Claim Your Offer
Unlock a fantastic deal at www.statisticsassignmenthelp.com with our latest offer. Get an incredible 10% off on all statistics assignment, ensuring quality help at a cheap price. Our expert team is ready to assist you, making your academic journey smoother and more affordable. Don't miss out on this opportunity to enhance your skills and save on your studies. Take advantage of our offer now and secure top-notch help for your statistics assignments.
We Accept
- Understanding Key Concepts
- 1. Sufficiency and Sufficiency Theorems
- 2. Completeness and Completeness Theorems
- 3. Exponential Families of Distributions
- Point Estimation
- Criteria for Evaluating Estimators
- Advanced Topics in Point Estimation
- Bayesian Techniques
- Tests of Hypotheses
- Strategies for Scoring High in Parametric Inference Assignments
- Conclusion
Parametric inference, a cornerstone of statistics, involves making inferences about population parameters based on sample data. Point estimation, a crucial aspect of this field, entails estimating a single value for an unknown parameter from the available data. In this blog, we will delve into various strategies and concepts to help you understand and excel in point estimation and related topics in parametric inference. If you're seeking assistance with your Inference assignment, this blog will provide comprehensive insights and strategies to enhance your understanding and proficiency in these statistical concepts.
Mastering point estimation requires a deep understanding of sufficiency, completeness, exponential families of distributions, and advanced criteria like mean square error, unbiasedness, and consistency. Additionally, Bayesian techniques, hypothesis testing methods, and strategic study approaches play a vital role in scoring well in assignments. If you're looking for help with your statistics assignment, mastering these concepts and techniques will be essential for achieving proficiency and success in your studies.
By focusing on these fundamental concepts, practicing their applications through examples, and employing effective study techniques, you can enhance your comprehension and proficiency in point estimation, enabling you to excel in your parametric inference assignments and examinations.
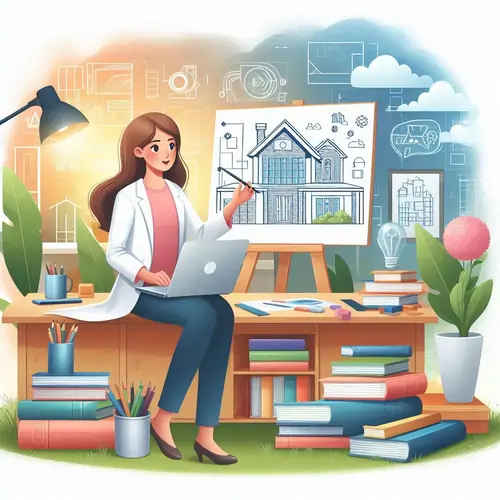
Understanding Key Concepts
1. Sufficiency and Sufficiency Theorems
Sufficiency is a fundamental concept in statistics that determines if a statistic contains all the information in the sample relevant to the parameter being estimated. A statistic is sufficient if no other statistic that can be calculated from the same sample provides any additional information about the parameter. This is crucial because it allows us to reduce the amount of data needed for estimation without losing information.
The Factorization Theorem, a key result in sufficiency theory, provides a formal criterion for identifying sufficient statistics. It states that a statistic is sufficient if the joint probability distribution of the sample data can be factored into two functions: one that depends on the sample data only through the sufficient statistic and another that depends on the parameter only.
Minimal sufficiency is an extension of sufficiency that indicates a statistic is minimal if it cannot be reduced further to another sufficient statistic. Understanding these concepts will help you identify the most efficient statistics for your estimations.
2. Completeness and Completeness Theorems
Completeness is another critical property that a family of probability distributions can possess. A family of distributions is complete if the only function of the parameter that has expected value zero for all distributions in the family is the function that is zero everywhere. Completeness ensures that the best unbiased estimator for the parameter exists and is unique.
Lehmann-Scheffe theorem is a fundamental result that relates sufficiency and completeness. It states that under certain conditions, the best unbiased estimator of a parameter is a function of a complete, sufficient statistic. This theorem highlights the importance of using complete, sufficient statistics in parametric inference.
Basu's theorem connects sufficiency and independence of a statistic and an ancillary statistic, another vital concept in the theory of estimation. An ancillary statistic is one whose distribution does not depend on the parameter being estimated, which can simplify the process of estimation.
3. Exponential Families of Distributions
Exponential families of distributions are a class of probability distributions that have a specific form, making them mathematically tractable. They include many commonly used distributions such as the normal, exponential, and gamma distributions.
These distributions are often characterized by canonical parameters and canonical sufficient statistics, which simplify the process of estimating their parameters. Canonical parameters are natural parameters that characterize the distribution in the exponential family, while canonical sufficient statistics are sufficient statistics that are natural to the exponential family and often used in deriving estimators.
Point Estimation
Point estimation is a fundamental concept in statistics that involves the process of using sample data to estimate an unknown parameter of interest with a single value, referred to as a point estimate. This estimate is crucial in making decisions and drawing conclusions about populations based on sample data. The goal of point estimation is to find the most likely value of the parameter given the observed data. Point estimates can be evaluated using several criteria, such as mean square error, unbiasedness, relative efficiency, consistency, and more. Understanding these criteria is essential for determining the reliability and accuracy of point estimates. Moreover, point estimation plays a vital role in various fields, including economics, biology, engineering, and social sciences, where understanding population parameters is critical for decision-making and policy development.
Criteria for Evaluating Estimators
There are several criteria for evaluating the quality of point estimators:
- Mean Square Error (MSE):MSE is the expected value of the squared difference between the estimator and the parameter.
- Unbiasedness: An estimator is unbiased if its expected value equals the true parameter value.
- Relative Efficiency: Relative efficiency compares the performance of two estimators in terms of their variances.
- Cramer-Rao Inequality: The Cramer-Rao inequality provides a lower bound on the variance of any unbiased estimator.
- Consistency:An estimator is consistent if it converges to the true parameter value as the sample size increases.
Advanced Topics in Point Estimation
Advanced topics in point estimation include:
- UMVUE (Uniformly Minimum Variance Unbiased Estimator): The UMVUE is the estimator that has the smallest variance among all unbiased estimators.
- Rao-Blackwell Theorem: This theorem states that a function of a sufficient statistic that is an unbiased estimator is also the best unbiased estimator (minimum variance estimator) in the class of unbiased estimators.
- Bayesian Estimation:Bayesian estimation involves using prior beliefs about the parameter to update and obtain a posterior distribution, from which estimates of the parameter can be made.
Bayesian Techniques
Bayesian estimation offers a distinctive approach to parametric inference, integrating prior beliefs about the parameter with observed data to refine those beliefs.
- Priors: These encapsulate existing knowledge or assumptions about the parameter before data collection, serving as the foundation for Bayesian analysis.
- Posteriors: As data is observed, priors are updated to form posteriors, representing the revised beliefs about the parameter based on both prior knowledge and observed data.
- Bayes' Estimators: These estimators are formulated using Bayesian principles, often by minimizing the expected loss based on the posterior distribution. They provide a coherent framework for decision-making in uncertain environments.
- Bayesian Credible Regions: These regions in parameter space signify intervals with a specified probability of encompassing the true parameter value, offering a measure of uncertainty in Bayesian inference.
Tests of Hypotheses
Statistical hypothesis testing is a fundamental part of parametric inference, involving several key components:
- Hypotheses:In hypothesis testing, we state a null hypothesis (typically denoted as 𝐻0) and an alternative hypothesis (𝐻1or 𝐻𝑎). The null hypothesis represents the status quo or no effect, while the alternative hypothesis represents what we are testing for.
- Critical Regions: These are defined regions of the sample space that, if the test statistic falls within them, lead to the rejection of the null hypothesis. The size and shape of these regions are determined by the significance level of the test.
- Neyman-Pearson Lemma:This lemma provides a method for constructing the most powerful tests, which maximize the probability of correctly rejecting the null hypothesis when it is false, subject to a constraint on the probability of rejecting it when it is true.
- UMP (Uniformly Most Powerful), UMPU (Uniformly Most Powerful Unbiased), and LMP (Locally Most Powerful) tests: These are types of hypothesis tests that are optimal in various senses. UMP tests are the most powerful tests for a given significance level, UMPU tests are the most powerful unbiased tests, and LMP tests are the most powerful tests in a local region of the parameter space.
- Monotone Likelihood Ratio Family: This refers to a class of distributions that satisfy the monotone likelihood ratio property. This property ensures that the likelihood ratio test is monotonic in the sense that the test statistic either always increases or always decreases as the sample size increases, simplifying hypothesis testing.
Understanding these components is essential for designing effective hypothesis tests and interpreting their results accurately in parametric inference.
Strategies for Scoring High in Parametric Inference Assignments
Mastering point estimation and related topics in parametric inference requires a combination of theoretical understanding and practical application. Here are some strategies to help you excel in your assignments:
- Understand Theoretical Concepts: Focus on understanding the definitions, theorems, and their implications. Practice deriving sufficient statistics, applying the factorization theorem, and checking the conditions for completeness.
- Practice Calculations:Work through examples to calculate UMVUEs, apply the Cramer-Rao inequality, and understand how to derive and apply Bayesian estimators.
- Review Assumptions:Understand the assumptions under which the theorems and inequalities hold. This will help in applying them correctly and avoiding common mistakes.
- Work with Real-World Data: Apply these concepts to real data sets to gain practical experience and see how the theory applies in practice.
- Review Numerical Methods: Understand computational methods for estimation and hypothesis testing, including numerical optimizations and simulation-based techniques.
- Utilize Resources:Use textbooks, lecture notes, online resources, and practice problems to reinforce your understanding.
Conclusion
Mastering point estimation and related topics in parametric inference is an intricate journey that demands a comprehensive grasp of theoretical underpinnings, adept computational abilities, and the acumen to apply concepts in real-world scenarios. By immersing yourself in understanding key concepts such as sufficiency, completeness, and Bayesian techniques, and honing your skills through diligent practice, you can navigate the complexities of parametric inference with confidence. Remember, consistent practice coupled with a resilient attitude towards challenges is paramount for achieving excellence in this domain. Embrace the learning process, seek out resources for reinforcement, and never shy away from seeking clarification when needed. With dedication and perseverance, you can unlock the door to success in this challenging yet immensely rewarding field.