Unlocking the Power of Statistics Assignments: A Guide to Using Excel in Conjunction with Other Software
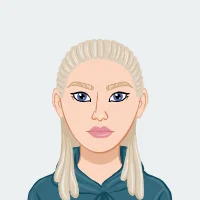
Statistics assignments often pose a formidable challenge for students, demanding not only a profound grasp of mathematical concepts but also adeptness in manipulating data with precision. Among the array of tools available, Microsoft Excel stands out as a stalwart in data analysis, providing invaluable assistance with Excel assignment. However, to truly excel in statistical assignments, it becomes imperative to synergize the capabilities of Excel with other specialized software. Throughout this blog, our exploration will traverse the landscape of data analysis, revealing how the strategic integration of Excel with other tools elevates statistical prowess. By delving into this symbiotic relationship, students can unravel the complexities of assignments, unlocking a realm of possibilities that goes beyond the capabilities of any singular tool. Join us on this journey as we unveil the potential of this dynamic integration, empowering students to navigate statistical landscapes with confidence and efficiency.
Excel Basics for Statistics
1: Understanding Excel Functions
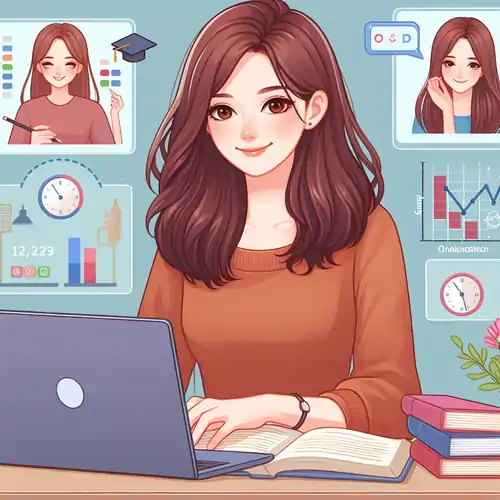
Before embarking on the journey of integrating Excel with other software, it's crucial to revisit the fundamental aspects of Excel for statistical analysis. Excel serves as a robust platform with a plethora of functions that lay the groundwork for data manipulation and interpretation. From the elementary AVERAGE and COUNT functions to more sophisticated ones like VLOOKUP and INDEX-MATCH, Excel provides a versatile toolkit for summarizing, organizing, and retrieving data. These functions form the backbone of statistical workflows, enabling students to efficiently handle datasets of varying complexities.
2: Data Cleaning and Preparation
In the realm of statistics, the adage "garbage in, garbage out" holds true. Excel, as a powerful data manipulation tool, excels in cleaning and preparing datasets for analysis. Sorting, filtering, and removing duplicates are just a few functionalities that empower students to transform raw data into a structured format, laying the groundwork for more advanced statistical analyses. A solid understanding of these Excel basics sets the stage for seamless integration with other software, enhancing the overall effectiveness of statistical assignments.
Integrating Excel with R for Advanced Analysis
1: Introduction to R
R, a robust statistical programming language, extends the analytical capabilities of Excel to unprecedented heights. Integrating Excel with R opens a gateway to advanced statistical analyses, empowering students with a formidable combination of user-friendly spreadsheet functionalities and the analytical prowess of R. With its extensive library of packages, R facilitates intricate statistical modeling and hypothesis testing, offering a more comprehensive approach to data analysis within the familiar Excel environment.
2: Setting Up R and Excel Integration
Seamlessly combining Excel with R involves leveraging the "RExcel" add-in, a conduit that facilitates smooth communication between the two platforms. Through this integration, students can effortlessly transfer data, harnessing the strengths of Excel's intuitive interface alongside R's sophisticated statistical capabilities. This symbiosis of Excel and R not only streamlines complex analyses but also provides an invaluable learning experience, bridging the gap between spreadsheet-based tasks and advanced statistical modeling. As students embark on integrating these tools, they unlock a realm of possibilities for elevating their statistical assignments to new heights.
Excel and Python: A Dynamic Duo for Data Science
In the ever-evolving landscape of data science, the integration of Excel and Python emerges as a dynamic duo, offering students unparalleled capabilities for statistical analysis. Python, renowned for its versatility and rich library ecosystem, complements Excel's user-friendly interface, creating a symbiotic relationship that transcends individual limitations.
In Excel, students can harness the power of Python's extensive libraries, such as NumPy for numerical operations, Pandas for data manipulation, and Matplotlib for data visualization. This seamless integration allows for the execution of Python code within Excel, empowering students to leverage advanced statistical functions without leaving the familiar Excel environment. The dynamic duo facilitates a smoother workflow, enabling users to perform complex analyses in Python and seamlessly transfer results back to Excel for presentation and further exploration. This synergy not only enhances efficiency but also broadens the scope of statistical analyses that students can undertake, preparing them for the multifaceted challenges of the data-driven world. As students navigate this symbiotic relationship, they unlock a new dimension of data science proficiency that goes beyond the capabilities of either tool in isolation.
Introduction to Python
Python has emerged as a highly versatile and dynamic programming language, particularly within the expansive realm of data science. Its popularity stems from its user-friendly syntax and a rich ecosystem of libraries tailored for statistical analysis. Integrating Python with Excel becomes a strategic move for students seeking to elevate their statistical capabilities, tapping into Python's robust libraries, including NumPy for numerical computations, Pandas for data manipulation, and Matplotlib for data visualization.
Bridging Excel and Python
When it comes to bridging the worlds of Excel and Python, the tool of choice is "xlwings." This Python library serves as a vital link, facilitating a seamless integration of Python code directly into Excel spreadsheets. Through xlwings, students gain the ability to execute Python's advanced data manipulation and statistical functions within the familiar Excel interface. This synergy not only simplifies the handling of complex statistical assignments but also empowers students to explore and implement sophisticated analyses with ease. As students navigate the intersection of Excel and Python, they unlock a powerful combination that opens up new horizons in statistical problem-solving.
Excel and SPSS: Bridging the Gap for Specialized Analyses
In the dynamic landscape of statistical analysis, the integration of Microsoft Excel and SPSS (Statistical Package for the Social Sciences) forms a potent alliance. SPSS, renowned for its prowess in social science research, brings specialized analytical capabilities to the table. Excel's flexibility and data management strengths, when combined with SPSS, create a synergy that is particularly valuable for students engaged in assignments requiring nuanced social science analyses.
By seamlessly bridging the gap between Excel and SPSS, students gain a comprehensive approach to handling data specific to the social sciences. The synergy extends beyond mere data transfer, allowing for a smooth transition from Excel's familiar interface to SPSS's specialized statistical functionalities. This integration ensures that students can harness the strengths of both platforms, streamlining workflows and enhancing efficiency in addressing the unique challenges posed by social science statistical assignments. As we explore the intricacies of this integration, a world of possibilities unfolds, empowering students to navigate specialized analyses with confidence and precision.
Introduction to SPSS
SPSS (Statistical Package for the Social Sciences) stands as a cornerstone in the toolkit of researchers, especially in the realm of social sciences. This specialized software offers a comprehensive suite of statistical tools tailored to meet the unique demands of social science research. By seamlessly integrating SPSS with Excel, students can harness a synergistic approach that combines the user-friendly interface of Excel with the advanced statistical capabilities of SPSS.
Exporting Data Between Excel and SPSS
Facilitating a smooth transition of data between Excel and SPSS is the "Import Data" feature, a crucial component in the integration process. This feature not only expedites the transfer of datasets but also ensures the preservation of data integrity. Importantly, it eliminates the need for manual data entry, a time-consuming process prone to errors. For students immersed in assignments demanding specialized statistical analyses common in social science research, this integration proves invaluable, providing a seamless bridge between the versatile Excel environment and the specialized analytical capabilities of SPSS.
Enhancing Visualization with Excel and Tableau
1: The Power of Visualization in Statistics
Understanding data is not just about numbers; it's about telling a compelling story through visualization. Excel's charting capabilities are robust, offering basic visual representations. However, when the need arises for more advanced and interactive visualizations, Tableau emerges as a game-changer.
2: Integrating Tableau with Excel
Tableau excels in creating dynamic, interactive dashboards that bring statistical insights to life. By exporting data from Excel to Tableau, students can seamlessly transition from static charts to engaging visual narratives. Tableau's drag-and-drop interface and extensive customization options allow for a deeper exploration of data trends, patterns, and outliers. This integration not only enhances the visual appeal of statistical assignments but also provides a platform for in-depth analysis, making the interpretation of complex data more accessible to both students and their audience.
Leveraging Excel and SQL for Database-Driven Analyses
1: Introduction to SQL
Structured Query Language (SQL) stands as a robust and versatile tool, playing a pivotal role in the management and manipulation of relational databases. Its significance becomes pronounced when dealing with statistical assignments encompassing vast datasets stored in databases. The integration of Excel with SQL serves as a strategic approach to streamline the complex processes of data retrieval and analysis.
2: Connecting Excel to SQL Databases
Leveraging Excel's Power Query feature offers students a direct pathway to connect with SQL databases effortlessly. This seamless integration facilitates not only the swift extraction of data but also its transformation within the familiar Excel environment. Whether delving into survey data, scrutinizing financial records, or engaging with any other database-driven dataset, mastering the art of Excel-SQL integration empowers students with a versatile skill set, enabling them to navigate and excel in the realm of diverse and intricate statistical assignments.
Navigating Challenges and Troubleshooting Integration
1: Common Challenges in Integration
While the prospect of integrating Excel with other software promises significant benefits, it is imperative to recognize and address potential challenges that may arise in this process. Data format discrepancies, version incompatibility, and software configuration issues are common hurdles that students may encounter. Data formats may vary between platforms, leading to complications in data transfer. Version disparities can result in features not being supported or functions behaving differently. Software configuration conflicts may arise due to settings misalignment, hindering seamless integration. Developing a comprehensive understanding of these challenges empowers students to troubleshoot effectively and ensures a smoother integration experience.
2: Tips for Smooth Integration
To facilitate a seamless integration process, students are advised to adhere to established best practices. Keeping software versions up-to-date is crucial to leverage the latest features and maintain compatibility. Double-checking data formats before and after transfer helps prevent formatting issues that may compromise the integrity of the data. Additionally, utilizing online resources and forums for troubleshooting provides a valuable support network. Proactive measures, such as staying informed about updates and seeking assistance when needed, enable students to navigate integration challenges efficiently. By addressing these considerations, students can optimize the efficiency of their integrated workflow and channel their focus towards the core aspects of statistical analysis with confidence.
Conclusion
In the dynamic realm of statistical assignments, the amalgamation of Excel with specialized software amplifies students' proficiency, instilling a robust confidence. By seamlessly integrating with R for intricate statistical modeling, harnessing Python's prowess in data science, or bridging gaps with SPSS for specialized analyses, these synergies open up novel avenues. As students navigate the intricate landscapes of statistical tasks, mastering the art of utilizing Excel in conjunction with other software emerges as a pivotal skill. This multifaceted competency not only propels academic success but also becomes an indispensable asset in their professional journeys, equipping them to meet the evolving demands of data-driven decision-making. The versatility gained from this integration empowers students to not just complete assignments but to truly excel in unraveling the complexities woven into the fabric of statistical analysis, laying a solid foundation for their future endeavors.