Text Mining and Sentiment Analysis in SPSS Assignments: Extracting Insights from Text Data

In the ever-evolving realm of data analysis, the indispensability of text mining and sentiment analysis has become increasingly pronounced, especially when seeking assistance with SPSS assignments. These robust methodologies serve as formidable tools for gleaning valuable insights from the often intricate and unstructured landscape of textual data. This blog is dedicated to delving into the multifaceted application of these analytical techniques within the context of SPSS assignments, serving as a compass for students navigating the complexities of text data analysis.
Text mining, as a foundational aspect of this discourse, entails the systematic extraction of meaningful information from unstructured text data. Within the purview of SPSS assignments, this can encompass diverse textual sources, ranging from open-ended survey responses to customer reviews or social media comments. The overarching objective is to metamorphose raw textual data into a structured format conducive to rigorous analysis, ensuring students receive the necessary assistance with SPSS assignments to decipher and analyze the intricacies of their data.
Similarly, sentiment analysis, a pivotal facet of text mining, seeks to discern the emotional tenor embedded within a piece of text. By categorizing sentiments as positive, negative, or neutral, students engaging in SPSS assignments can unravel deeper insights from customer feedback, product reviews, or any corpus of text-based data carrying inherent sentiment value.
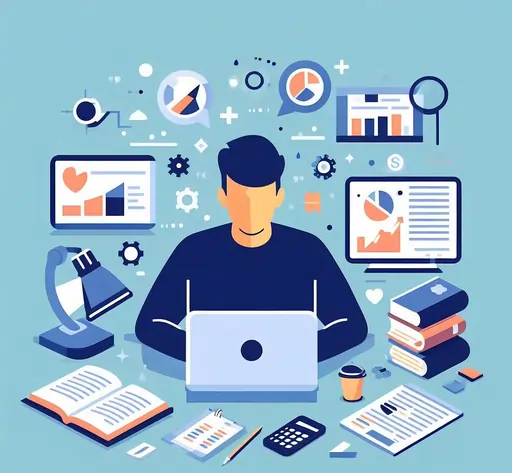
This comprehensive guide aims to equip students with the requisite knowledge and practical insights essential for augmenting their analytical prowess in the realm of SPSS assignments. By traversing the intricate terrain of text mining and sentiment analysis, students can not only unravel the latent potential within their data but also cultivate skills that are increasingly vital in the dynamic landscape of contemporary data analysis and receive valuable assistance with their SPSS assignment.
Understanding Text Mining in SPSS Assignments
Text mining in the context of SPSS assignments involves a systematic approach to extracting valuable insights from unstructured text data. As students embark on this journey, they delve into the intricacies of data collection and preparation, understanding the importance of transforming raw text into a format compatible with SPSS tools. The process includes tokenization, breaking down text into meaningful units, and leveraging SPSS's Text Analytics for Surveys module to ensure the data is ready for analysis. This section serves as a foundational guide for students, equipping them with the essential skills to navigate the complexities of text mining within the SPSS environment.
Introduction to Text Mining
Text mining, a transformative process in SPSS assignments, revolves around extracting valuable insights and patterns from unstructured textual data. In the realm of SPSS tasks, it finds application in diverse textual formats, spanning open-ended survey responses, customer reviews, and social media comments. The primary objective lies in converting raw text into structured data, laying the groundwork for in-depth analysis. This dynamic approach equips students with the ability to decipher complex narratives, unveiling hidden trends and patterns. Whether navigating through the nuances of survey data or unraveling sentiments within customer feedback, text mining in SPSS assignments becomes an indispensable skill, bridging the gap between unstructured information and actionable insights.
Text Mining Process in SPSS
- Data Collection and Preparation
- Text Transformation and Tokenization
The first step in text mining is collecting relevant textual data. This can be done through surveys, web scraping, or accessing existing datasets. Once the data is collected, it needs to be preprocessed to remove noise, irrelevant information, and standardize the format. In SPSS, this involves using the Text Analytics for Surveys module.
Text transformation involves converting raw text into a format suitable for analysis. Tokenization is a crucial step where the text is broken down into smaller units, such as words or phrases. SPSS provides tools to perform these tasks efficiently, enabling students to prepare their data for in-depth exploration.
Sentiment Analysis in SPSS Assignments
Sentiment analysis plays a pivotal role in the realm of SPSS assignments, offering students a unique lens through which to unravel the emotional intricacies woven into textual data. This section delves into the multifaceted landscape of sentiment analysis, providing students with a comprehensive understanding of its nuances within the SPSS environment. Here, students are introduced to the robust tools that SPSS offers for both lexicon-based and machine learning-based approaches to sentiment analysis. As students navigate the categorization of sentiments into positive, negative, or neutral, they acquire a powerful skill set enabling them to unearth profound insights from an array of sources, ranging from customer feedback to product reviews. This proficiency not only amplifies their analytical acumen but also enriches their toolkit, positioning them to tackle the complexities of sentiment analysis with confidence and finesse.
Introduction to Sentiment Analysis
Sentiment analysis, a pivotal aspect of modern data analytics, is designed to discern the emotional tone embedded in a given piece of text, providing insights into whether the sentiment is positive, negative, or neutral. Within the realm of SPSS assignments, sentiment analysis finds practical application in diverse scenarios, such as assessing customer feedback, evaluating product reviews, and analyzing any text-based data where gauging sentiment is paramount. This analytical technique empowers students to unravel the subjective aspects of textual information, contributing to a more comprehensive understanding of the data. By employing sentiment analysis in SPSS, students can uncover patterns, trends, and sentiments within large datasets, enabling them to draw meaningful conclusions and make informed decisions based on the emotional nuances conveyed through the text. Mastering sentiment analysis in SPSS equips students with a valuable skill set applicable to various industries, reinforcing the significance of incorporating this technique into their analytical toolkit.
SPSS Tools for Sentiment Analysis
- Lexical-Based Approaches
- Machine Learning-Based Approaches
SPSS offers lexical-based approaches for sentiment analysis, where predefined dictionaries are used to assign sentiment scores to words. This allows students to analyze large volumes of text efficiently. They can explore how sentiment varies across different groups or time periods, providing valuable insights for decision-making.
For a more advanced analysis, students can delve into machine learning-based sentiment analysis in SPSS. This involves training models on labeled datasets to predict sentiment in new, unseen text. SPSS Modeler provides a user-friendly interface for building and deploying machine learning models, making it accessible for students with varying levels of expertise.
Practical Implementation Tips for SPSS Assignments
Guiding students beyond theoretical concepts, this section offers practical implementation tips specifically tailored to SPSS assignments. Selecting the appropriate text mining techniques is paramount, demanding a nuanced understanding of the data at hand. Students gain insights into the intricacies of SPSS, empowering them to navigate real-world challenges such as misspellings and slang. Armed with this knowledge, they become adept at addressing the subtleties of text data, ensuring that their text mining endeavors in SPSS assignments are not only effective but also efficient. By delving into the practical aspects, students can confidently apply their skills, transforming theoretical knowledge into actionable insights that contribute meaningfully to their academic and professional growth.
Choosing the Right Text Mining Techniques
Before embarking on the analysis journey, it is imperative for students to delve into the intricacies of the text data they are working with. A critical factor is recognizing that different text mining techniques suit various types of data. Factors such as dataset size, language complexity, and specific analysis objectives should be meticulously considered. This thoughtful approach ensures a harmonious alignment between the chosen techniques and the assignment's overarching goals. Whether dealing with voluminous customer reviews or succinct social media comments, understanding the nuances of the data paves the way for more effective and insightful analyses.
Handling Challenges in Text Mining
Text mining, though powerful, is not without its hurdles. Challenges like misspellings, slang, or context-specific language can complicate the analysis process. In the realm of SPSS assignments, students need to be cognizant of these challenges and actively seek strategies to overcome them. This might involve employing text preprocessing techniques to clean and standardize the data, incorporating domain knowledge to contextualize language nuances, or leveraging external resources such as language-specific dictionaries to augment the accuracy of their analysis. Navigating these challenges adeptly not only refines the analytical process but also hones valuable problem-solving skills essential for real-world applications.
Enhancing Interpretation and Visualization
In the realm of SPSS assignments, the journey doesn't end with analysis; it extends to interpretation and visualization. This section empowers students to create meaningful visualizations using SPSS, transforming their analytical findings into compelling narratives. By focusing on effective communication of insights, students refine their skills in conveying the implications of text mining and sentiment analysis to diverse stakeholders, solidifying their role as proficient data analysts.
As students navigate the interpretative phase, they should consider utilizing a variety of visual elements within SPSS, such as charts, graphs, and tables. These tools not only enhance the aesthetic appeal of their reports but also facilitate a deeper understanding of complex patterns and trends. An insightful visualization can elucidate the nuances of sentiment fluctuations over time or highlight distinctive patterns within different textual categories. Through these visual narratives, students can eloquently articulate their analytical journey, ensuring that their findings resonate with both technical and non-technical audiences. In doing so, they not only fulfill the academic requirements of their assignments but also cultivate a skill set crucial for effective communication in the professional realm.
Creating Meaningful Visualizations
In the realm of SPSS assignments, the importance of interpretation extends beyond the intricacies of analysis. As students navigate the diverse landscape of text mining and sentiment analysis, leveraging SPSS's robust visualization capabilities becomes paramount. Crafting visuals that are both clear and compelling enhances the impact of their findings. Whether illustrating a sentiment trend evolving over time or making nuanced comparisons across different demographic groups, the right visualization can elucidate patterns that might be obscured in raw data. This not only aids in understanding complex relationships but also facilitates effective communication of findings to diverse audiences.
Communicating Insights Effectively
The culmination of any SPSS assignment lies in the art of communication, transcending mere technical analysis. Once students have unraveled the insights from their text mining and sentiment analysis, the challenge is to translate these revelations into actionable recommendations. This step is integral, whether the goal is to elevate customer satisfaction, fine-tune marketing strategies, or pinpoint areas for product enhancement. The ability to communicate insights effectively transcends data proficiency, becoming a valuable skill set for students aiming to impact decision-making processes with their analytical prowess. By presenting findings in a coherent and actionable manner, students not only showcase their technical skills but also demonstrate the real-world applicability of their insights.
Conclusion
In conclusion, the integration of text mining and sentiment analysis within SPSS assignments not only provides students with a unique opportunity to engage with real-world, unstructured data but also serves as a pivotal gateway to advancing their analytical proficiency. As students grasp the foundational principles of text mining and adeptly navigate sentiment analysis techniques, they gain a deeper understanding of the complexities inherent in textual data. Embracing practical implementation tips further empowers students to apply their knowledge in diverse scenarios, fostering a comprehensive skill set. In the dynamic landscape of data analytics, where continual evolution is the norm, the ability to proficiently analyze text data stands as a paramount skill for aspiring data analysts and researchers. As industries increasingly rely on nuanced insights from unstructured information, students equipped with these capabilities are not merely analysts; they are adept navigators shaping the future of data-driven decision-making.