Understanding Problems Based on Measures of Dispersion in Statistics Assignments

Statistics assignments often present students with a diverse range of problems related to measures of dispersion, requiring a deep understanding of the intricacies of data spread. One of the primary challenges lies in comprehending the concept of range, where students must decipher the significance of the difference between the highest and lowest values in a dataset. Interpreting the range becomes especially complex when outliers are present, demanding students to critically assess the overall data distribution. Additionally, problems involving quartiles and percentiles necessitate students to not only calculate these values accurately but also grasp their implications in dividing the dataset into equal parts. The ability to discern the distributional characteristics of data through quartiles and percentiles is crucial for making informed statistical decisions and drawing meaningful conclusions from the dataset. So, when you need to complete your dispersion assignment using statistics, these concepts will be essential in your coursework.
Furthermore, students often grapple with problems related to variance and standard deviation, central measures of dispersion. Calculating variance requires meticulous attention to detail, as it involves squaring differences from the mean and averaging them. The intricacy deepens when interpreting the standard deviation, which measures the spread of data points from the mean. It's not merely a numerical value; rather, it signifies the extent of variability within the dataset. Developing a profound understanding of these concepts not only aids students in acing their assignments but also equips them with the analytical skills necessary to tackle real-world problems, making them proficient statisticians capable of navigating the complexities of diverse datasets.
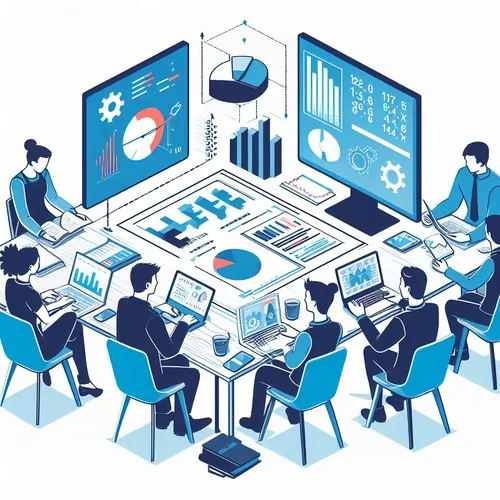
Problems Involving Range, Quartiles, and Percentiles
Solving problems related to range, quartiles, and percentiles presents students with a multifaceted understanding of data distribution. Calculating the range not only teaches the fundamental skill of finding the spread between the maximum and minimum values but also hones the ability to recognize outliers that can significantly impact the interpretation. Delving into quartiles challenges students to divide data into four segments, enhancing their comprehension of the dataset's structure and allowing them to grasp the central tendencies more effectively. Meanwhile, grappling with percentiles equips students with the skill to identify specific data points' relative positions, providing valuable insights into the dataset's overall composition. Mastering these concepts not only sharpens statistical prowess but also empowers students to draw meaningful conclusions about data variability, essential in both academic and practical contexts.
Range: Interpreting Data Spread
Interpreting the range of a dataset is akin to deciphering the boundaries within which data fluctuates. The range, the simplest measure of dispersion, represents the numeric distance from the lowest to the highest value in a dataset. For instance, consider a dataset representing the ages of students in a classroom. If the youngest student is 10 years old, and the oldest is 18, the range would be 8 years. However, while the range provides a quick glimpse into the dataset's diversity, it often fails to account for outliers—those exceptional values that lie far apart from the rest. Therefore, when interpreting the range, it's vital to consider the context of the data. Understanding the range demands not just computational accuracy but also a critical eye for the nuances of the dataset, ensuring a nuanced perspective on the true spread of the information at hand.
Quartiles: Understanding Data Distribution
Quartiles play a pivotal role in understanding the distribution of data within a dataset. When students delve into problems related to quartiles, they are confronted with the task of dividing a dataset into four equal parts, each representing a quarter of the data. The first quartile (Q1) marks the 25th percentile, indicating that 25% of the data falls below this value. Similarly, the third quartile (Q3) represents the 75th percentile, signifying that 75% of the data lies below this point. The second quartile (Q2) is the median, denoting the midpoint of the data. Analyzing quartiles allows students to comprehend not only the central tendency but also the spread of the data. By grasping the nuances of quartiles, students gain insights into how data is dispersed across different segments, enabling them to make informed interpretations about the dataset's distribution and identify potential outliers or skewed patterns within the data.
Percentiles: Analyzing Data Position
In the realm of statistics, understanding percentiles is akin to deciphering a map that guides us through the landscape of data distribution. Percentiles represent specific data points below which a given percentage of observations reside. For instance, the 25th percentile signifies that a quarter of the data falls below that value. Mastering problems related to percentiles demands a keen eye for detail and a knack for interpolation, especially when dealing with datasets of varying sizes. Analyzing percentiles allows statisticians to discern the relative position of data, crucial for tasks ranging from educational assessments to economic analyses. By honing their skills in calculating and interpreting percentiles, students gain invaluable insights into the nuances of data positioning, enabling them to navigate the intricate pathways of statistical analysis with confidence and precision.
Variance and Standard Deviation Challenges
Calculating variance and standard deviation presents a formidable challenge for students delving into the depths of statistics. The intricacies lie not only in comprehending the underlying formulas but also in grasping the profound implications these measures have on data interpretation. Variance, demanding meticulous squaring of differences from the mean followed by averaging, can be a daunting task, especially with extensive datasets. Students grapple with the complexities of ensuring accuracy through each step, understanding that even a minor miscalculation can significantly alter the final result. Equally perplexing is the realm of standard deviation, the square root of variance. Its calculation, though rooted in the variance formula, adds another layer of complexity. Interpreting the standard deviation values challenges students to discern the nuances of data spread accurately. Misjudging this measure can lead to erroneous conclusions, underscoring the importance of a thorough understanding of these challenges in the world of statistics.
Variance: Calculating Average Dispersion
Calculating variance is a fundamental yet intricate task in statistics, demanding a meticulous approach to data analysis. In this context, variance quantifies the average dispersion of individual data points from the mean of the dataset. The process involves squaring the differences between each data point and the mean, summing up these squared differences, and then dividing the result by the total number of data points. This squared difference not only highlights the spread of data but also accounts for the significance of each deviation, whether positive or negative, emphasizing the deviations' magnitude. In essence, variance provides a comprehensive view of the dataset's variability, making it a crucial measure for understanding the overall distribution of data points and enabling statisticians and researchers to draw meaningful conclusions from their analyses.
Standard Deviation: Interpreting Data Spread
Standard deviation, the square root of variance, quantifies the amount of variation or dispersion in a dataset. Problems concerning standard deviation often require students to calculate it and then interpret what the value signifies about the data spread. Misinterpretation of standard deviation values can lead to erroneous conclusions, making it a critical area of focus for students.
Problems Involving Mean Deviation and Coefficient of Variation
When students encounter problems involving mean deviation, they grapple with understanding the subtle intricacies of data discrepancy. Mean deviation, or the average absolute deviation of data points from the mean, presents a deceptively simple concept that demands meticulous calculation and interpretation. It challenges students to compute precise deviations for each data point and comprehend the implications of these deviations in the context of the dataset. This process not only sharpens their mathematical skills but also hones their analytical abilities, enabling them to discern patterns and outliers effectively.
Similarly, delving into problems related to the coefficient of variation (CV) requires students to navigate the realm of relative variability. CV, as a relative measure of dispersion, compares the standard deviation to the mean, providing a normalized perspective on data variability. Tackling CV problems demands a deep understanding of both measures and a keen eye for detail. Students need to compute CV for different datasets and discern the nuances of these calculations to draw meaningful comparisons. Grappling with CV problems not only enhances their quantitative skills but also fosters a holistic understanding of data variability across diverse contexts, preparing them for more intricate statistical challenges in their academic and professional journeys.
Mean Deviation: Assessing Data Discrepancy
Mean deviation serves as a crucial measure in assessing the discrepancy of data points from the mean, offering a direct insight into the spread of a dataset. In problems related to mean deviation, students delve into the absolute differences between each data point and the mean, calculating their average. This process emphasizes the importance of understanding not only individual data points but also their relationships with the central tendency. A smaller mean deviation indicates a more consistent dataset, where data points cluster closely around the mean, while a larger mean deviation signals a more dispersed dataset. Mastering mean deviation problems hones students' ability to discern patterns within data, enabling them to draw meaningful conclusions about the dataset's overall variability and distribution.
Coefficient of Variation: Comparing Relative Variability
The coefficient of variation (CV) is a powerful statistical tool that allows us to compare the relative variability of different datasets, regardless of their units or scales. In essence, CV provides a standardized measure of dispersion, enabling meaningful comparisons between datasets of varying magnitudes. By expressing the standard deviation as a percentage of the mean, CV offers valuable insights into the consistency of data dispersion relative to the dataset's average. A low CV indicates that the data points are closely clustered around the mean, suggesting stability and reliability. On the other hand, a high CV suggests greater variability, indicating a wider spread of data points concerning the mean. Mastering the calculation and interpretation of the coefficient of variation empowers students to make informed decisions in various fields, from finance and economics to scientific research, where precise comparisons are paramount to drawing accurate conclusions.
Conclusion
In conclusion, delving into the intricacies of measures of dispersion is indispensable for students navigating the realm of statistics. Through a comprehensive exploration of problems related to range, quartiles, percentiles, variance, standard deviation, mean deviation, and coefficient of variation, students gain a profound understanding of data variability. As they grapple with these challenges, students not only hone their mathematical skills but also develop critical analytical thinking. Embracing these complexities equips students with the expertise needed to dissect real-world datasets with confidence and precision. By conquering the diverse problems posed by measures of dispersion, students pave the way for accurate statistical analyses, ensuring a strong foundation for both academic excellence and practical applications in various fields. Mastering these concepts empowers learners to decipher the language of data, making them adept problem solvers in the intricate world of statistics.