Simple Linear Regression: Mastering Statistics Assignments

Simple Linear Regression stands as a cornerstone in the realm of statistics, offering a fundamental framework for understanding the intricate relationships between variables. In the world of academia and real-world applications, mastering this essential concept is pivotal. This comprehensive guide serves as your compass, navigating through the complexities of Simple Linear Regression and equipping you with the expertise needed to excel in Linear Regression assignments. From unraveling the basic principles to delving into real-world applications, this guide provides a deep dive, ensuring you gain the confidence and skills necessary to analyze data, draw meaningful insights, and solve intricate problems with precision and finesse. Embark on this journey, and empower yourself to navigate the statistical landscape with ease and confidence.
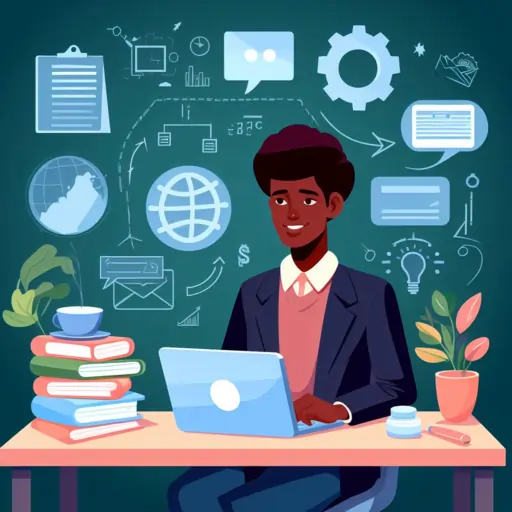
Basics of Simple Linear Regression
Understanding the basics of Simple Linear Regression is akin to deciphering the language of data. At its core, this technique elucidates the relationship between two variables, revealing patterns that form the backbone of statistical analysis. By comprehending the underlying principles, students gain a profound insight into how changes in one variable correspond to predictable shifts in another. From the foundational equation to the critical assumptions underpinning regression analysis, this section delves deep into the essence of Simple Linear Regression. It equips learners with the fundamental knowledge needed to construct regression models, interpret their results, and apply this invaluable tool to unravel intricate data puzzles. Mastering these basics not only paves the way for a solid grasp of statistics but also lays the foundation for exploring more advanced analytical techniques, enabling students to navigate the vast landscape of data analysis with confidence and precision.
What is Simple Linear Regression?
Simple Linear Regression, a fundamental statistical method, offers a structured approach to understanding the relationship between two continuous variables. At its core, it investigates how changes in one variable, known as the independent variable, directly influence changes in another variable, referred to as the dependent variable. The essence of Simple Linear Regression lies in its assumption of a linear connection between these variables, indicating that a consistent change in the independent variable results in a proportional shift in the dependent variable. Through this method, analysts can establish a regression equation that best fits the data, enabling precise predictions and valuable insights. Whether it's predicting sales based on advertising expenditure or understanding the impact of study hours on exam scores, Simple Linear Regression serves as a foundational tool, providing a clear and quantitative understanding of relationships within datasets.
Assumptions of Simple Linear Regression
Ensuring the veracity of Simple Linear Regression demands adherence to several critical assumptions. Linearity mandates a direct proportional relationship between the variables, while independence emphasizes the autonomy of each observation. Homoscedasticity necessitates consistent variability across all levels of the independent variable, ensuring the stability of predictions. Normality in residuals facilitates accurate hypothesis testing. Lastly, multicollinearity cautions against high correlations among predictors, safeguarding the integrity of regression coefficients.
Calculating Regression Coefficients
The process of calculating regression coefficients in Simple Linear Regression is at the heart of statistical modeling. These coefficients, represented as b0 (the y-intercept) and b1 (the slope of the regression line), encapsulate the essence of the relationship between variables. The computation involves intricate mathematical techniques, notably the method of least squares, which minimizes the sum of squared residuals. In essence, the regression line is crafted to minimize the discrepancies between observed data points and their corresponding values predicted by the model. This meticulous calculation not only refines the model's accuracy but also forms the basis for making reliable predictions. Mastery over this process empowers analysts to derive meaningful interpretations from data, making it a pivotal skill in the arsenal of any data scientist or statistician. Understanding how to calculate these coefficients equips students with the ability to construct robust regression models, providing a solid foundation for advanced statistical analyses and informed decision-making.
Interpreting Regression Output
Interpreting regression output is akin to deciphering a statistical narrative. The coefficients unravel the quantitative impact of the independent variable on the dependent variable. R-squared, ranging from 0 to 1, gauges the goodness of fit, indicating the proportion of variance explained by the model. P-values validate the significance of coefficients, guiding researchers in discerning variables of consequence. Proficiency in this interpretation equips students with the acumen to discern patterns, make predictions, and substantiate their findings effectively.
Applications of Simple Linear Regression
The applications of Simple Linear Regression span across a diverse array of fields, playing a pivotal role in transforming raw data into actionable insights. Predictive analysis stands as one of its primary applications, enabling businesses to forecast trends and make data-driven decisions. In the realm of trend analysis, Simple Linear Regression provides the lens through which historical data can be dissected, allowing analysts to identify patterns and anticipate future trajectories. Moreover, it serves as a powerful tool for error detection and outlier identification, ensuring the integrity of datasets by flagging irregularities. Additionally, Simple Linear Regression facilitates nuanced comparisons between variables, aiding researchers and decision-makers in understanding the relative impact of different factors. From finance to healthcare, this versatile technique empowers professionals to extract valuable knowledge from data, making it an indispensable asset in the realm of statistical analysis.
Predictive Analysis
Simple Linear Regression finds its zenith in predictive analysis. By extrapolating patterns from historical data, students can forecast future trends, aiding businesses in strategic planning and informed decision-making. Predictive models underpin a myriad of sectors, from finance to healthcare, reshaping industries through data-driven insights.
Trend Analysis
Trend analysis, an indispensable facet of Simple Linear Regression, empowers students to discern evolving patterns over time. By scrutinizing historical data, they unravel trends essential for anticipating market dynamics. This application provides a compass for businesses and policymakers, guiding them in adapting strategies to align with future trajectories.
Error Detection and Outlier Identification
Regression analysis acts as a magnifying glass, enabling students to identify errors and outliers concealed within datasets. Residual analysis illuminates deviations from the regression line, flagging potential anomalies. Addressing these outliers is pivotal, ensuring statistical models reflect the true nature of the data and enhancing the credibility of analysis outcomes.
Comparison of Variables
Simple Linear Regression facilitates a nuanced comparison between variables. By juxtaposing the influence of diverse predictors on the dependent variable, students gain insights into the relative significance of factors. This comparative analysis, be it in marketing strategies or scientific experiments, equips decision-makers with invaluable insights, enabling them to channel resources judiciously.
Challenges and Solutions in Simple Linear Regression
While Simple Linear Regression offers invaluable insights, mastering its nuances comes with its set of challenges. Students often grapple with the complexity of mathematical derivations and interpretations, hindering their understanding of regression coefficients and their significance. Moreover, identifying and rectifying assumption violations, a common occurrence in real-world datasets, requires a discerning eye. Additionally, the translation of theoretical knowledge into practical applications, especially in selecting pertinent variables, demands critical analytical thinking. However, these challenges can be surmounted through interactive learning tools and tutorials, enabling students to visualize abstract concepts effectively. Real-world examples and case studies bridge the gap between theory and application, providing context for complex statistical theories. Collaborative study groups foster an environment for shared learning and problem-solving, enhancing students' ability to tackle challenging concepts. Furthermore, regular practice with hands-on exercises hones analytical skills, equipping students with the confidence to navigate the complexities of Simple Linear Regression and emerge as adept statisticians.
Common Challenges Faced by Students
Mathematical Complexity: The intricate derivations and calculations involved in Simple Linear Regression often pose a formidable challenge to students.
- Interpretation Dilemmas: Grappling with the interpretation of regression coefficients and p-values can lead to confusion, hindering the extraction of meaningful insights.
- Assumption Violations: Recognizing and addressing violations of regression assumptions, common in real-world datasets, require a discerning eye.
- Application Difficulties: Translating theoretical knowledge into practical applications and selecting pertinent variables demand critical analytical thinking.
Solutions and Strategies for Overcoming Challenges
- Interactive Learning: Engaging in interactive learning through software tools and interactive tutorials demystifies complex concepts, fostering a deeper understanding of regression techniques.
- Real-World Examples: Immersing students in real-world examples and case studies bridges the gap between theory and application, enhancing comprehension and igniting curiosity.
- Collaborative Study Groups: Creating an environment conducive to collaborative learning enables students to pool their knowledge, discuss challenges, and learn from diverse perspectives.
- Practice and Hands-On Exercises: Regular practice with datasets and hands-on exercises hones students' analytical skills, imbuing them with the confidence to tackle intricate statistical problems with finesse.
Conclusion
In essence, Simple Linear Regression transcends mere mathematical computations; it embodies the art and science of distilling meaningful narratives from data. Mastery over its intricacies empowers students to decipher patterns, forecast trends, and substantiate their hypotheses with empirical evidence. As students embark on their statistical journey, the knowledge gleaned from Simple Linear Regression serves as a beacon, guiding them through the labyrinthine realm of data analysis. Armed with this proficiency, students emerge not merely as statisticians but as architects of informed decisions, steering industries and research endeavors toward a future illuminated by data-driven insights.