Claim Your Offer
Unlock an exclusive deal at www.statisticsassignmenthelp.com with our Spring Semester Offer! Get 10% off on all statistics assignments and enjoy expert assistance at an affordable price. Our skilled team is here to provide top-quality solutions, ensuring you excel in your statistics assignments without breaking the bank. Use Offer Code: SPRINGSAH10 at checkout and grab this limited-time discount. Don’t miss the chance to save while securing the best help for your statistics assignments. Order now and make this semester a success!
We Accept
Explore Poisson Process Assignment Samples for Expert Guidance
Access our sample Poisson Process assignments to enhance your understanding and skills. Our expertly crafted samples provide comprehensive insights into tackling Poisson Process problems effectively. From analyzing data patterns to predicting outcomes, our samples offer step-by-step solutions and explanations. Explore various scenarios and learn how to apply Poisson Process concepts in real-world situations.
Statistics
Statistics
Statistics
Statistics
Statistics
Statistics
Statistics
Statistics
Statistics
Statistics
Statistics
Statistics
Statistics
Statistics
Statistics
Statistics
Statistics
Statistics
Statistics
Statistics
Explore Our Transparent Pricing Model for Expert Poisson Process Assignment Help
Unlock the power of precise Poisson Process assignment support with our transparent pricing structure. We believe in clarity, ensuring you understand the value you receive. Our expert assistance guarantees top-notch solutions, and our transparent pricing model eliminates any uncertainties. Experience a seamless process, knowing exactly what you pay for exceptional Poisson Process assignment help. Your academic success is our priority, and transparency is the key.
Service | Description | Price Range | Turnaround Time |
---|---|---|---|
Basic Data Analysis in Poisson Processes | (Data Entry, Initial Analysis) | $20 - $40 | 24-48 hours |
Intermediate Poisson Process Analysis | (Hypothesis Testing, Descriptive Stats) | $45 - $75 | 2-4 days |
Advanced Poisson Process Modeling | (Regression, ANOVA, Time Series) | $80 - $120 | 5-7 days |
Complex Poisson Process Simulations | (Monte Carlo Simulations, Advanced Techniques) | $150 - $250+ | 10 days - 1 month |
Rapid Service for Urgent Assignments | Additional 50% | - | 12-24 hours |
- What is Poisson Process Assignment Help?
- Unlocking Success: Why Invest in Poisson Process Assignment Help for Students?
- Students from Diverse Universities Benefiting from Our Services
- Diverse Topics Covered in Poisson Process Assignment Help Services
- Engaging Poisson Process Assignment Help: Streamlined Process
What is Poisson Process Assignment Help?
Poisson Process Assignment Help refers to specialized assistance provided to students seeking support with assignments related to Poisson Processes, a fundamental concept in probability theory and stochastic processes. Poisson Process Assignment Help experts offer personalized guidance from experienced tutors, ensuring clarity in concepts and improving problem-solving skills. By leveraging expert assistance, students can navigate through the intricacies of Poisson Processes with confidence, leading to improved academic performance and a deeper understanding of this critical mathematical concept. Whether students require help with Poisson Process Assignment on basic concepts or advanced analytical techniques like regression analysis or multivariate modeling, StatisticsAssignmentHelp.com services provide tailored solutions to meet their specific academic needs. Additionally, these services often offer transparent pricing models and efficient turnaround times, enhancing the overall experience for students seeking assistance with Poisson Process assignments. Ultimately, the Poisson Process Assignment Helper aims to empower students to overcome challenges, master complex concepts, and achieve success in their academic endeavors.
Poisson Process Assignment Help is your dedicated resource for conquering challenges related to Poisson Processes in probability theory and stochastic processes. Our expert tutors provide personalized guidance, ensuring clarity in concepts and honing problem-solving skills. We go beyond mere assistance in arrival times understanding; our team specializes in fostering excellence in assignment writing. With a commitment to delivering top-notch statistics assignment solutions, we prioritize timely completion, ensuring your assignments on time submission. Whether you're grappling with complex statistics problems or navigating through your academic journey, our tailored solutions empower you to overcome challenges and excel in mastering the intricate concepts of Poisson Processes.
Unlocking Success: Why Invest in Poisson Process Assignment Help for Students?
Poisson Process Assignment Help is an invaluable resource for students grappling with the complexities of Poisson Process assignments. The intricate landscape of Poisson Processes can often be daunting, making professional assistance essential. By investing in expert guidance from seasoned mathematicians and statisticians, students can save valuable time and deepen their understanding of intricate concepts. Tailored solutions aligned with students' academic levels ensure enhanced learning outcomes, while professional assistance in coding fosters mastery of essential programming languages. With assured confidentiality, affordability, and round-the-clock support, Poisson Process Assignment Helper empowers students to excel academically and confidently apply advanced tools and methodologies in their coursework.
- Time Constraints: Students, often juggling multiple courses and commitments, seek help to save time on complex Poisson Process assignments.
- Understanding Complex Concepts: Investing ensures a deeper comprehension of intricate Poisson Process concepts, improving overall learning outcomes.
- Expert Guidance: Access to experienced mathematicians and statisticians provides valuable insights and guidance beyond what textbooks offer.
- Improved Grades: Seeking professional assistance contributes to better assignment quality, potentially boosting grades and overall academic performance.
- Application of Real-World Skills: Learn how to apply Poisson Process skills in real-world scenarios, enhancing practical knowledge.
- Coding Proficiency: For assignments involving programming, students benefit from expert assistance to improve coding skills in relevant languages.
- Meeting Academic Standards: Professional help ensures that assignments meet academic standards, reducing stress and ensuring compliance with course requirements.
- Confidence Building: Success in assignments builds confidence, motivating students to actively engage with and excel in Poisson Process coursework.
- Time for Other Commitments: Outsourcing assignments allows students to allocate time to other crucial aspects of their academic and personal lives.
- Future Career Prospects: A solid understanding of Poisson Processes, facilitated by expert help, enhances students' prospects in future careers involving data analysis and statistics.
Students from Diverse Universities Benefiting from Our Services
At our statistics assignment help service, we take pride in assisting students from a wide range of universities, catering to their unique academic needs and ensuring their success in probability theory and stochastic processes. Here are some of the universities from which students have availed our services:
- University of Nebraska at Kearney (UNK): Students from UNK trust our expertise to provide them with comprehensive assistance in understanding and completing their Poisson Process assignments.
- Ohio Wesleyan University: Students from Ohio Wesleyan University rely on our professional guidance to navigate through the complexities of probability theory and excel in their coursework.
- University of North Alabama (UNA): At UNA, students benefit from our tailored solutions and expert support to enhance their understanding of Poisson Processes and achieve academic success.
- Clark Atlanta University: Students from Clark Atlanta University turn to us for assistance in tackling challenging assignments and mastering the concepts of probability theory with confidence.
- Tuskegee University: At Tuskegee University, students trust our dedicated team to provide them with personalized guidance and support, enabling them to excel in their studies.
- University of Southern Mississippi: Students from the University of Southern Mississippi rely on our professional assistance to overcome obstacles and achieve their academic goals in probability theory.
- Indiana University Northwest: At Indiana University Northwest, students benefit from our reliable services, ensuring timely completion of assignments and a deeper understanding of Poisson Processes.
- Arkansas State University: Students at Arkansas State University count on our expertise to provide them with quality assistance and help them succeed in their probability theory coursework.
- Morgan State University: Students from Morgan State University trust our team to deliver top-notch solutions and guidance, enabling them to excel in their studies and achieve academic excellence.
- Bowie State University: At Bowie State University, students turn to us for professional support and expert assistance in mastering the intricacies of probability theory and stochastic processes.
Through our dedicated efforts and personalized approach, we continue to serve students from diverse universities, offering expert assistance with Poisson Process assignments. Our aim is to help them overcome academic challenges and succeed in their academic endeavors with confidence.
Diverse Topics Covered in Poisson Process Assignment Help Services
Our Statistics Assignment Help services offer comprehensive assistance to students seeking support in various topics related to probability theory and stochastic processes. Here are some of the diverse topics we cover:
- Homogeneous Poisson Process: Understanding the concept of a Homogeneous Poisson Process, its properties, and applications in modeling random events occurring over time or space.
- Non-Homogeneous Poisson Process: Exploring the Non-Homogeneous Poisson Process and its implications, including varying event rates over time or space.
- Compound Poisson Process: Learning about the Compound Poisson Process, which combines a Poisson Process with random magnitudes for each event, and its significance in modeling phenomena such as insurance claims and earthquakes.
- Renewal Theory: Delving into Renewal Theory, a branch of probability theory closely related to Poisson Processes, and its applications in analyzing stochastic processes with intermittent arrivals.
- Queueing Theory: Understanding Queueing Theory and its connection to Poisson Processes, particularly in modeling the arrival process of customers or tasks in systems such as telecommunication networks and computer systems.
- Reliability Theory: Exploring the application of Poisson Processes in Reliability Theory, including modeling the failure and repair processes of systems to assess their reliability and availability.
- Markov Processes: Studying Markov Processes and their relationship with Poisson Processes, particularly in analyzing continuous-time Markov chains and stochastic processes with memoryless properties.
- Point Processes: Understanding Point Processes as generalizations of Poisson Processes, encompassing a broader class of stochastic processes for modeling random point patterns in time or space.
- Spatial Poisson Processes: Examining Spatial Poisson Processes, which extend the concept of Poisson Processes to two or three-dimensional spaces, and their applications in spatial statistics and spatial modeling.
- Poisson Regression: Learning about Poisson Regression models, which utilize Poisson Processes to analyze count data and estimate the relationship between predictor variables and event occurrences.
- Application in Finance: Exploring the application of Poisson Processes in finance, including modeling stock price movements, interest rate processes, and credit risk assessment.
- Biological Applications: Investigating biological applications of Poisson Processes, such as modeling gene mutations, radioactive decay, and neuronal firing patterns.
- Environmental Applications: Understanding how Poisson Processes are applied in environmental studies, including modeling natural disasters, rainfall patterns, and air quality monitoring.
- Epidemiological Applications: Exploring the use of Poisson Processes in epidemiology, including modeling disease outbreaks, population growth, and healthcare resource allocation.
- Machine Learning and Data Analysis: Applying Poisson Processes in machine learning and data analysis tasks, such as anomaly detection, event prediction, and recommendation systems.
Our team of experts, with advanced degrees in statistics, is proficient in these topics and more, providing tailored solutions and guidance to help statistics students overcome challenges and excel in their Poisson Process assignments. Whether it's understanding theoretical concepts, applying statistical methods, or interpreting real-world data, we're here to support students at every step of their academic journey.
We emphasize clarity through effective data visualization, ensuring that students grasp intricate concepts in probability theory and stochastic processes. Our commitment extends to providing expertise in data science applications and utilizing statistical software for comprehensive analysis. Whether students are dealing with the nuances of the Poisson distribution, conducting hypothesis testing, or utilizing statistical tools to analyze the number of events, our assistance is tailored to enhance their understanding and proficiency in these crucial aspects of statistics.
Engaging Poisson Process Assignment Help: Streamlined Process
Navigating the process of hiring our Poisson Process Assignment Help experts is designed to be straightforward and hassle-free. We understand the importance of simplicity and efficiency in delivering academic support to students. Here's a step-by-step guide to engaging our services:
- Submission of Assignment Details: Begin by filling out our online form, providing comprehensive details about your Poisson Process assignment. Include information such as assignment guidelines, deadlines, and any specific requirements you have.
- Quotation and Agreement: Once we receive your assignment details, our team will assess the complexity and scope of the task and provide you with a personalized quotation. Review the quotation and agree to proceed with our statistics assignment help service.
- Secure Payment Process: Choose a convenient payment method from our various options, ensuring a secure and seamless transaction. We offer flexible payment options to accommodate your preferences and budget constraints.
- Confirmation of Order: Confirm your order by approving the quotation and making the payment. This secures the services of a qualified expert who specializes in Poisson Processes to work on your assignment.
- Expert Allocation: Once your payment is confirmed, we assign your assignment to a knowledgeable expert who possesses expertise in probability theory and stochastic processes, ensuring high-quality assistance tailored to your needs.
- Continuous Progress Updates: Throughout the assignment process, you'll receive regular updates on the progress of your task. This ensures transparency and allows you to provide feedback or ask questions as needed.
- Completion and Delivery: Upon completion of your assignment, we deliver the final solution within the agreed-upon timeframe. Rest assured that your assignment will adhere to academic standards and meet all specified requirements.
- Feedback and Revision Requests: After receiving the completed assignment, you'll have the opportunity to review it and provide feedback. If any revisions are necessary, simply communicate your requirements, and we'll make the necessary adjustments promptly.
- Dedicated Customer Support: Throughout the entire process, our dedicated customer support team is available 24/7 to address any inquiries or concerns you may have. We're here to ensure a supportive and responsive experience from start to finish.
Our team excels in a diverse array of topics, offering tailored solutions at affordable prices to help students thrive in their Poisson Process assignments. Whether you need assistance grasping theoretical concepts, applying mathematical models, or interpreting real-world data, we're here to support you. If you're looking for someone to 'do my Poisson Process assignment', count on us to deliver quality results. With our expertise in probability theory and stochastic processes, we empower students to succeed academically with confidence. Explore more about our services at statisticsassignmenthelp.com.
Master Poisson Process Assignments: Explore Our Informative Blogs for Enhanced Skills
Explore our blog dedicated to Poisson Process assignment help. Our professionally written blogs offer invaluable insights, tips, and strategies to tackle complex topics in probability theory. From understanding Poisson Processes to solving intricate problems, our blog provides expert guidance tailored to your academic needs. Learn effective strategies for approaching assignments, essays, and dissertations related to Poisson Processes, written by our experienced Poisson Process assignment writers.
Poisson Process Assignment Help: See What Our Clients Say
Discover what our clients have to say about our Poisson Process assignment help services. Read firsthand accounts of their experiences working with us and how our assistance has positively impacted their academic journey. From timely delivery to expert guidance, our clients share their satisfaction with our services and how it has helped them excel in Poisson Process assignments.
Unlock Success with Our Poisson Process Assignment Help Experts
Connect with our team of seasoned Poisson Process assignment experts specializing in Poisson Process assignments. Our experts possess extensive experience and expertise in probability theory and stochastic processes, enabling them to provide tailored assistance for your academic needs. From understanding fundamental concepts to solving complex problems, our experts offer personalized guidance to ensure your success. With their in-depth knowledge and dedication, they're committed to helping you excel in Poisson Process assignments.
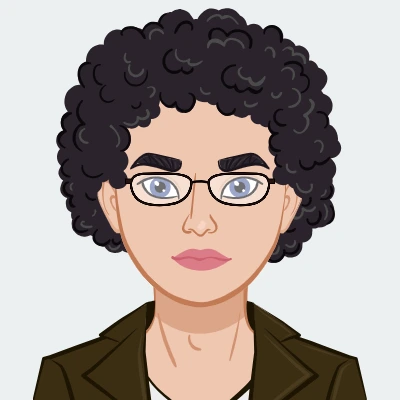
Dr. James Roberts
Ph.D. in Applied Statistics
🇬🇧 United Kingdom
Dr. James Roberts is an experienced statistics assignment expert with a Ph.D. in Applied Statistics from Greenfield University, UK. With over 14 years of expertise, Dr. Roberts specializes in advanced optimization techniques, including Simulated Annealing, and offers dedicated support to students working on complex statistical assignments, helping them achieve academic excellence.

Dr. Sarah Mitchell
Ph.D. in Statistics
🇬🇧 United Kingdom
Dr. Sarah Mitchell is a seasoned data science and statistics expert with a Ph.D. in Statistics from the University of Lincoln, UK. With over 14 years of experience, Dr. Mitchell specializes in helping students navigate complex statistical methods, particularly in multivariate analysis, and offers expert support on assignments to help students achieve exceptional academic success.
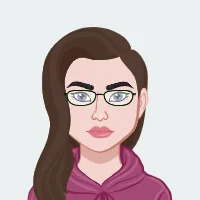
Barbara Taylor
PhD in Statistics
🇺🇸 United States
Barbara Taylor is an experienced data analyst with over 10 years of expertise in hypothesis testing. She holds a master's degree in Statistics from Georgia University and has taught data analysis and hypothesis testing at several top institutions.
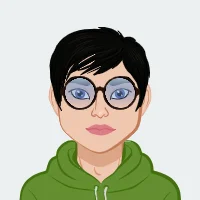
Dr. Jason Bergin
PhD in Statistics
🇺🇸 United States
Dr. Jason Bergin is a seasoned data scientist with over a decade of experience in statistical modeling and linear regression analysis. Holding a Ph.D. in Statistics, he specializes in applying advanced regression techniques to complex datasets. Jason is known for his expertise in model fitting, evaluation, and translating data insights into actionable strategies for various industries.
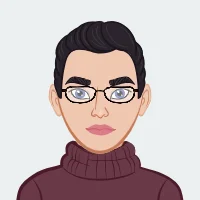
Brad Garrett
PhD in Statistics
🇬🇧 United Kingdom
Brad Garrett is an experienced statistician with a master's degree in Statistics and over 10 years of expertise in multivariate analysis, data modeling, and statistical algorithms. Specializing in the EM algorithm and Gaussian mixture models, Brad offers in-depth assignment help and guidance, ensuring students grasp complex concepts and excel in their academic pursuits in statistics.
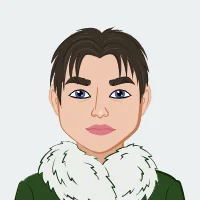
Jack Lambert
Ph.D. in Statistics
🇬🇧 United Kingdom
Jack Lambert is an experienced statistics assignment expert with a Ph.D. in statistics from the University of Stirling, UK. With over 13 years of experience, he excels in providing expert analysis and academic support in statistics.
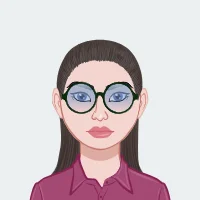
Dr. Ava Thompson
Ph.D. in Statistics
🇺🇸 United States
Dr. Ava Thompson, with a PhD in Statistics from the University of Illinois at Chicago, brings 8 years of experience in data analysis and regression techniques.
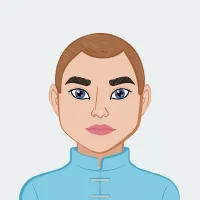
Neil Pike
Ph.D. in Statistics
🇺🇸 United States
Neil Pike is a seasoned statistics expert with a master's degree in applied Statistics. Specializing in correlation analysis and regression techniques, Neil offers precise and insightful solutions for complex data sets. His expertise lies in interpreting Pearson's correlation coefficients and regression equations, ensuring accurate analysis and clear guidance for students tackling challenging statistics assignments.
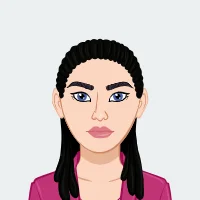
Mason Reynolds
Ph.D. in Statistics
🇺🇸 United States
Mason Reynolds is an experienced statistics assignment expert with a Ph.D. in statistics from the University of Southern Maine, USA. With over 9 years of experience, Mason specializes in helping students master complex statistical concepts and excel in their assignments.
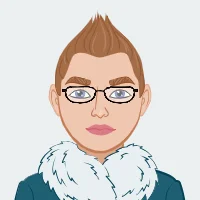
Lucas Rogers
Ph.D. in Statistics
🇺🇸 United States
Lucas Rogers is a seasoned statistics assignment expert with a Ph.D. in Statistics from the University of Vermont, USA. With over 10 years of experience, Lucas excels in applying advanced statistical techniques to complex problems, ensuring top-notch academic support.
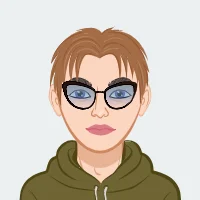
Jamie Roberts
Ph.D. in Statistics
🇺🇸 United States
Jamie Roberts is an experienced statistics assignment expert with a Ph.D. in statistics from the University of Suffolk. With over 9 years of experience, Jamie specializes in guiding students through complex statistical concepts and assignments.
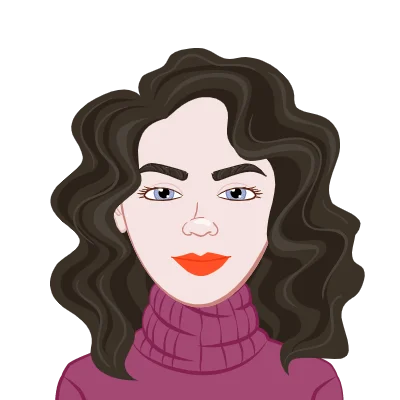
Dr. Yumi Sato
PhD in Biostatistics
🇨🇳 China
Dr. Yumi Sato earned her PhD in Biostatistics from Peking University. She has extensive experience with over 330 ANCOVA assignments and 9 years in the field. Dr. Sato is known for her exceptional ability to translate complex data into understandable results and her expertise in both theoretical and applied statistics.
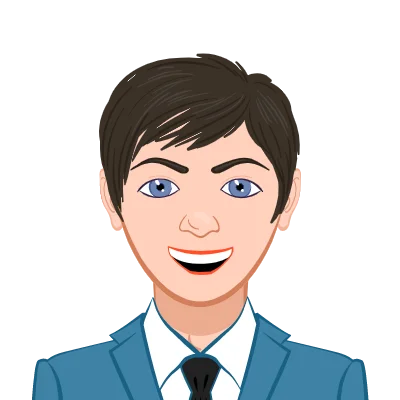
Mr. Rajesh Kumar
Master’s in Statistics
🇺🇸 United States
Mr. Rajesh Kumar holds a Master’s in Statistics from the University of British Columbia. With over 8 years of experience and more than 320 ANCOVA assignments completed, he combines academic knowledge with practical application. Rajesh is adept at addressing intricate statistical problems and providing clear, actionable solutions.
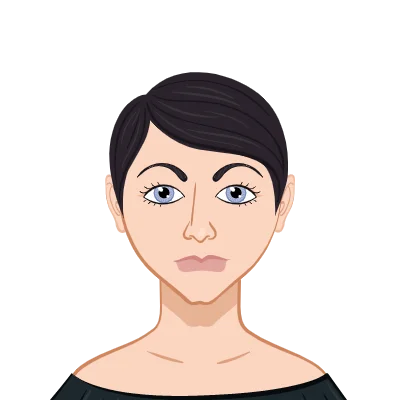
Dr. Laura Whitfield
PhD in Applied Statistics
🇬🇧 United Kingdom
Dr. Laura Whitfield received her PhD in Applied Statistics from the University of Edinburgh. She has completed over 400 ANCOVA assignments and brings more than a decade of experience in teaching and research. Dr. Whitfield is renowned for her innovative approach to statistical analysis and her dedication to enhancing students' comprehension of complex topics.

Dr. Hiroshi Nakamura
PhD in Statistics
🇯🇵 Japan
Dr. Hiroshi Nakamura earned his PhD in Statistics from the University of Tokyo. With over 12 years in academia and practical consultancy, he has completed more than 350 ANCOVA assignments. Dr. Nakamura is recognized for his thorough understanding of complex statistical models and his ability to simplify them for students and researchers alike.
Owen Bates
Ph.D. in Statistics
🇬🇧 United Kingdom
Owen Bates completed his Ph.D. in Statistics at the University of Michigan-Ann Arbor and has 9 years of experience. He specializes in Bayesian hierarchical models and causal inference, providing expert guidance in Doctorate Statistics Assignments.
Jordan Fox
Ph.D. in Statistics
🇺🇸 United States
Jordan Fox received his Ph.D. from the Georgia Institute of Technology and brings 11 years of experience. His proficiency lies in statistical quality control and network analysis, making him an invaluable expert in Doctorate Statistics Assignments.
Jake Storey
Ph.D. in Statistics
🇬🇧 United Kingdom
Jake Storey holds a Ph.D. in Statistics from the University of Oxford with 15 years of experience. Specializing in sequential analysis and robust statistics, he is a dedicated expert in Doctorate Statistics Assignments.
Tyler Hunt
Ph.D. in Statistics
🇺🇸 United States
Tyler Hunt earned his Ph.D. in Statistics from the University of Wisconsin-Madison and has 18 years of experience. His expertise includes statistical modeling and simulation techniques, making him a top expert in Doctorate Statistics Assignments.
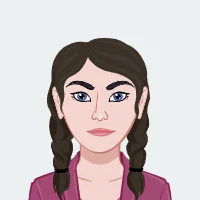
Sophie Perkins
Ph.D. in Statistics
🇺🇸 United States
Sophie Perkins is an experienced statistics assignment expert with a Ph.D. in statistics from the University of Idaho, USA. With over 8 years of experience, she excels in guiding students through complex statistical concepts and providing expert assignment support.
Related Topics
Frequently Asked Questions
Explore commonly asked questions about our Poisson process assignment help services. Find answers to queries regarding our expertise, process, pricing, and more. Learn how our experienced tutors can assist you in mastering Poisson Processes and excelling in your assignments. From understanding the basics to addressing specific concerns, our FAQ section provides comprehensive information to support your academic journey.