap price. Our expert team is ready to assist you, making your academic journey smoother and more affordable. Don't miss out on this opportunity to enhance your skills and save on your studies. Take advantage of our offer now and secure top-notch help for your statistics assignments.
We Accept
Affordable Linear Programming Assignment Help
Embark on your academic journey with confidence, knowing our Linear Programming Assignment Help is not just exceptional but also budget-friendly. Our transparent pricing structure ensures fairness, considering factors like assignment complexity and deadlines. We offer competitive rates without compromising quality. To further enhance convenience, we provide various payment options, ensuring a secure and seamless transaction process. At statisticsassignmenthelp.com, we believe in providing top-notch assistance that aligns with your financial considerations. Your academic success is our priority, and our pricing reflects our commitment to accessibility and excellence.
Service Package | Price Range |
---|---|
Basic Package | $40 - $70 |
Standard Package | $70- $90 |
Premium Package | $90- $120 |
Customized Solutions | $120- $150 |
- What is Linear Programming?
- Importance of Linear Programming: A Strategic Tool
- Practical Uses of Linear Programming: Real-World Impact
- Why Opt for Linear Programming Assignment Help?
- Process for Accessing Our Linear Programming Assignment Help Services
- A Preferred Choice of Leading Universities Students
- Enhancing Your Experience Beyond Assignment Completion
What is Linear Programming?
Linear Programming (LP) stands as a cornerstone in the realm of mathematical optimization, offering a systematic approach to decision-making and resource allocation. At its core, LP deals with maximizing or minimizing a linear objective function while adhering to a set of linear constraints. This mathematical technique finds widespread application across diverse fields, revolutionizing the way problems are solved in business, economics, engineering, and operations research.
Importance of Linear Programming: A Strategic Tool
The significance of Linear Programming lies in its ability to streamline complex decision-making processes. By providing a structured framework for optimizing resources, LP empowers organizations to make informed choices, enhance efficiency, and achieve optimal outcomes. Its versatility extends to production planning, financial portfolio management, logistics, and beyond, making it an invaluable tool for professionals and decision-makers.
Practical Uses of Linear Programming: Real-World Impact
Linear Programming is not confined to theoretical concepts; its practical applications are profound. From supply chain management to financial planning, the technique is instrumental in designing efficient systems, minimizing costs, and maximizing profits. LP tackles real-world challenges, aiding in the allocation of resources, workforce scheduling, and even resolving complex network flow problems.
Understanding Linear Programming is not just an academic pursuit; it's a gateway to unlocking optimization potential in the real world, shaping smarter decisions, and fostering operational excellence. Explore the multifaceted realm of LP and witness its transformative impact on problem-solving across various industries.
Why Opt for Linear Programming Assignment Help?
Navigating through Survey Methodology assignments can be a challenging task for students, often demanding a deep understanding of mathematical concepts and intricate problem-solving skills, which makes students think "Who will help me to do my Survey Methodology Assignment." Here's why opting for a specialized Survey Methodology Assignment Help service at statisticsassignmenthelp.com can be a game-changer for students. Our customer support team is available 24/7 via live chat, ensuring that students can feel free to contact us anytime they encounter assignment problems. We guarantee timely delivery of assignments, assisting students in meeting their deadlines and achieving academic success. With our team of experienced writers, you can trust us to deliver high-quality academic writing at an affordable price. Don't let the complexities of Survey Methodology projects overwhelm you; let us guide you through with our online Survey Methodology assignment help services:
- Achieve Top Grades:
- Benefit from the expertise of professionals who have mastered the intricacies of Linear Programming.
- Ensure accuracy and precision in your assignments, leading to higher grades and academic success.
- Timely Submission:
- Meet tight deadlines with confidence as assignment help with Linear Programming Assignment services and prioritize timely delivery.
- Eliminate the stress of last-minute rushes, allowing for thorough reviews before submission.
- Concept Clarity:
- Gain a comprehensive understanding of Linear Programming concepts through expertly crafted solutions.
- Clarify doubts and enhance your knowledge, paving the way for improved performance in exams and future applications.
- Personalized Assistance:
- Receive customized solutions tailored to your specific assignment requirements.
- Address unique challenges with the help of professionals who understand the nuances of Linear Programming problems.
- Reduce Stress and Workload:
- Delegate the complexity of Linear Programming assignments to professionals, allowing you to focus on other academic and personal commitments.
- Experience a reduction in stress levels, promoting a healthier and more balanced approach to your studies.
- Learn from Experts:
- Absorb valuable insights and methodologies used by seasoned professionals in the field of Linear Programming.
- Leverage the experience of experts to enhance your problem-solving skills and overall academic performance.
Process for Accessing Our Linear Programming Assignment Help Services
Navigating through our Linear Programming Assignment Help services is a seamless process designed to provide you with tailored solutions and Linear Programming Assignment expert assistance. If you find yourself grappling with the Linear Programming Assignment, you can confidently tackle any academic task just by sending a "Do My Linear Programming Assignment" request. Here's a step-by-step guide on how to make the most of our help with Linear Programming Assignment services:
- Submit Your Assignment Details: Begin by submitting the details of your Linear Programming assignment. Use our user-friendly submission form to provide information on the topic, specific requirements, and any additional instructions.
- Receive a Competitive Quote: Shortly after submitting your assignment details, our system will generate a competitive quote for the services required. This transparent pricing ensures clarity with no hidden costs.
- Confirm Your Order: Once you've reviewed the quote, proceed to confirm your order by making a secure online payment. We offer a variety of payment options for your convenience.
- Assignment Allocation: Upon confirmation, our system will allocate your assignment to a qualified Linear Programming Assignment expert with expertise in Linear Programming. Rest assured that your assignment is in capable hands.
- Track Progress and Communicate: Stay informed about the progress of your assignment. Our platform allows you to track the status of your order and communicate directly with the assigned Linear Programming Assignment expert for any clarifications or updates.
- Receive Completed Assignment: Experience the satisfaction of timely delivery as we ensure your completed assignment is sent to you well before the agreed deadline. This provides you with ample time to review the solution and seek any necessary revisions.
A Preferred Choice of Leading Universities Students
Join the ranks of successful students from renowned institutions globally who have entrusted their survey methodology assignments to our survey methodology Assignment Help experts. Our commitment to excellence and personalized assistance has made the Statistics Assignment Help service a preferred choice among students from prestigious universities, including but not limited to. With our expertise, you can understand the concepts of survey methodology and excel in your linear programming projects. Our online linear programming services ensure that you receive your assignment on time without compromising on quality. Trust us for top-notch writing services tailored to your needs.
- Fordham University
- University of New Mexico
- Drexel University
- Louisiana Tech University
Our reputation for delivering high-quality, tailored solutions has earned the trust of students pursuing academic excellence in diverse fields. Whether you're enrolled in a top-tier university or any other educational institution, our Help with Linear Programming Assignment services at statisticsassignmenthelp.com are designed to meet the rigorous standards set by leading academic programs. Join the league of satisfied students who have experienced the difference with our trusted Linear Programming Assignment Help services.
Enhancing Your Experience Beyond Assignment Completion
Completing your Linear Programming assignment with Statistics Assignment Help goes beyond receiving a well-crafted solution. We believe in rewarding your trust and continued partnership through a range of post-assignment benefits designed to make your academic journey even more fulfilling:
- Loyalty Rewards: Our loyalty program is our way of thanking you for choosing Statistics Assignment Help. Earn points with every completed assignment, and redeem them for exclusive discounts, free services, or even personalized assistance on future tasks.
- Referral Bonuses: Spread the word about our exceptional Help with Linear Programming Assignment services and reap the rewards. Refer your friends and classmates, and enjoy exclusive referral bonuses, including discounts on your next assignment or additional services at no cost.
- Discounts for Returning Clients: We value your continued trust. Returning clients enjoy special discounts on subsequent assignments as a token of appreciation for choosing our help with Linear Programming Assignment services once again.
Explore Linear Programming Insights: Our Informative Blogs
Dive into the world of Linear Programming with our insightful blogs that go beyond assignments. Our dedicated team of experts at statisticsassignmenthelp.com shares valuable knowledge, practical tips, and in-depth analyses to enrich your understanding of LP concepts. Explore the dynamic landscape of Linear Programming through our engaging blogs, crafted with expertise and a passion for fostering learning. Welcome to a reservoir of knowledge that goes beyond traditional assignment help!
Student Success Stories with Our Linear Programming Assignment Help
Discover the impact of our Linear Programming Assignment Help through the voices of satisfied students. Our reviews at statisticsassignmenthelp.com narrate tales of academic triumph, where our expert assistance has not only met but exceeded expectations. Students from diverse backgrounds commend our precision, timely deliveries, and personalized approach. Their success stories echo the commitment and dedication of our team in ensuring top-notch assistance.
Meet Our Expert Team in Linear Programming Assignment Help
Our dedicated team of Linear Programming Assignment experts at statisticsassignmenthelp.com is the backbone of our exceptional Linear Programming Assignment Help services. Armed with advanced degrees and extensive experience in the field, our Linear Programming Assignment experts bring unparalleled knowledge and precision to every assignment. They specialize in tackling complex Linear Programming problems, providing clear explanations, and offering step-by-step solutions that not only meet academic requirements but also enhance your understanding of the subject.
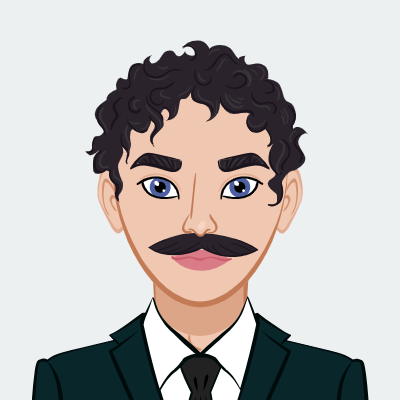
Dr. Evelyn
PhD in Statistics
🇺🇸 United States
Related Topics
Frequently Asked Questions (FAQs)
Curious about how our Linear Programming Assignment Help works? Delve into our FAQs for comprehensive insights. Learn about our submission process, turnaround times, and how we ensure the quality of your assignments. Discover the details of our pricing structure, loyalty programs, and referral bonuses. Uncover the steps to track your assignment's progress and communicate with our Linear Programming Assignment helper team. If you're new to seeking assignment help or have specific queries about our Statistics Assignment Help services, our FAQs section provides clarity and transparency. Navigate your academic journey confidently with the information you need at your fingertips.
Our pricing is transparent and competitive. Factors such as assignment complexity, deadline, and any specific requirements contribute to the quoted amount.