Claim Your Offer
Unlock an exclusive deal at www.statisticsassignmenthelp.com with our Spring Semester Offer! Get 10% off on all statistics assignments and enjoy expert assistance at an affordable price. Our skilled team is here to provide top-quality solutions, ensuring you excel in your statistics assignments without breaking the bank. Use Offer Code: SPRINGSAH10 at checkout and grab this limited-time discount. Don’t miss the chance to save while securing the best help for your statistics assignments. Order now and make this semester a success!
We Accept
- Understanding the Assignment
- 1. Formulating Hypotheses
- 2. Choosing the Right Measure of Central Tendency
- 3. Rationale for Statistical Analysis
- 4. Conducting the Analysis with SPSS
- 5. Reporting Additional Statistics
- 6. Writing the Results
- Conclusion
Statistical analysis can often seem daunting, especially when navigating complex methods like paired samples t-tests and repeated-measures ANOVA. These techniques are crucial in various research scenarios, particularly in fields such as psychology, medicine, and social sciences, where understanding the nuances of data is essential for drawing valid conclusions. Mastering these statistical methods not only allows researchers to test hypotheses but also enables them to make informed decisions based on their findings. This blog aims to provide students with a comprehensive overview of these statistical methods, breaking down the processes involved in conducting them step by step.
We will focus on how to effectively use SPSS, a powerful and widely used statistical software, to carry out analyses that can significantly enhance your understanding of data. By utilizing SPSS, students can streamline the analytical process, making it easier to interpret results and visualize data. Throughout this blog, you will learn about the essential features of SPSS that facilitate the execution of these analyses, as well as tips and best practices for ensuring accurate results. If you find yourself needing assistance, our SPSS assignment help can provide the guidance you require. By familiarizing yourself with these concepts and learning how to implement them using SPSS, you will be better equipped to tackle your assignments with confidence and rigor. This knowledge not only contributes to your academic performance but also builds a solid foundation for your future research endeavors, allowing you to approach complex datasets and statistical challenges with clarity and expertise.
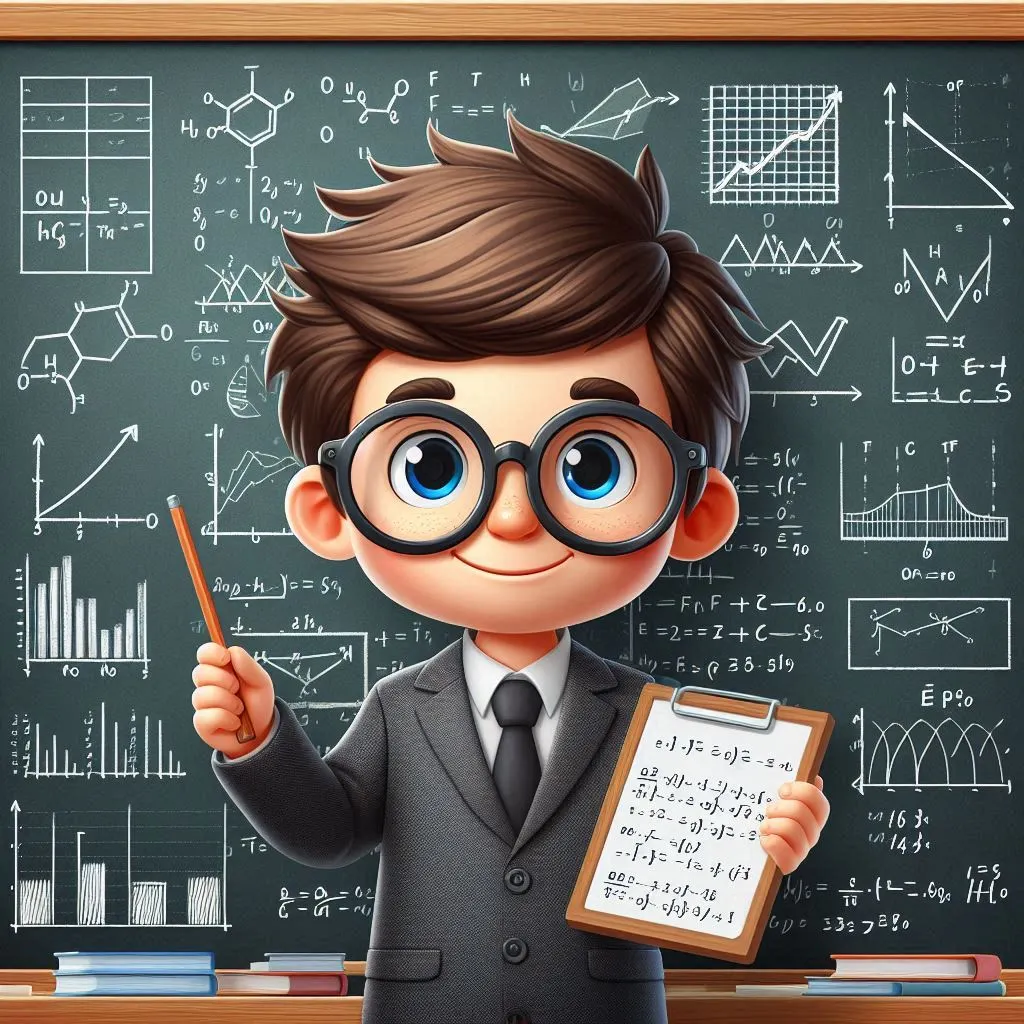
Understanding the Assignment
Before diving into the analysis, it’s essential to thoroughly read the assignment instructions to grasp what is required. This initial step is crucial as it sets the stage for the entire analytical process. Identifying the type of analysis needed—whether it’s paired samples t-tests, ANOVA, or another method—plays a pivotal role in determining your approach. If you encounter challenges along the way, seeking statistics assignment help can provide the support you need to navigate complex concepts. Here are some general steps to guide you through similar statistical assignments:
1. Formulating Hypotheses
Every statistical analysis begins with formulating clear and concise hypotheses:
- Null Hypothesis (H0): This hypothesis states that there is no effect or difference in the study. For instance, if you are analyzing the effect of an intervention, your null hypothesis might suggest that the intervention has no impact on the outcome variable being studied. This serves as the baseline for comparison, allowing you to evaluate any changes in data against a standard assumption of no effect.
- Research Hypothesis (H1): In contrast, this hypothesis represents your prediction about the expected outcome. It indicates that there is a significant effect or difference due to the intervention, setting the direction for your analysis. Formulating these hypotheses is essential, as they guide your statistical testing and help you articulate your research objectives clearly.
Clearly stating these hypotheses not only sets the foundation for your analysis but also provides a framework for interpreting your results. This foundational step is crucial in statistical research, as it defines the scope of your investigation and helps to ensure that your analysis remains focused and relevant.
2. Choosing the Right Measure of Central Tendency
For each variable involved in your analysis, it is vital to identify the appropriate measure of central tendency—mean, median, or mode. Understanding these concepts will enhance the clarity and accuracy of your results:
- Mean: This is the average of your data points and is sensitive to extreme values, making it useful in many scenarios but potentially misleading if the data contains outliers. The mean provides a quick snapshot of your data but should be interpreted with caution, especially in skewed distributions.
- Median: The median is the middle value when the data is ordered and is particularly useful when dealing with skewed distributions. It provides a more accurate reflection of the central tendency when extreme values are present. This is especially important in fields like psychology and social sciences, where data distributions can often be skewed. The median is often favored in cases where you want to understand the typical value without the influence of outliers.
- Mode: The mode represents the most frequently occurring value in your data set and is applicable for categorical data. It can provide insights into the most common responses or occurrences within your data, highlighting trends that might not be apparent through the mean or median.
Choosing the right measure will ensure that your findings are reported accurately and meaningfully. It’s crucial to consider the nature of your data and select the measure that best represents its characteristics, as this decision can significantly influence the interpretation of your results.
3. Rationale for Statistical Analysis
In your assignment, it’s important to provide a well-reasoned justification for your chosen statistical analysis. Discussing why this method is appropriate for your data and research questions is essential. This rationale might involve considerations such as:
- The type of data you are working with (continuous vs. categorical). Understanding whether your data falls into these categories helps determine the appropriate tests to employ. For example, if you have categorical data, non-parametric tests might be more suitable than parametric tests.
- The design of your study (independent vs. paired samples). Recognizing whether your samples are dependent (e.g., pre-test/post-test) or independent affects the choice of statistical tests. Paired samples t-tests, for instance, are used for comparing two related groups, while independent samples t-tests are employed for comparing two separate groups.
- The assumptions of the tests being employed (e.g., normality, homogeneity of variances). Familiarizing yourself with these assumptions allows for the appropriate selection of tests and enhances the validity of your results. Conducting tests for normality (e.g., Shapiro-Wilk test) or homogeneity of variances (e.g., Levene’s test) can provide additional insights into the appropriateness of your analysis.
Providing a strong rationale not only enhances the credibility of your analysis but also demonstrates your understanding of statistical methodologies, reinforcing the integrity of your research. This depth of analysis reflects a high level of academic rigor, making your work more persuasive and reliable.
4. Conducting the Analysis with SPSS
Utilizing statistical software like SPSS is fundamental to conducting your analysis efficiently. Here are some general steps to follow:
- Input Data: Enter your data correctly in SPSS. Pay careful attention to variable names, types, and the coding of categorical variables. Properly formatted data is crucial for accurate results. It’s often helpful to use labels for your variables to enhance clarity.
- Select Analysis: Navigate to the appropriate statistical test (e.g., Paired Samples t-test, One-Way ANOVA) under the “Analyze” menu. Make sure to choose the correct test based on your hypotheses and data characteristics. This decision greatly impacts your analysis and results. Familiarize yourself with the various options available within SPSS, as understanding the software's capabilities can facilitate smoother analysis.
- Interpret Output: After running the analysis, SPSS will generate an output file with essential statistics, including p-values, means, confidence intervals, and effect sizes. It is crucial to ensure you understand each component of the output to draw accurate conclusions from your results. Familiarize yourself with SPSS output terminology and formats, which can help you navigate and interpret the results more efficiently.
- Validation of Results: After interpreting the output, it’s wise to validate your findings. Consider using visual aids like histograms or box plots to examine the distribuValidation of Results: After interpreting the output, it’s wise to validate your findings. Consider using visual aids like histograms or box plots to examine the distribution of your data. These visualizations can help identify any anomalies or trends that might not be apparent from the numerical output alone.
5. Reporting Additional Statistics
Beyond the primary analysis, it is beneficial to report additional statistics that provide context to your findings. Consider calculating and reporting effect sizes (such as Cohen's d or eta-squared) to illustrate the magnitude of the effects observed. Effect sizes provide valuable insight into the practical significance of your results beyond mere statistical significance. This information can help clarify the practical implications of your findings and is often a critical element in discussing the impact of your research.
Additionally, you might want to report confidence intervals for your estimates, which can help to illustrate the range within which the true population parameter lies. Reporting these intervals gives readers a clearer picture of the reliability of your estimates and enhances the comprehensiveness of your findings. Effectively communicating the reliability and significance of your results is key to contributing meaningfully to the existing body of knowledge.
6. Writing the Results
When documenting your findings, adherence to APA style is essential for clarity and professionalism. Structure your report effectively:
- Procedure Section: In this section, describe the steps taken in your analysis. Include details on your hypotheses, data collection methods, and the statistical tests employed. This transparency is vital for replicability and understanding. A detailed procedure not only aids in clarity but also allows others to follow your analytical path. Including any challenges you faced during the analysis can also provide valuable insights into the analytical process.
- Results Section: Present your findings clearly and concisely. Include statistical outputs, graphs, and any relevant statistics. Make sure your writing is precise and free from jargon, ensuring that it remains accessible for your intended audience. Use clear headings, bullet points, and tables where appropriate to enhance readability. Ensure that your visual aids (graphs and tables) are well-labeled and referenced in the text to facilitate understanding.
- Discussion Section: Consider adding a discussion section where you interpret the findings in the context of your research question. Discuss the implications of your results, how they align with or contradict existing literature, and any potential limitations of your study. Addressing limitations shows a critical engagement with your work and can lead to further research opportunities.
By following these structured steps, you will not only enhance your understanding of statistical analyses but also improve your ability to communicate your findings effectively. This approach will make your assignments more robust and credible, ultimately leading to better academic performance. Embracing these analytical skills will serve you well throughout your academic career and beyond, as statistical reasoning is a valuable asset in various fields of study and professional domains.
Conclusion
In conclusion, mastering paired samples t-tests and repeated-measures ANOVA through the effective use of SPSS equips you with powerful statistical tools essential for analyzing and interpreting data. These methods allow researchers to examine differences within related groups, making them invaluable for studies that require a nuanced understanding of the effects of interventions or treatments over time. By comprehensively grasping these techniques, you enhance your analytical skill set, enabling you to extract meaningful insights from complex datasets.
Furthermore, the ability to conduct and interpret these analyses not only prepares you for academic success but also significantly enhances your capabilities in the workforce. In today's data-driven environment, employers increasingly seek individuals who can adeptly analyze data and translate findings into actionable strategies. Thus, your proficiency in statistical methods such as paired samples t-tests and repeated-measures ANOVA becomes a crucial asset in various fields, from healthcare and psychology to marketing and social sciences.
As you continue to develop your statistical skills, remember that the journey does not end with merely understanding how to conduct these analyses. The real mastery lies in your ability to contextualize the results, communicate them effectively, and apply the insights gained to real-world scenarios. Each analysis you perform serves as a stepping stone toward greater expertise in data interpretation and decision-making.
Moreover, embracing a mindset of continuous learning in statistics will serve you well beyond your academic pursuits. The landscape of statistical analysis is ever-evolving, with new methods and software updates emerging regularly. Staying abreast of these developments will not only enhance your current knowledge but also prepare you for future challenges in your academic and professional journey. Consider exploring additional resources such as online courses, workshops, or webinars to deepen your understanding of advanced statistical techniques and their applications.
In addition, collaborating with peers and engaging in discussions about statistical methods can further enrich your learning experience. Sharing insights, troubleshooting problems, and celebrating successes with fellow students fosters a supportive learning environment that can help demystify complex concepts. Remember that asking questions and seeking clarification are signs of strength, not weakness. Engaging actively with your learning community can lead to deeper understanding and inspire new ideas.
It’s also important to recognize the ethical implications of statistical analysis. As you analyze data, consider the impact your findings may have on individuals and communities. Responsible reporting and interpretation of results are vital to ensuring that research contributes positively to society. Strive to maintain integrity and transparency in your analyses, and always be mindful of the potential consequences of your work.
In summary, the skills you cultivate in utilizing SPSS for paired samples t-tests and repeated-measures ANOVA are not just tools for completing assignments; they are foundational competencies that will aid you in your quest for knowledge and professional development. By effectively leveraging these analytical techniques, you position yourself as a competent and confident researcher, ready to tackle complex questions and contribute meaningfully to your field. Embrace these tools with enthusiasm, and let them guide you as you navigate the fascinating world of data analysis, where each finding has the potential to impact lives and inform future research.
As you embark on this journey, maintain a sense of curiosity and an eagerness to learn. The world of statistics is vast and rich with opportunities for exploration. Every dataset tells a story, and you hold the key to uncovering its secrets. With determination, resilience, and a commitment to continuous improvement, you will not only enhance your analytical abilities but also empower yourself to make informed decisions that can lead to meaningful change.