Claim Your Offer
Unlock a fantastic deal at www.statisticsassignmenthelp.com with our latest offer. Get an incredible 10% off on all statistics assignment, ensuring quality help at a cheap price. Our expert team is ready to assist you, making your academic journey smoother and more affordable. Don't miss out on this opportunity to enhance your skills and save on your studies. Take advantage of our offer now and secure top-notch help for your statistics assignments.
We Accept
- Wald’s Sequential Probability Ratio Test (SPRT)
- Understanding Wald’s SPRT:
- Illustration with Binomial Distribution:
- Average Sample Number (ASN) and Operating Characteristic (OC) Function
- ASN and OC Function:
- Application and Analysis:
- Stein’s Two-Stage Fixed-Length Confidence Interval
- Understanding Stein’s Method:
- Practical Example with Normal Distribution:
- Sequential Estimation
- Fundamental Concepts:
- Practical Application:
- Tips for Success in Assignments on Sequential Methods:
- Conclusion
Sequential methods are pivotal in modern statistical analysis, offering dynamic approaches to decision-making that adapt as new data becomes available. For students delving into this field, mastering sequential methods like Wald’s Sequential Probability Ratio Test (SPRT), Average Sample Number (ASN), Operating Characteristic (OC) function, Stein’s two-stage fixed-length confidence interval, and sequential estimation is crucial. This blog explores these advanced topics, providing detailed explanations and practical examples using Binomial and Normal distributions. It also offers strategies for effective studying to excel in assignments. Understanding these methods not only enhances theoretical knowledge but also equips students with practical skills applicable across various industries. By grasping the nuances of sequential analysis, students can confidently tackle complex decision-making scenarios, optimize resource allocation, and contribute to evidence-based practices in fields such as healthcare, finance, and quality control. If you're seeking assistance with your statistics assignment, this guide will provide the necessary insights and strategies to help you master these essential sequential methods.
Wald’s Sequential Probability Ratio Test (SPRT)
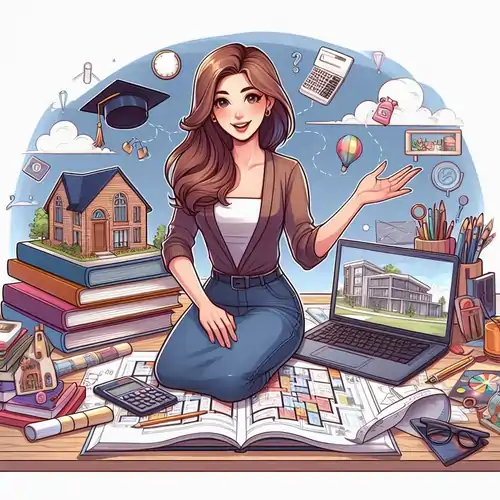
Wald’s Sequential Probability Ratio Test (SPRT) stands as a pivotal advancement in the realm of hypothesis testing. It introduces a dynamic approach where decisions evolve sequentially based on accumulating data, thus optimizing sample sizes and error rates simultaneously. This methodological innovation is particularly significant in scenarios requiring timely and cost-effective decision-making without compromising statistical rigor. By continuously updating the likelihood ratio and comparing it against predefined thresholds, SPRT ensures that decisions are made efficiently and accurately. This adaptive process not only reduces the overall sample size needed but also controls Type I and Type II error rates effectively. Understanding SPRT involves grasping the intricacies of sampling plans, decision rules, and the theoretical underpinnings of sequential analysis. Mastery of SPRT empowers statisticians and researchers to navigate complex decision landscapes with confidence, making it an indispensable tool in modern statistical practice and academic study alike.
Understanding Wald’s SPRT:
Wald’s Sequential Probability Ratio Test (SPRT) is a sophisticated method that transforms how we approach hypothesis testing by allowing decisions to evolve as data accumulates. This sequential approach hinges on continuously updating the likelihood ratio and comparing it against predefined thresholds to ensure efficient decision-making.
- Sampling Plans: Designing effective sampling plans is crucial in Wald’s SPRT methodology. It entails making strategic choices regarding sample sizes and decision thresholds that strike a delicate balance between Type I and Type II errors. The challenge lies in optimizing these parameters to achieve robust statistical conclusions while minimizing the risk of both false positives and false negatives.
- Decision Rules: Integral to Wald’s SPRT are the decision rules that govern the termination of sequential sampling. These rules establish clear criteria based on the likelihood ratio surpassing predefined bounds, thus signaling when to halt sampling and reach a decision. By setting precise thresholds, analysts ensure that conclusions are drawn with minimal data, promoting efficiency without compromising statistical rigor.
Illustration with Binomial Distribution:
Consider a scenario where you need to determine if a new manufacturing process meets quality standards:
- Scenario:Testing if a machine produces defective items at an acceptable rate (p ≤ 0.05).
- Implementation:Sequentially sample items, updating the likelihood ratio after each sample until a decision threshold (e.g., stop if the ratio exceeds bounds for acceptance or rejection) is reached.
Average Sample Number (ASN) and Operating Characteristic (OC) Function
Average Sample Number (ASN) and Operating Characteristic (OC) Function are indispensable tools for assessing the effectiveness of sequential tests in decision-making scenarios. ASN quantifies the expected number of samples required to reach a decision under a specific sampling plan and decision rule, offering insights into the efficiency of sequential testing strategies. It provides a metric to optimize resource allocation and decision-making timelines, crucial in fields like quality control and clinical trials where minimizing sample sizes without compromising accuracy is paramount.
On the other hand, OC Function graphically illustrates the probability of making correct decisions across different stages of sequential sampling. By plotting decision probabilities against sample sizes or decision thresholds, OC curves enable analysts to evaluate and compare the performance of various sequential testing procedures. Understanding ASN and OC Function not only enhances theoretical knowledge but also equips students with practical skills to design and implement efficient sequential testing strategies in real-world applications.
ASN and OC Function:
- Average Sample Number (ASN): Represents the expected sample size needed to reach a decision under a given sampling plan and decision rule.
- Operating Characteristic (OC) Function: Graphically depicts the probability of making correct decisions at different stages of sampling, crucial for evaluating the performance of sequential tests.
Application and Analysis:
- Comparative Analysis: Compare different sequential sampling plans (e.g., fixed sample size vs. sequential) using ASN and OC curves. Understand how these metrics vary with decision thresholds and error rates.
- Real-World Relevance: Explore applications in clinical trials or quality control where minimizing sample sizes while ensuring reliable decisions is critical.
Stein’s Two-Stage Fixed-Length Confidence Interval
Stein’s method introduces an innovative strategy for constructing fixed-length confidence intervals in a sequential manner, effectively balancing the trade-offs between precision and sample size. Unlike traditional methods that require predetermined sample sizes, Stein’s approach adapts dynamically to accumulating data, ensuring confidence intervals remain fixed in length regardless of sample size.
Understanding Stein’s Method:
- Fixed-Length Intervals: Provide confidence intervals that do not depend on the sample size, crucial for applications requiring quick decisions or resource constraints.
- Procedure:Involve an initial stage to estimate parameters followed by a second stage to refine and finalize the interval based on accumulated data.
Practical Example with Normal Distribution:
- Scenario:Estimating the mean of a population with a limited number of observations.
- Implementation:Sequentially calculate intervals using initial data points, adjusting boundaries based on subsequent observations to maintain fixed-length criteria.
Sequential Estimation
Sequential estimation plays a pivotal role in adaptive statistical inference, allowing for continuous updates of estimates as fresh data streams in, which is vital for dynamic decision-making scenarios. This methodological approach is particularly advantageous in environments where decisions must be made promptly and with evolving information. By employing Bayesian updating techniques, sequential estimation iteratively refines initial beliefs or parameters based on the most recent observations. This iterative refinement not only improves the accuracy of estimates but also provides a flexible framework to adjust strategies in response to changing circumstances. For instance, in fields like finance, healthcare, or engineering, where real-time adjustments are critical, sequential estimation ensures that decisions are informed by the latest available data, thereby enhancing the precision and reliability of outcomes. Mastering sequential estimation equips students with a powerful toolset to navigate complex decision-making processes that require responsiveness to ongoing data flows and uncertainties.
Fundamental Concepts:
- Bayesian Updating: Continuously update prior beliefs with new evidence, refining estimates iteratively.
- Likelihood Ratio Tests:Utilize likelihood ratios to compare competing hypotheses, adjusting estimates based on accumulating data.
Practical Application:
- Example:Monitoring the effectiveness of a marketing campaign based on incremental sales data.
- Implementation: Sequentially update expected outcomes using Bayesian methods, adjusting strategies in real-time based on evolving market responses.
Tips for Success in Assignments on Sequential Methods:
Mastering sequential methods requires a multifaceted approach that combines theoretical understanding with practical application and real-world relevance. Here’s how you can enhance your proficiency:
- Practice Problems: Engage extensively in solving a diverse range of problems involving SPRT, ASN, OC function, Stein’s intervals, and sequential estimation. Apply these methods rigorously to theoretical scenarios and practical case studies to reinforce your understanding and problem-solving skills.
- Conceptual Mastery:Develop a robust grasp of the theoretical foundations and underlying assumptions of sequential methods. Explore scenarios where sequential approaches demonstrate superiority over traditional methods, gaining insight into when and why these methods are advantageous.
- Numerical Proficiency:Strengthen your computational skills to execute calculations involved in sequential testing and estimation accurately. Utilize statistical software for simulations and complex computations, gaining hands-on experience in implementing sequential methodologies.
- Application Awareness: Stay informed about real-world applications across diverse industries such as healthcare, finance, and manufacturing. Understand how sequential methods facilitate efficient decision-making processes, preparing yourself to apply these techniques effectively in practical settings.
By implementing these strategies and mastering the intricacies of sequential methods, students can not only excel in academic assignments but also prepare themselves for practical challenges in data-driven decision-making scenarios.
Conclusion
In conclusion, mastering sequential methods is essential for students pursuing studies in statistics and data-driven decision-making. The advancements in Wald’s Sequential Probability Ratio Test (SPRT), Average Sample Number (ASN), Operating Characteristic (OC) function, Stein’s two-stage fixed-length confidence interval, and sequential estimation represent significant strides in statistical theory and practice. These methods empower efficient and adaptive decision-making processes essential across diverse fields such as healthcare, finance, and manufacturing. By understanding these methodologies deeply, students can navigate complex data scenarios with confidence, making informed decisions based on incremental data updates. As the demand for precision and efficiency in decision-making grows, proficiency in sequential methods equips students to contribute effectively in both academic settings and practical applications. Embracing these advancements fosters a deeper understanding of statistical inference and enhances the ability to derive actionable insights from data, positioning students for success in their academic and professional endeavors alike.