Exploring Real-World Applications of Poisson Processes: Insights from Statistics Assignment Help Experts

Section 1: Understanding Poisson Processes
Definition and Features:
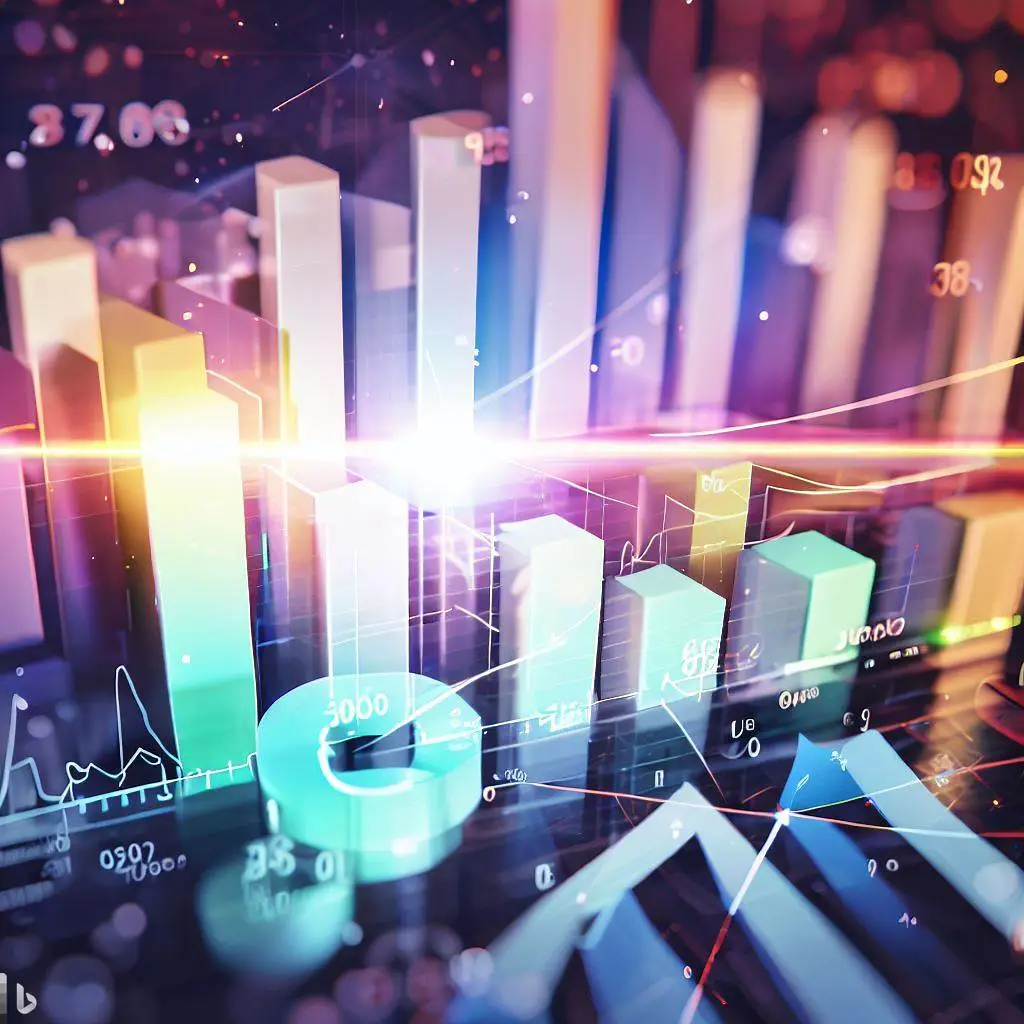
Parameters of the Poisson Process:
The intensity parameter (lambda), denoted by, is the only parameter that controls the Poisson process. The average rate of events is represented by this parameter. It establishes the Poisson process's mean and variance. In particular, the average number of events in a given period of time or space is represented by the Poisson process mean, which is equal to. A measure of the spread or dispersion of event occurrences around the mean is provided by the variance of the Poisson process, which is also equal to.
Section 2: Applications in Telecommunications
Analysis of Call Centers:
The analysis of call centers is one of the many areas in which Poisson processes are extensively used in telecommunications. Statisticians can forecast call volumes and tailor staffing needs by modeling customer arrival rates as a Poisson process. Call centers can estimate the intensity parameter by looking at historical data on call arrival patterns, which enables them to efficiently manage their resources and adhere to service level agreements. Additionally, Poisson processes can be used for service level analysis to determine the percentage of calls that are answered within a predetermined amount of time, allowing call centers to track and enhance their customer service performance.
Analysis of Network Traffic:
Poisson processes are frequently used in network traffic analysis. Researchers and network administrators can learn more about packet arrival rates and anticipate potential congestion problems by modeling the arrival of network packets as a Poisson process. Poisson processes' constant arrival rate presumption fits the network traffic's ad hoc nature well, enabling precise capacity planning and routing algorithm optimization. Additionally, Poisson process-based models make it possible to assess Quality of Service (QoS) variables like packet loss rates and delays, which are crucial for preserving high network performance.
Section 3: Financial Applications
Modeling Credit Risk:
For the purpose of modeling credit risk, Poisson processes are essential. Financial institutions can predict the likelihood of default for a specific portfolio or client by treating credit events like defaults or loan delinquencies as a Poisson process. These models make it possible for banks and lending organizations to efficiently evaluate and handle credit risk. Financial institutions can determine important credit risk metrics, like the expected loss given default, to help with portfolio management and risk assessment. This is done by looking at historical data on default rates and estimating the intensity parameter.
Analysis of the stock market:
The field of stock market analysis includes the use of Poisson processes. The random and erratic nature of price movements can be captured by researchers by modeling the arrival of stock price changes as a Poisson process. Poisson jump models, for example, extend the fundamental Poisson process framework by including arbitrary jumps in stock prices. These models make it possible to estimate volatility more precisely and make it easier to create high-frequency trading strategies. Investors and traders can make well-informed decisions about portfolio management and trading strategies by comprehending the stochastic nature of stock price movements.
Section 4: Healthcare Applications
Modeling an Epidemic:
In modeling and forecasting the spread of infectious diseases, Poisson processes are incredibly useful. Epidemiologists can calculate disease transmission rates and evaluate the effects of different interventions, such as vaccination campaigns or social isolation measures, by viewing the emergence of new infections as a Poisson process. These models help in developing public health strategies, understanding the efficacy of control measures, and forecasting the course of epidemics. Healthcare professionals and policymakers can reduce the impact of epidemics by analyzing historical data on infection rates and estimating the intensity parameter.
Planning hospital resources:
Hospitals and healthcare facilities can better allocate resources and staffing by using Poisson processes to model patient arrival rates in healthcare settings. Statistics experts can estimate the intensity parameter and create models that help with capacity planning, analyzing the wait times in emergency rooms, and improving operational effectiveness by looking at historical data on patient arrivals. With the aid of these models, healthcare administrators can allocate resources wisely, ensuring that the necessary staff and facilities are on hand to meet patients' needs while reducing wait times and maximizing patient flow.
Section 5: Queuing Theory Applications
Analysis of Waiting Time:
Poisson processes are widely used in queueing theory to model arrival and service times. Queuing theorists can analyze and forecast various performance metrics by assuming that customer arrivals follow a Poisson process and service times follow a different distribution, such as exponential or Erlang. Average wait times, queue lengths, and server utilization rates are some of these metrics. By choosing the ideal number of servers, assessing the effects of various scheduling algorithms, and reducing customer wait times, Poisson process-based models help to optimize queue management strategies.
Transport Infrastructure:
Poisson processes are used to simulate traffic patterns and assess arrival rates at transportation hubs like train stations and airports. The arrival of vehicles or passengers can be viewed as a Poisson process, which allows transportation planners to estimate travel times, gauge the amount of congestion, and decide on the best course of action for infrastructure upgrades or scheduling alterations. Planners can optimize transportation systems, increase productivity, and improve the overall passenger experience by comprehending the random nature of arrivals.
Section 6: Advanced Topics and Poisson Process Extensions
Non-Homogeneous Poisson Processes:
Non-homogeneous Poisson processes allow for time-varying arrival rates in contrast to the standard Poisson process, which assumes a constant intensity parameter over time or space. These models have uses in a variety of industries, such as finance, telecommunications, and environmental science, where event rates can vary over time or space. Non-homogeneous Poisson processes offer a more adaptable framework for simulating actual situations where event rates vary over time or space.
Processes with Compound Poisson:
By adding random magnitudes to arrival events, compound Poisson processes expand the scope of the original Poisson process. In these models, events occur according to a Poisson process, but their sizes or magnitudes are controlled by a different distribution. In modeling insurance claims, where the arrival of claims is modeled as a Poisson process and the claim amounts follow a different distribution, compound Poisson processes are frequently used. These models make it easier to estimate overall claims, assess risk, and calculate related metrics like expected claim costs.
Restrictions and Complements:
Although Poisson processes provide a potent framework for simulating random events, it's critical to recognize their presumptions and constraints. Poisson processes, for instance, make the assumption that events are independent of one another and of a constant intensity parameter, which may not always be true in real-world situations. Alternative stochastic processes, like Markov processes or renewal processes, may offer more accurate representations in cases where these presumptions are broken. To choose the most suitable stochastic process, researchers and analysts must carefully consider the context and nature of the phenomena being modeled.
Conclusion:
Poisson processes are crucial tools for comprehending and analyzing random events in a variety of contexts. These procedures have numerous uses in modeling, prediction, and decision-making across a variety of industries, from telecommunications and finance to healthcare and queuing theory. We have looked at the useful implications and advantages of using Poisson processes in real-world contexts by incorporating the knowledge of Statistics Assignment Help experts. Poisson processes will continue to be a useful tool for researchers, analysts, and decision-makers as our understanding of probability and statistics develops, allowing us to gain a deeper understanding of the random events that shape our world.