Claim Your Offer
Unlock an exclusive deal at www.statisticsassignmenthelp.com with our Spring Semester Offer! Get 10% off on all statistics assignments and enjoy expert assistance at an affordable price. Our skilled team is here to provide top-quality solutions, ensuring you excel in your statistics assignments without breaking the bank. Use Offer Code: SPRINGSAH10 at checkout and grab this limited-time discount. Don’t miss the chance to save while securing the best help for your statistics assignments. Order now and make this semester a success!
We Accept
- Understanding Regression Analysis
- Least-Squares Estimates
- Testing the Slope
- Confidence Interval for the Slope
- Confidence Bands
- Applying Regression Analysis to Problems
- Problem 1: Campaign Expenditures and Voter Turnout
- Problem 2: Sales Revenue and Advertising Expenditure
- Problem 3: Educational Resources and Student Performance
- Problem 4: Predicting Pathology Levels in Psychotic Patients
- Problem 5: Relationship Between Reading Hours and Various Factors
- General Tips for Solving Statistical Assignments
- Conclusion
When tackling statistical assignments involving regression analysis, hypothesis testing, and confidence intervals, a thorough understanding of these fundamental concepts and methodologies is crucial. Whether you're analyzing relationships between variables or testing hypotheses, having a solid grasp of regression techniques and statistical inference methods will enable you to handle complex problems effectively. If you're seeking assistance with regression analysis assignment, it's important to follow a structured approach to ensure accurate and meaningful results. This guide is designed to help you navigate the intricacies of these statistical techniques, offering a clear framework for solving various types of problems. From understanding how to compute least-squares estimates to interpreting confidence intervals and performing hypothesis tests, this comprehensive overview will equip you with the skills needed to excel in your statistical assignment.
Understanding Regression Analysis
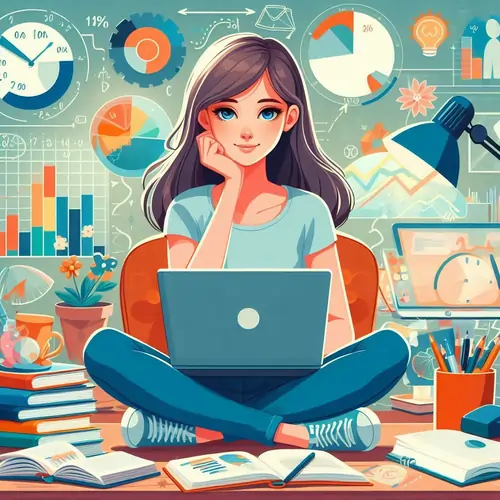
Regression analysis is a powerful statistical tool used to explore and quantify the relationship between a dependent variable (Y) and one or more independent variables (X). The goal of regression analysis is to determine how the dependent variable changes in response to variations in the independent variables. By fitting a linear equation to the data, regression analysis helps in estimating the coefficients that define the relationship between these variables. This linear equation provides a model that predicts the dependent variable based on the values of the independent variables. In essence, regression analysis allows us to understand and measure the strength and direction of the association between variables, making it a crucial method for forecasting, trend analysis, and decision-making in various fields. The results from regression analysis are used to draw insights, make predictions, and guide strategic planning based on statistical evidence.
Least-Squares Estimates
The least-squares method minimizes the sum of the squared differences between the observed values and the values predicted by the model. Here are the steps to determine the least-squares estimates:
- Data Collection: Collect the data for the dependent and independent variables.
- Mean Calculation: Calculate the means of the independent and dependent variables.
- Slope and Intercept Calculation: Compute the slope and intercept using appropriate statistical formulas.
- Plotting: Plot the data points and draw the regression line using the calculated slope and intercept.
This approach helps in creating a visual representation of the relationship between the variables, providing insights into how well the model fits the data.
Testing the Slope
To test the null hypothesis that the true slope is zero, use a t-test. This test helps determine whether there is a significant relationship between the independent and dependent variables. If the p-value obtained from the test is less than the significance level (usually 0.05), you can reject the null hypothesis, indicating a significant relationship.
Confidence Interval for the Slope
A 95% confidence interval for the slope provides a range of values within which the true slope is likely to fall. If the interval does not include zero, it suggests a significant relationship between the variables. This interval gives a measure of the reliability of the estimated slope.
Confidence Bands
Confidence bands around the regression line provide a visual representation of the uncertainty in the predicted values. To sketch confidence bands:
- Standard Error Calculation: Calculate the standard error of the estimate.
- Confidence Interval Determination: Use the standard error to determine the confidence interval for each predicted value.
Confidence bands help in understanding the range within which the true values are expected to lie, providing a clearer picture of the model's accuracy.
Applying Regression Analysis to Problems
Let's apply these concepts to different types of problems to understand their practical implementation:
Problem 1: Campaign Expenditures and Voter Turnout
1. Determine the Least-Squares Estimates:
- Calculate the slope and intercept for the relationship between TVEXP (percentage of total campaign expenditures delegated to television advertisements) and VOTE (percentage of registered voter turnout).
- Draw the regression line on a scatter diagram.
2. Test the Null Hypothesis:
- Perform a t-test to determine if campaign expenditures significantly influence voter turnout.
3. Confidence Interval for the Slope:
- Calculate the 95% confidence interval for the slope and interpret its significance.
4. Confidence Bands and Mean Estimation:
- Sketch 95% confidence bands and estimate the mean voter turnout for a given TVEXP value. This helps in understanding the expected voter turnout for a specified campaign expenditure on television advertisements.
Problem 2: Sales Revenue and Advertising Expenditure
1. Examine Linear Relationship:
- Use a scatter diagram to determine if there is a linear relationship between sales revenue and advertising expenditure.
2. Regression Model:
- State the model for the straight-line regression of sales revenue on advertising expenditure. Calculate the least-squares estimates for the regression line and interpret the estimated values of the slope and intercept in the context of the problem.
3. Significance of Slope:
- Test the significance of the slope parameter to see if advertising expenditure significantly affects sales revenue.
4. Confidence Interval for the Slope:
- Determine and interpret the 95% confidence interval for the slope, providing insights into the reliability of the relationship between sales revenue and advertising expenditure.
Problem 3: Educational Resources and Student Performance
1. ANOVA Table:
- Construct the ANOVA table for the regression model involving multiple independent variables (e.g., per pupil expenditure, percentage of teachers with a master's degree or higher, and pupil-teacher ratio).
2. R-Squared Value:
- Calculate and interpret the R-squared value to assess the model's fit. A higher R-squared value indicates a better fit, suggesting that the educational resource variables are significantly associated with student performance.
Problem 4: Predicting Pathology Levels in Psychotic Patients
1. Overall Regression F Tests:
- Conduct F tests to evaluate the overall fit of different regression models. This helps in determining the significance of the variables in predicting pathology levels.
2. Variables-Added-In-Order Tests:
- Perform tests to determine the importance of each independent variable in predicting the dependent variable. This involves adding variables in a specific order and evaluating their impact on the model.
3. Appropriate Model Selection:
- Based on the results of the tests, select the most appropriate regression model for predicting pathology levels. This ensures that the model includes the most significant variables, providing accurate predictions.
Problem 5: Relationship Between Reading Hours and Various Factors
1. Model Comparison Tests:
- Compare different regression models using F tests to evaluate the significance of each independent variable (e.g., social class, number of years of school completed, and reading speed).
2. Interpreting Results:
- Use the test results to draw conclusions about the relationship between the number of hours devoted to reading and the independent variables. This helps in understanding the factors that significantly influence reading habits.
General Tips for Solving Statistical Assignments
- Understand the Problem: Carefully read the problem statement to understand what is being asked. Identify the dependent and independent variables and the type of analysis required.
- Collect and Prepare Data: Gather the necessary data and prepare it for analysis. Ensure that the data is clean and free of errors.
- Choose the Right Method: Select the appropriate statistical method based on the problem. This could involve regression analysis, hypothesis testing, or confidence interval estimation.
- Perform Calculations: Use statistical software or manual calculations to perform the necessary analysis. Ensure that you follow the correct steps and use accurate formulas.
- Interpret Results: Carefully interpret the results of your analysis. Consider the significance of the findings and their implications for the problem at hand.
- Present Findings: Clearly present your findings, including any graphs, tables, or charts that help illustrate the results. Provide a detailed explanation of the results and their significance.
By following these steps and understanding the underlying concepts, you can effectively solve statistical assignments involving regression analysis, hypothesis testing, and confidence intervals. This structured approach will help you tackle a wide range of problems in your statistics coursework, providing you with the skills and confidence to excel in your studies.
Conclusion
In conclusion, effectively solving statistical assignments requires a solid grasp of key concepts such as regression analysis, hypothesis testing, and confidence intervals. By following a structured approach—starting with data collection, applying appropriate statistical methods, and accurately interpreting results—students can navigate complex problems with confidence. Understanding how to calculate least-squares estimates, test hypotheses, and construct confidence intervals is crucial for drawing meaningful conclusions from data. Applying these techniques to practical problems, such as analyzing campaign expenditures or sales revenue, further enhances your analytical skills. With a clear framework and careful execution, students can tackle a wide range of statistical assignments, ensuring robust and insightful analyses. Mastering these methods not only aids in academic success but also equips you with valuable skills applicable in real-world scenarios.