Understanding Statistical Power and Sample Size Determination

In the realm of scientific research and data analysis, understanding statistical power and sample size determination is paramount to conducting meaningful and reliable studies. These concepts play a critical role in the design of experiments, the interpretation of results, and the overall credibility of research findings. In this article, we will delve into the intricacies of statistical power, explore the factors that influence it, and discuss how sample size determination can impact the validity of research outcomes. If you're navigating through the complexities of statistical power and sample size determination in your research, consider seeking help with your statistical analysis assignment to ensure you approach these concepts effectively and produce robust research outcomes.
The Power of Statistical Power
Statistical power refers to the probability that a statistical test will correctly reject a false null hypothesis. In simpler terms, it's the ability of a study to detect an effect when one truly exists. A study with high statistical power is more likely to yield significant results when a real effect is present, while a study with low power is less likely to do so.
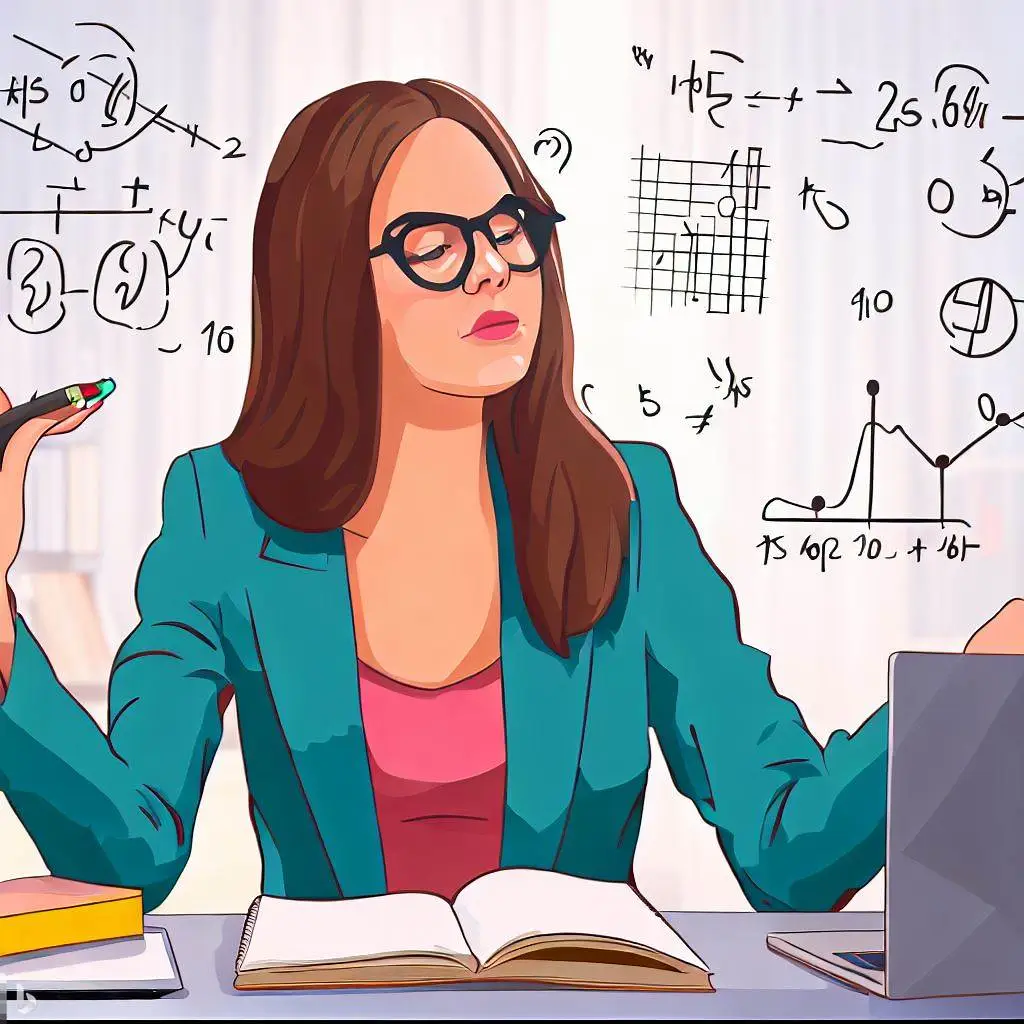
Imagine a scenario where researchers are investigating a new drug's effectiveness in treating a particular medical condition. If the study has high statistical power, it means that if the drug truly has a positive impact, the study is more likely to detect this effect. On the other hand, if the study has low power, it might fail to detect the drug's efficacy even if it's actually effective.
Statistical power is influenced by several key factors:
1. Effect Size: The Magnitude of Illumination
The first beacon of influence on statistical power is the effect size—a measure of the magnitude of the difference or relationship being studied. Picture a lighthouse shining its brilliant light across a dark sea; this light represents the effect size, and its brilliance signifies the impact of the phenomenon under investigation. A larger effect size acts as a powerful beacon, casting a wider net of influence, and making it easier for statistical tests to detect the effect. In essence, the brighter the light, the higher the power of the study to illuminate the presence of a true effect amidst the sea of data.
2. Significance Level (Alpha): Navigating the Waters of Significance
As researchers set sail in the sea of statistical analysis, they must decide upon a significance level—commonly denoted as alpha. This level acts as a threshold that the p-value, a measure of statistical significance, must surpass to declare a result as significant. Traditionally set at 0.05, this threshold determines the stringency of the test. Lowering the alpha level strengthens the study's power, allowing it to better distinguish genuine effects from the noise of randomness. Yet, this decision is not without its trade-offs. A lowered alpha heightens the risk of false negatives—erroneously failing to detect true effects due to the heightened threshold.
3. Sample Size: The Ensemble of Evidence
In the theater of research, the sample size takes center stage. It encompasses the number of participants or observations in the study and acts as the ensemble cast that brings the phenomenon to life. Just as a grand orchestra produces a richer symphony, a larger sample size enhances the study's statistical power. It provides a more comprehensive representation of the population and increases the likelihood of capturing even subtle effects. Much like the volume of an orchestra, the sample size amplifies the signal of true effects against the backdrop of noise.
4. Variability or Standard Deviation: Taming the Tides of Data
The variability or standard deviation in the data represents the undulating tides of uncertainty within a study. When these tides are turbulent, it becomes challenging to discern the true effect amidst the fluctuations. Lower variability tames these tides, creating a smoother data landscape that allows the study to more easily spot the signals of genuine effects. Thus, the calmer the seas of variability, the higher the power of the study to detect meaningful results.
5. Test Type: Choosing the Right Tools
Different statistical tests wield distinct power characteristics, akin to diverse tools in a researcher's toolbox. Just as a carpenter selects the appropriate tool for a specific task, researchers must choose the right test to match the nature of the effect they seek. Some tests are adept at detecting specific types of effects, and selecting the wrong test could be akin to wielding a wrench when a hammer is needed. The alignment between test type and the expected effect determines the test's power to uncover truth.
6. Study Design: Crafting the Blueprint
The study design serves as the blueprint for the research endeavor. It outlines the path to be traversed, the variables to be measured, and the conditions to be controlled. A well-designed study mitigates the potential for biases and confounding factors, optimizing the conditions for detecting true effects. Elements such as experimental design, randomization, and control groups impact the study's power by ensuring that the effect is not obscured by extraneous influences.
7. Effect Direction: Navigating Uncharted Territories
In the vast expanse of research, the direction of an effect can be as significant as its presence. Certain tests are better equipped to detect specific directions of effects. One-tailed tests, for instance, focus on detecting effects in a particular direction, while two-tailed tests encompass effects in both directions. Choosing the right test direction enhances the power to uncover the effect in the intended manner, allowing researchers to navigate their chosen hypothesis with precision.
The Balance: A Delicate Choreography
Amidst these influential factors, a delicate choreography unfolds. Striking a balance between effect size, significance level, sample size, variability, test type, study design, and effect direction is akin to conducting a symphony where each note must harmonize with the others. Increasing the sample size boosts power, but practical and ethical considerations must be heeded. Lowering the significance level enhances power but increases the risk of false negatives. Choosing the right test and direction aligns the study with the intended goals.
It's important to strike a balance between these factors. For instance, while increasing sample size generally increases power, there are practical and ethical considerations. Additionally, reducing the significance level might lead to more stringent requirements for significance but also increase the likelihood of missing real effects.
Sample Size Determination: The Goldilocks Principle
Determining the appropriate sample size for a study is crucial to ensure that the study has an adequate chance of detecting meaningful effects. A sample size that is too small can result in low statistical power, while a sample size that is too large might waste resources without offering substantial benefits.
Sample size determination involves finding the sweet spot, just like Goldilocks looking for the perfect porridge—not too hot, not too cold. Researchers often conduct a power analysis to estimate the sample size required to achieve a certain level of power for detecting a specified effect size. This analysis helps researchers make informed decisions about how many participants or observations are needed to conduct a meaningful study.
Several factors are taken into consideration during sample size determination:
1. Desired Power: The North Star of Confidence
In the constellation of factors, the desired power of the study shines as the North Star—a guiding light that determines the confidence researchers wish to instill in their findings. Power represents the probability of correctly detecting a true effect. Like intrepid explorers charting new territory, researchers set a course towards an acceptable level of statistical power. Often, this aim hovers around 80% or higher, ensuring that the study has a robust chance of unveiling genuine effects.
2. Effect Size: Navigating with a Compass of Expectations
As the journey unfolds, researchers must wield the compass of effect size—a gauge of the magnitude of the anticipated effect. Just as explorers rely on maps to estimate the challenges they'll face, researchers draw on prior research or pilot studies to estimate the likely effect size. This estimation is crucial, as it serves as a beacon guiding the ship towards the shores of statistical significance.
3. Significance Level: The Threshold of Discovery
Much like explorers designating landmarks along their route, researchers set a significance level—the threshold at which they consider results to be statistically significant. Often symbolized by the alpha level, this threshold impacts the required sample size. Lowering the alpha level enhances the rigor of the study, but this heightened stringency necessitates a larger sample size to maintain the desired power. Just as a challenging path requires more supplies, a more demanding significance level requires a larger sample size to achieve the same level of confidence.
4. Variability: Gauging the Waves of Uncertainty
In the tumultuous sea of data, variability—the degree of dispersion or scatter in the data—acts as the waves of uncertainty that researchers must navigate. The estimation of variability serves as a compass, indicating the level of tumult that must be overcome. Higher variability necessitates a larger sample size to ensure that the study's findings are not lost amidst the unpredictable fluctuations.
5. Study Design: The Map of Complexity
The design of the study is akin to a map outlining the intricacies of the research journey. Just as explorers must consider the topography and terrain, researchers must account for the complexity of the study design. Experimental designs, control groups, and randomization patterns all contribute to the overall sample size calculation. A more complex design, resembling a challenging route, often demands a larger crew—equivalent to a larger sample size.
6. Practical Constraints: Navigating Real-World Waters
In the world of exploration, practical considerations play a pivotal role. Resources, time, and ethical considerations collectively shape the feasibility of the journey. Similarly, the decision on sample size is deeply influenced by practical constraints. Researchers must strike a balance between the ideal sample size for maximum power and the practical limitations imposed by available resources. Just as explorers must manage supplies for the voyage, researchers must manage the resources required for data collection and analysis.
7. Expected Attrition: Accounting for the Unknown
In the unpredictable landscape of research, attrition—the departure of participants from the study—looms as a challenge. Just as explorers anticipate unforeseen obstacles, researchers must consider the possibility of attrition and its impact on the sample size. The initial sample size must account for the expected attrition rate to ensure that the study remains adequately powered even in the face of participant departures.
The Confluence of Influences: Setting Sail for Discovery
As these factors converge, the determination of an appropriate sample size transforms into a careful choreography—a dance of precision and pragmatism. The North Star of desired power, the compass of effect size, the threshold of significance, the waves of variability, the intricacies of study design, the practical constraints, and the anticipation of attrition—all these elements blend to guide the ship of research towards its destination: meaningful and reliable findings.
Real-World Implications
Insufficient statistical power and inadequate sample sizes can lead to serious consequences in the world of research. Studies with low power might fail to detect genuine effects, leading to missed opportunities for advancing knowledge or making informed decisions. Moreover, studies with small samples are more susceptible to chance fluctuations, which can lead to unreliable and irreproducible results.
On the other hand, studies with excessively large sample sizes can waste resources, both in terms of time and funding. This is especially important in fields where data collection is costly or time-consuming.
Consider a scenario where a pharmaceutical company is testing a new drug for a rare medical condition. If the sample size is too small, the study might not have the statistical power to detect the drug's potential benefits, even if they exist. Conversely, if the sample size is too large, the company might spend unnecessary resources on recruiting and monitoring participants, diverting funds from other important research initiatives.
Ethical Considerations and Human Subjects
In the context of human subjects research, there are ethical considerations related to sample size determination. It's essential to balance the need for a sufficient sample size to achieve meaningful results with the ethical responsibility to minimize risks and burdens on participants.
A study with a very small sample size might expose participants to unnecessary risks without contributing valuable insights. Conversely, a study with an excessively large sample size might involve more participants than needed, potentially subjecting them to inconveniences or burdens without proportional benefits.
Ethical guidelines and review boards often play a role in ensuring that sample size decisions are justifiable and well-considered, taking into account the potential impact on participants and the broader scientific community.
The Evolving Landscape
The fields of statistics and research methodology are constantly evolving, and so is our understanding of statistical power and sample size determination. Advances in computational power, statistical techniques, and data collection methods have led to more sophisticated approaches to power analysis and sample size estimation.
Simulation-based methods, for instance, allow researchers to simulate various scenarios to assess how different factors influence power and sample size requirements. Bayesian approaches also offer alternative perspectives on these concepts, allowing researchers to incorporate prior knowledge and uncertainties into their analyses.
Furthermore, the importance of transparency and replication in research has brought these issues to the forefront. Journals and funding agencies increasingly require researchers to justify their sample size decisions and provide detailed power analyses to ensure the robustness of their findings.
Conclusion
Statistical power and sample size determination are not mere technicalities in research; they are pivotal elements that shape the validity and impact of scientific investigations. High statistical power enhances the likelihood of detecting real effects, while appropriate sample sizes balance practical considerations with the need for meaningful insights. As researchers continue to push the boundaries of knowledge, a solid understanding of these concepts will remain essential for conducting rigorous and reliable studies that contribute to the advancement of science and society. Just as Goldilocks found the perfect porridge, researchers must find the "just right" balance in their sample size decisions to unlock the true potential of their research endeavors.