Claim Your Offer
Unlock an exclusive deal at www.statisticsassignmenthelp.com with our Spring Semester Offer! Get 10% off on all statistics assignments and enjoy expert assistance at an affordable price. Our skilled team is here to provide top-quality solutions, ensuring you excel in your statistics assignments without breaking the bank. Use Offer Code: SPRINGSAH10 at checkout and grab this limited-time discount. Don’t miss the chance to save while securing the best help for your statistics assignments. Order now and make this semester a success!
We Accept
- Sampling Methods: With Replacement vs. Without Replacement
- Sample Mean vs. Population Mean: Understanding the Difference
- Parameter of Interest: What It Is and Why It Matters
- Sampling Variability: Definition and Example
- Point Estimate: What It Is and Its Importance
- The Sampling Frame: A Critical Component of Sampling
- Conclusion
In the field of statistics, the concepts of sampling and estimation are foundational for conducting research and making inferences about populations. Whether you're working on a simple survey or a complex study, understanding these concepts is crucial for ensuring the accuracy and reliability of your findings. This knowledge is essential for students who aim to solve their statistics assignment effectively. This blog will delve into several key ideas, including sampling methods, the distinction between sample and population means, parameters of interest, sampling variability, point estimates, and the importance of a well-defined sampling frame. By grasping these concepts, you'll be better equipped to tackle assignments and real-world problems that require statistical analysis.
Sampling Methods: With Replacement vs. Without Replacement
Sampling is a process by which a subset of individuals or observations is selected from a larger population for the purpose of making inferences about the population. There are two primary methods of sampling: with replacement and without replacement.
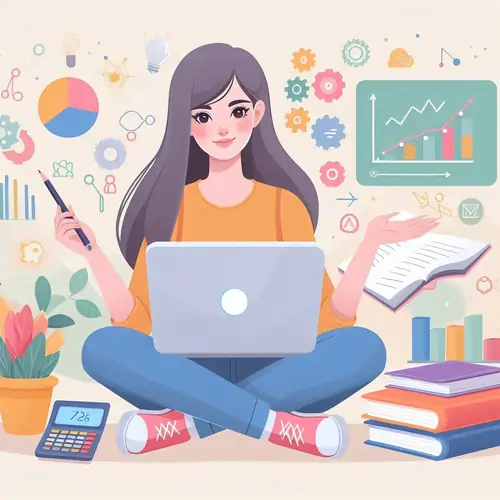
- Sampling With Replacement: In this method, after an individual or observation is selected from the population, it is returned to the population before the next selection is made. This means that the same individual or observation can be selected more than once. Sampling with replacement is often used in simulations and theoretical models because it simplifies the mathematics and allows for independent selections. However, it may not always reflect real-world scenarios where repeated selection of the same individual is unlikely.
- Sampling Without Replacement: In contrast, when sampling without replacement, once an individual or observation is selected, it is not returned to the population. This method ensures that each individual or observation can only be selected once. Sampling without replacement is more commonly used in practical research because it mirrors real-world situations where, for example, a person cannot be surveyed twice in the same study. This method tends to reduce sampling variability and often provides more precise estimates of population parameters.
Understanding the differences between these two methods is essential for choosing the appropriate sampling strategy for your research and ensuring that your results are both accurate and applicable to the population of interest.
Sample Mean vs. Population Mean: Understanding the Difference
One of the fundamental distinctions in statistics is between the sample mean and the population mean. These two measures are used to summarize data, but they serve different purposes and have different implications.
- Population Mean: The population mean (often denoted as μ) is the average of all the values in a population. It is a fixed value that represents the true central tendency of the entire population. Since the population mean includes every member of the population, it is often unknown and can be challenging to calculate, especially when dealing with large populations.
- Sample Mean: The sample mean (denoted as xˉ\bar{x}xˉ) is the average of the values in a sample, which is a subset of the population. The sample mean is used as an estimate of the population mean. Because it is based on a subset of the population, the sample mean can vary from one sample to another. This variability is known as sampling variability, and it highlights the importance of selecting a representative sample to ensure that the sample mean is a good approximation of the population mean.
The key difference between the sample mean and the population mean lies in their variability. While the population mean is a constant value, the sample mean can fluctuate depending on the sample chosen. Understanding this difference is crucial for interpreting statistical results and making inferences about the broader population.
Parameter of Interest: What It Is and Why It Matters
In statistical research, the parameter of interest is a specific numerical characteristic of a population that a researcher aims to estimate or test. This parameter could be the population mean, proportion, variance, or any other measurable attribute that defines the population's characteristics.
Identifying the parameter of interest is a critical step in any statistical study because it determines the focus of the analysis. For example, in a study aiming to understand the average height of a population, the population mean height is the parameter of interest. In another study focusing on the proportion of individuals with a particular trait, the population proportion is the parameter of interest.
The parameter of interest guides the choice of statistical methods, sampling strategies, and data analysis techniques. It also influences the formulation of hypotheses and the interpretation of results. By clearly defining the parameter of interest, researchers can ensure that their study is well-structured and that the conclusions drawn are relevant to the research question.
Sampling Variability: Definition and Example
Sampling variability refers to the natural fluctuation in sample statistics that occurs when different samples are drawn from the same population. This variability is an inherent aspect of the sampling process and is a key consideration when making inferences about a population based on sample data.
For example, imagine you are estimating the average income of residents in a city. If you take multiple random samples from the city's population, the sample means you calculate for each sample will likely differ slightly. This variation in sample means is due to sampling variability. The extent of sampling variability depends on factors such as the sample size, the sampling method, and the inherent variability within the population.
Sampling variability is important because it affects the precision of your estimates. A high level of sampling variability can lead to less precise estimates, making it more challenging to draw accurate conclusions about the population. To mitigate sampling variability, researchers often increase the sample size or use more sophisticated sampling techniques. Understanding and accounting for sampling variability is crucial for interpreting statistical results and assessing the reliability of your conclusions.
Point Estimate: What It Is and Its Importance
A point estimate is a single value derived from sample data that serves as a best guess for a population parameter. For instance, the sample mean is a point estimate of the population means, and the sample proportion is a point estimate of the population proportion.
Point estimates are widely used in statistics because they provide a straightforward and concise summary of what the data suggests about the population. However, point estimates are subject to sampling variability, meaning that different samples from the same population may yield different point estimates.
While point estimates are useful, they do not provide information about the precision of the estimate or the uncertainty associated with it. For this reason, point estimates are often accompanied by confidence intervals, which offer a range of values within which the true population parameter is likely to fall. By combining point estimates with confidence intervals, researchers can present their findings more accurately and provide a clearer picture of the uncertainty inherent in their estimates.
The Sampling Frame: A Critical Component of Sampling
A sampling frame is a list or database that includes all the elements or individuals from which a sample will be drawn. The sampling frame is a critical component of the sampling process because it defines the population from which the sample is selected and ensures that the sample is representative of the entire population.
For example, if you are conducting a survey of university students, your sampling frame might be a list of all enrolled students. The quality of the sampling frame directly affects the accuracy of your sample. If the sampling frame is incomplete or biased (e.g., it excludes certain groups of students), the sample may not accurately reflect the population, leading to biased or misleading results.
Choosing an appropriate and comprehensive sampling frame is essential for minimizing sampling bias and ensuring that the sample is truly representative of the population. Researchers must carefully consider the sampling frame during the design phase of a study to avoid errors that could compromise the validity of their findings.
Conclusion
Understanding the concepts of sampling and estimation is vital for conducting reliable and valid statistical research. Whether you're dealing with sampling methods, distinguishing between sample and population means, identifying parameters of interest, or understanding sampling variability, these concepts form the backbone of statistical analysis.
By mastering these topics, you'll be better equipped to tackle assignments and real-world problems that require statistical reasoning. Remember that the choice of sampling method, the clarity in defining your parameter of interest, and the careful selection of a sampling frame are all crucial steps in ensuring the accuracy and reliability of your statistical conclusions.
As you apply these concepts to your assignments, consider how each element—whether it's a point estimate or the variability introduced by sampling—affects your overall findings. By doing so, you'll not only improve your statistical skills but also enhance the quality of your research, leading to more meaningful and trustworthy results.