Estimation Problems in General Linear Models: A Comprehensive Guide for Statistics Students

Estimating General Linear Models (GLMs) stands at the core of statistical analysis, yet it remains a formidable challenge for many students. The intricacies of choosing the right model specification, understanding underlying assumptions, and implementing suitable estimation techniques often lead to confusion and frustration. If you need help with your Statistics assignment, this comprehensive guide aims to unravel the complexities associated with GLM estimation, offering students a clear roadmap to navigate through the intricacies of statistical modeling. By dissecting the hurdles students commonly face at every stage of the estimation process, from handling categorical variables and addressing assumption violations to selecting appropriate estimation methods, this guide provides actionable solutions. Armed with this knowledge, students can approach their statistics assignments with confidence, ensuring that their analyses are accurate, reliable, and grounded in a deep understanding of the fundamental concepts of General Linear Models.
Navigating through the maze of GLM estimation challenges is essential for any statistics student striving for excellence. This guide goes beyond the surface, delving into the nuances of model specification, assumptions, and implementation techniques. By addressing these challenges head-on, students can enhance their analytical skills and build a strong foundation in statistical modeling. With the insights provided in this guide, students will not only conquer their immediate statistics assignments but also develop a robust skill set that will serve them well in their future endeavors, whether in academic research or professional applications. Estimation problems in General Linear Models need not be daunting; armed with knowledge and understanding, students can transform these challenges into opportunities for growth and mastery in the realm of statistical analysis.
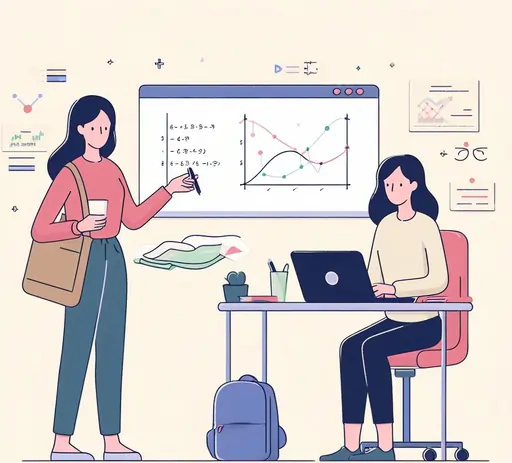
Challenges in Estimating GLMs and Their Solutions
Estimating General Linear Models (GLMs) presents multifaceted challenges that demand astute problem-solving skills from students. One major hurdle involves selecting the right model specification, where dealing with categorical variables, identifying meaningful interaction terms, and addressing multicollinearity are pivotal. Understanding the assumptions of GLMs, including normality, independence, and linearity, is another critical challenge. Detecting and managing outliers, ensuring the absence of autocorrelation in time series data, and choosing the appropriate estimation method for different data types add complexity. However, these challenges can be overcome through thorough data exploration, visualization techniques, and understanding of specialized tools such as Poisson regression for count data and autoregressive integrated moving average (ARIMA) models for time series data. By addressing these hurdles with meticulous attention and utilizing the right analytical techniques, students can successfully estimate GLMs and enhance the quality of their statistical analyses.
Choosing the Right Model Specification
Choosing the right model specification is a critical step in the estimation of General Linear Models (GLMs). One of the primary challenges students face is deciding the appropriate type of response variable and predictors for their model. Categorical variables, for instance, require careful consideration, with decisions about binary, nominal, or ordinal representations impacting the model's accuracy. Overcoming this challenge involves a keen understanding of the data's nature and applying suitable encoding methods like one-hot encoding or binary representation. Additionally, students often grapple with identifying meaningful interaction terms among predictors. Visualization techniques and exploratory data analysis play a vital role here, enabling students to graphically assess relationships and make informed decisions about which interaction terms to include in their GLM. Moreover, handling multicollinearity demands vigilance; through tools like variance inflation factor (VIF) analysis, students can pinpoint highly correlated predictors, ensuring their model remains robust. Finally, addressing missing data is pivotal. Imputation techniques such as regression imputation or mean imputation empower students to fill in missing values effectively, ensuring a complete dataset for accurate model specification. Mastering these aspects equips students with the essential skills to confidently navigate the intricate process of choosing the right model specification in GLM estimation. Estimating a GLM begins with selecting the appropriate model specification, which involves deciding the type of response variable and the predictors.
- Understanding Categorical Variables: One common hurdle is dealing with categorical variables. Students often struggle to decide whether to use binary, nominal, or ordinal variables. A solution lies in understanding the nature of the data and selecting the appropriate encoding method. For example, binary variables can be represented as 0 and 1, while nominal variables may require techniques like one-hot encoding.
- Handling Interaction Terms: Another challenge arises when incorporating interaction terms. Identifying meaningful interactions among predictors is crucial. Students can overcome this by visualizing the data and conducting exploratory data analysis. Plotting interactions helps in understanding the relationships, making it easier to decide which interaction terms to include in the model.
- Addressing Multicollinearity: Multicollinearity, the phenomenon where predictors are highly correlated, can cause estimation issues. Techniques like variance inflation factor (VIF) analysis help in detecting multicollinearity. If high multicollinearity is found, students can either remove one of the correlated predictors or consider methods like principal component analysis (PCA) to mitigate the problem.
- Handling Missing Data: Missing data is a common problem in statistics assignments. Students should address this by using imputation techniques, such as mean imputation or regression imputation, to fill in missing values. Proper handling of missing data ensures that the estimation process is not biased.
Assumptions of GLMs and Their Implications
Understanding the assumptions of General Linear Models (GLMs) is fundamental to accurate estimation and interpretation of statistical results. When these assumptions are not met, the implications can significantly impact the validity of the model. Violations such as non-linearity, lack of independence, or heteroscedasticity can lead to biased parameter estimates and unreliable predictions. One critical assumption is the normality of residuals, which affects the precision of parameter estimates and the validity of hypothesis tests. Departures from normality might necessitate data transformations or the use of non-parametric methods. Moreover, when dealing with time series data, overlooking autocorrelation can distort inference, emphasizing the importance of employing appropriate diagnostic tools like residual plots and Durbin-Watson tests. Overall, a keen awareness of these assumptions and their consequences equips students with the essential tools to diagnose issues, make informed adjustments, and enhance the robustness of their GLM estimations. Estimating a GLM also involves understanding the assumptions underlying the model and their implications on the estimation process.
- Understanding Assumption Violations: Assumptions like linearity, independence, and homoscedasticity are integral to GLMs. When these assumptions are violated, estimation results can be unreliable. To tackle this, students should employ diagnostic plots, such as residual plots, to identify violations. Addressing these issues might involve transforming variables or using robust regression techniques.
- Normality Assumption and Transformations: GLMs often assume that the residuals are normally distributed. If this assumption is violated, transformations like log or square root transformations can be applied to the response variable. Additionally, students can use non-parametric methods, like generalized additive models, which are less sensitive to the normality assumption.
- Dealing with Outliers: Outliers can significantly impact the estimation process, skewing results. Students should use visualization techniques like box plots and leverage statistical tests, such as the Z-score or the IQR method, to identify outliers. Once identified, students can decide whether to remove outliers or use robust regression methods that are resistant to outliers.
- Checking for Autocorrelation: Autocorrelation, the correlation of a variable with itself over time, can affect the estimation of GLMs, especially in time series data. Students should use autocorrelation plots and Durbin-Watson tests to detect autocorrelation. If present, techniques like differencing or autoregressive integrated moving average (ARIMA) modeling can be employed to address this issue.
Implementing Estimation Techniques
Implementing estimation techniques is a critical aspect of mastering General Linear Models (GLMs). Choosing the appropriate method, whether it's ordinary least squares (OLS), generalized least squares (GLS), or maximum likelihood estimation (MLE), hinges on a nuanced understanding of the data's characteristics. For normally distributed continuous variables, OLS is the preferred choice, while GLS comes into play when dealing with heteroscedasticity. Additionally, understanding the intricacies of different link functions, such as logit and probit, is essential for handling non-normally distributed response variables. Specialized scenarios, like count data, demand models such as Poisson regression, adjusted for overdispersion using techniques like negative binomial regression. Time series data, with its temporal dependencies, necessitates the application of autoregressive integrated moving average (ARIMA) models or state space models, making it imperative to comprehend the order of differencing and the interplay of autoregressive and moving average terms. Mastery of these techniques empowers students to accurately estimate GLMs, ensuring their statistical analyses are robust and reliable. Implementing estimation techniques involves selecting the appropriate method based on the type of response variable and the distribution of the data.
- Choosing the Right Estimation Method: The choice between ordinary least squares (OLS), generalized least squares (GLS), or maximum likelihood estimation (MLE) depends on the characteristics of the data. OLS is suitable for normally distributed continuous variables, while GLS is used when there is heteroscedasticity. MLE is versatile and can be applied to various types of data. Students should understand the data distribution and choose the appropriate method accordingly.
- Dealing with Non-Normal Data: In cases where the response variable is non-normally distributed, students can use GLMs with different link functions (e.g., logit, probit) to handle non-normality. These link functions transform the response variable to fit the GLM assumptions, allowing for accurate estimation. Proper understanding and application of these link functions are essential for estimating GLMs successfully.
- Handling Count Data: Count data, common in fields like biology and finance, require specialized models such as Poisson regression. Poisson regression is suitable for count data, assuming that the counts follow a Poisson distribution. If overdispersion is present (variance greater than the mean), students can opt for negative binomial regression, a variation of Poisson regression that accounts for overdispersion.
- Dealing with Time Series Data: Time series data present unique challenges due to temporal dependencies. Students can use autoregressive integrated moving average (ARIMA) models or state space models to account for these dependencies. Understanding the order of differencing, autoregressive terms, and moving average terms is crucial when applying ARIMA models to time series data.
Conclusion
In conclusion, mastering the complexities of General Linear Model estimation is fundamental for students undertaking statistics assignments. Navigating the challenges related to model specification, assumptions, and implementation techniques is crucial. By understanding the intricacies of handling categorical variables, addressing multicollinearity, and dealing with assumption violations, students can enhance the accuracy of their analyses. Additionally, selecting the right estimation method based on the data's characteristics, such as ordinary least squares (OLS) for normally distributed continuous variables or Poisson regression for count data, is pivotal. Equally important is the adept use of visualization tools and diagnostic techniques to identify outliers, assess residuals, and check for autocorrelation. Armed with this knowledge, students can approach their statistics assignments with confidence, ensuring they produce robust, reliable, and insightful results.