Claim Your Offer
Unlock an exclusive deal at www.statisticsassignmenthelp.com with our Spring Semester Offer! Get 10% off on all statistics assignments and enjoy expert assistance at an affordable price. Our skilled team is here to provide top-quality solutions, ensuring you excel in your statistics assignments without breaking the bank. Use Offer Code: SPRINGSAH10 at checkout and grab this limited-time discount. Don’t miss the chance to save while securing the best help for your statistics assignments. Order now and make this semester a success!
We Accept
- Understanding the Basics
- Data Entry and Import
- Inputting Data in SPSS
- Importing External Data
- Variable Transformation and Recoding
- Variable Transformation
- Recoding Variables
- Cleaning and Managing Data
- Handling Missing Data
- Identification of Missing Data
- Imputation Techniques
- Data Screening and Validation
- 1. Outlier Detection
- 2. Data Validation Checks
- Advanced Data Management Techniques
- Merging and Aggregating Data
- Merging Datasets
- Aggregating Data
- Automation through Syntax
- Writing and Executing Syntax
- Creating and Using Syntax Templates
- Conclusion
In the realm of statistical analysis, SPSS (Statistical Package for the Social Sciences) stands as a formidable tool for students and researchers alike. As students navigate through their academic journey, mastering SPSS is often a crucial component. Effective data management is at the heart of SPSS proficiency, ensuring accurate and reliable results in assignments and research projects. In this blog post, we will delve into the best practices for data management in SPSS, providing students with a comprehensive guide to enhance their skills.
As the cornerstone of statistical exploration, SPSS empowers students to unravel complex datasets, draw meaningful insights, and make informed decisions. The significance of adept data management cannot be overstated, as it forms the bedrock upon which successful analyses are built. By adhering to best practices, students not only enhance the robustness of their findings but also cultivate a skill set that extends beyond academia, serving them well in their future endeavors. For those seeking assistance with SPSS assignment, the sections that follow will explore essential facets of data entry, transformation, cleaning, and advanced techniques, equipping students with the knowledge to navigate the intricacies of SPSS seamlessly.
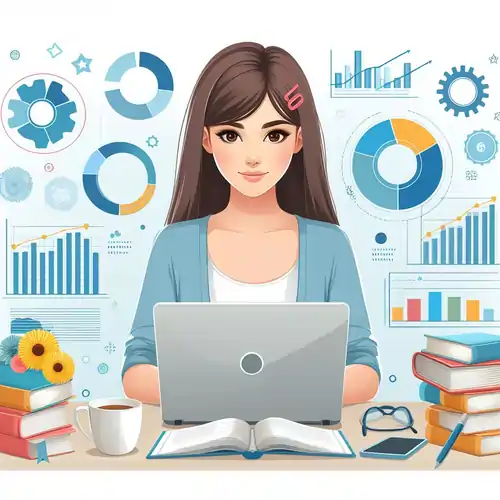
Understanding the Basics
As students embark on their SPSS journey, grasping the fundamental aspects lays the groundwork for successful data management. This section provides insights into the core principles that set the stage for proficient SPSS utilization.
Developing a solid foundation begins with comprehending the intricacies of data entry and import. Whether inputting data directly into SPSS or importing external datasets, meticulous attention to detail ensures accuracy. Understanding variable transformation and recoding is equally vital, as it enables students to adapt variables to the specific requirements of their analyses. Emphasizing these foundational principles, students gain the confidence to navigate the complexities of SPSS, paving the way for a more robust and insightful data management experience.
Data Entry and Import
Efficient data entry and importation are pivotal skills in SPSS. This section delves into the nuances of inputting data directly into SPSS and importing external datasets seamlessly. Understanding these processes ensures a solid foundation for subsequent analyses. When inputting data directly, students can navigate to the "Variable View," where they define variables by specifying names, types, and measurement levels. Switching to the "Data View," they input actual data, paying close attention to data types. For external datasets, SPSS offers multiple import options through the "File" menu, facilitating a smooth transition by selecting the appropriate data format and verifying data upon importation. These fundamental steps lay the groundwork for proficient data handling and analysis, a cornerstone for academic success.
Inputting Data in SPSS
Before diving into the intricacies of data management, it's essential to grasp the fundamentals of entering data into SPSS. Students often encounter datasets in various formats, ranging from Excel spreadsheets to CSV files. SPSS allows for seamless importation of these datasets, facilitating a smooth transition into the analysis phase.
To input data directly into SPSS, navigate to the "Variable View" and define the variables by specifying their names, types, and measurement levels. Then, switch to the "Data View" to input the actual data. Pay close attention to data types, ensuring numerical variables are correctly designated as such.
Importing External Data
When dealing with datasets stored externally, SPSS presents a plethora of import options accessible through the "File" menu, streamlining the data import process for students. The significance of selecting the right data format cannot be overstated, as it lays the foundation for accurate analyses. It is paramount for students to meticulously verify the imported data, scrutinizing it for anomalies or discrepancies. This careful examination ensures that the dataset aligns with the intended analysis, fostering a seamless integration of external data into SPSS and enhancing the overall reliability of the statistical procedures undertaken.
Variable Transformation and Recoding
Variable transformation and recoding are indispensable for tailoring variables to specific analysis requirements. This section explores the tools SPSS provides for transforming variables mathematically or recoding them to align with analytical goals, facilitating a nuanced approach to data manipulation.
In the realm of variable transformation, SPSS offers a diverse set of mathematical operations, empowering students to derive new variables based on complex expressions. Whether it's creating composite scores, standardizing variables, or applying logarithmic transformations, students can leverage SPSS to implement these operations seamlessly.
Recoding, on the other hand, allows students to redefine variable values to better suit the analytical context. SPSS simplifies this process with its user-friendly interface, enabling students to collapse categories, recategorize values, or create entirely new variables based on logical conditions.
By mastering these tools, students gain the flexibility to tailor variables precisely, ensuring their datasets align with the nuanced demands of sophisticated statistical analyses. This versatility is a key asset as students engage in diverse research projects and assignments that demand a tailored and precise approach to variable manipulation.
Variable Transformation
In numerous academic scenarios, students are confronted with variables in their raw state that might not align with the prerequisites of their analysis. SPSS stands as a comprehensive solution, offering a diverse array of tools for variable transformation. This functionality empowers students to craft new variables through the application of mathematical expressions or logical conditions. Such transformations prove invaluable, especially when creating composite scores or converting units of measurement to harmonize with the analytical framework at hand. This adaptability within SPSS ensures that students can tailor variables precisely to their analytical needs, fostering a nuanced and insightful approach to data manipulation.
Recoding Variables
The process of recoding is a common necessity for students working with SPSS, involving the conversion of existing variable values into new categories. Students often encounter situations where recoding is essential, such as simplifying categories for clarity or redefining values to align with the research context. SPSS streamlines this procedure through the "Recode into Different Variables" option, offering a user-friendly interface to handle such transformations. This ensures that the recoded variables seamlessly integrate into the broader analysis, maintaining the coherence and integrity of the dataset throughout the research journey.
Cleaning and Managing Data
A clean dataset is indispensable for conducting robust analyses that yield meaningful insights. This section serves as a guide for students, equipping them with essential techniques for handling missing data, identifying outliers, and conducting thorough validation checks. Addressing missing data is a critical step; by utilizing SPSS tools to identify and strategically handle missing values, students can mitigate potential biases in their analyses. Identifying outliers is equally pivotal, and SPSS offers tools such as boxplots and Z-scores for accurate detection. Furthermore, conducting validation checks, including range, consistency, and frequency checks, ensures the dataset's accuracy and reliability.
Armed with these skills, students can navigate the intricacies of data management, cultivating datasets that serve as a solid foundation for their analyses. By upholding the principles of data integrity and accuracy, students set the stage for successful and insightful statistical explorations in their academic pursuits.
Handling Missing Data
Missing data is a pervasive challenge in statistical analysis, demanding meticulous attention from students to ensure the integrity of their results. Identifying missing values is the initial step in this process, and SPSS provides indispensable tools for this purpose. By navigating to the "Analyze" menu and selecting "Descriptives," students can obtain comprehensive statistics on missing values for each variable, laying the groundwork for informed decision-making.
Once missing data is identified, students face the critical task of implementing suitable imputation techniques. SPSS offers a repertoire of methods, including mean imputation, regression imputation, and multiple imputation. Understanding the nuances of each technique empowers students to choose the most appropriate strategy based on their dataset's characteristics.
By navigating this terrain with proficiency, students can fortify their analyses against the pitfalls of missing data, ensuring the robustness and reliability of their research findings. This section serves as a compass, guiding students through the complexities of handling missing data and fostering a skill set essential for data-driven academic success.
Identification of Missing Data
Missing data holds the potential to significantly undermine the robustness of statistical analyses, emphasizing the need for meticulous data management. Within the SPSS toolkit, an invaluable ally emerges in the form of tools dedicated to identifying missing values. Students are encouraged to acquaint themselves with these tools, with the "Descriptives" option under the "Analyze" menu standing out. This feature furnishes detailed statistics on missing values for each variable, offering a comprehensive overview that serves as the foundation for informed decision-making in subsequent steps.
Imputation Techniques
Upon successful identification of missing data, students embark on the critical task of selecting an appropriate imputation strategy. SPSS extends a repertoire of imputation techniques, encompassing mean imputation, regression imputation, and the more sophisticated multiple imputation. Delving into the nuances of each method is paramount, as it empowers students to make judicious choices aligned with the specificities of their dataset. This understanding not only safeguards the integrity of the data but also positions students to navigate the intricacies of imputation with confidence and precision, ensuring the reliability of their subsequent analyses.
Data Screening and Validation
Data screening serves as a pivotal phase in the data management process, acting as a gatekeeper for reliable analyses. This section delves into outlier detection and essential validation checks, equipping students with tools to fortify the reliability and consistency of their datasets before embarking on detailed analyses.
1. Outlier Detection
The identification and management of outliers are paramount in maintaining the integrity of statistical analyses. SPSS, a versatile analytical tool, provides both graphical and numerical tools for outlier detection, including boxplots and Z-scores. Students should conscientiously interpret these results, weighing the impact of outliers on their analyses. This step allows students to make informed decisions, whether through the exclusion or transformation of outlier values, ensuring the robustness of subsequent statistical procedures.
2. Data Validation Checks
A cornerstone of data integrity, validation checks are indispensable for accuracy and consistency. SPSS facilitates a range of checks, encompassing validation for ranges, consistency, and frequencies. Students must employ these tools systematically, scrutinizing their datasets for anomalies. Rectifying any identified discrepancies at this stage fortifies the dataset, laying a solid foundation for trustworthy and meaningful analyses in the subsequent phases of their academic pursuits.
Advanced Data Management Techniques
Advanced Data Management Techniques serve as a crucial gateway for students aiming to enhance their proficiency in SPSS. This section, specifically concentrating on merging datasets and aggregating data, provides students with indispensable skills for navigating the intricacies of diverse datasets. Merging datasets is a skill that enables students to combine information from multiple sources seamlessly, enriching their analyses with comprehensive insights. Understanding various merge types, such as inner, outer, left, and right joins, empowers students to make informed decisions during the merging process.
Similarly, the ability to aggregate data is paramount for summarizing information at a higher level, facilitating a more in-depth understanding of the dataset. Utilizing the "Data" menu's "Aggregate" function, students can calculate means, totals, or other summary statistics for specific groups. These advanced techniques not only broaden students' data management toolkit but also pave the way for a more nuanced and sophisticated approach to data analysis, ultimately contributing to a holistic understanding of statistical methods and their practical applications.
Merging and Aggregating Data
Merging datasets and aggregating data are indispensable skills in the analytical toolkit, elevating the quality and depth of statistical analyses. This section serves as a guide, shedding light on the intricate process of merging information from diverse sources seamlessly and summarizing data effectively. By grasping these techniques, students acquire the prowess to tackle intricate analytical scenarios with confidence. The ability to combine and distill information from multiple datasets not only broadens their analytical scope but also equips them to derive meaningful insights from complex data structures. As students embrace these advanced techniques, they pave the way for a more nuanced and sophisticated approach to data management, setting the stage for robust and insightful analyses.
Merging Datasets
In real-world scenarios, students often encounter the need to merge datasets to enrich their analyses. SPSS facilitates dataset merging through the "Data" menu, allowing students to combine information from multiple sources. It is crucial to understand the different types of merges, such as inner, outer, left, and right joins, to make informed decisions during the merging process. The choice of the merging strategy depends on the specific research question and the nature of the datasets involved. Inner joins retain only the common elements, while outer joins preserve all records, even those without matches. Left and right joins prioritize the data from one specified dataset. This nuanced understanding empowers students to tailor their merging approach to extract the most relevant insights from diverse datasets.
Aggregating Data
Aggregating data involves summarizing information at a higher level, such as calculating means or totals for specific groups. SPSS provides the "Data" menu's "Aggregate" function for this purpose. Students should carefully choose aggregation variables and functions to obtain meaningful summary statistics for their analyses. This process is particularly valuable when dealing with large datasets or when aiming to derive overarching trends. The aggregation step transforms raw data into concise, interpretable summaries, providing a clearer picture for subsequent analyses. Selecting appropriate aggregation methods ensures that the summarized data accurately represents the characteristics of interest, laying the foundation for more robust and insightful research outcomes.
Automation through Syntax
Syntax automation stands as a crucial proficiency, significantly enhancing efficiency within the SPSS environment. In this section, students will be guided through the nuanced process of not only writing and executing syntax but also the pivotal aspect of creating and leveraging syntax templates. This multifaceted approach ensures a more streamlined and reproducible data management process.
By mastering syntax, students gain the capability to automate routine tasks, providing a substantial boost to their analytical workflows. The ability to write and execute syntax becomes a potent skill, enabling users to effortlessly replicate analyses and maintain consistency across different projects. Furthermore, the emphasis on creating syntax templates offers a strategic advantage, allowing students to build a library of reusable scripts tailored to specific assignments. This holistic understanding of syntax automation equips students with a versatile toolset, transforming their SPSS experience into a dynamic and efficient venture.
Achieving proficiency in syntax is not merely a technical skill but a strategic asset, empowering students to navigate the complexities of data management with confidence and precision.
Writing and Executing Syntax
As students progress in their SPSS journey, embracing syntax becomes increasingly valuable. Syntax allows for the automation of tasks, making the data management process more efficient and reproducible. Writing and executing syntax in SPSS is a skill that students should cultivate, as it empowers them to replicate analyses consistently and share their workflows with others.
The ability to craft syntax scripts in SPSS brings a transformative dimension to data analysis. By leveraging syntax, students gain the capability to create a detailed, step-by-step set of instructions that not only conducts analyses but also documents the entire process. This documentation proves invaluable for later reference, ensuring transparency and facilitating collaboration.
Creating and Using Syntax Templates
To further streamline their workflow, students can create syntax templates for common data management tasks. These templates serve as reusable scripts that can be modified for specific assignments. Building a library of syntax templates not only enhances efficiency but also promotes a systematic approach to data management.
Incorporating syntax templates into their repertoire enables students to establish a standardized methodology. This not only saves time but also enhances the replicability of their analyses. The ability to adapt and customize these templates for different assignments showcases a sophisticated level of proficiency in SPSS, setting students on a trajectory towards becoming adept data analysts.
Conclusion
In conclusion, the mastery of data management in SPSS stands as a fundamental and transformative skill for students navigating the intricate realm of statistical analysis. Moving beyond the rudiments of data entry, students must embrace advanced techniques such as syntax automation to truly harness the power of SPSS. A holistic comprehension of SPSS not only instills confidence in approaching assignments but also cultivates a nuanced understanding of the data-driven landscape.
By diligently implementing the best practices elucidated in this comprehensive blog post, students fortify their data management proficiency. This not only ensures the precision and dependability of their analyses but also lays the groundwork for scholarly excellence. As students embark on their academic journey, the acquisition of SPSS expertise emerges as a valuable asset, serving as a gateway to a profound and nuanced comprehension of the intricate dynamics that govern the data-driven world they are set to explore.