Claim Your Offer
Unlock an exclusive deal at www.statisticsassignmenthelp.com with our Spring Semester Offer! Get 10% off on all statistics assignments and enjoy expert assistance at an affordable price. Our skilled team is here to provide top-quality solutions, ensuring you excel in your statistics assignments without breaking the bank. Use Offer Code: SPRINGSAH10 at checkout and grab this limited-time discount. Don’t miss the chance to save while securing the best help for your statistics assignments. Order now and make this semester a success!
We Accept
- Reasons for Data Transformation
- Normality Assumption
- Homoscedasticity
- Linearity
- Removing Skewness and Outliers
- Common Data Transformation Techniques
- Log Transformation
- Square Root Transformation
- Reciprocal Transformation
- Box-Cox Transformation
- Winsorization
- Applying Data Transformation in SPSS
- Descriptive Statistics
- Data Screening
- Data Transformation Procedure
- Conclusion
Reasons for Data Transformation
Data transformation is employed for various reasons to ensure the validity and reliability of statistical analyses. Some common reasons include:
Normality Assumption
Many parametric statistical tests assume that the data follows a normal distribution. However, real-world datasets often deviate from normality. By transforming the data, we can bring it closer to a normal distribution, which ensures the accuracy of parametric tests such as t-tests, ANOVA, and regression.
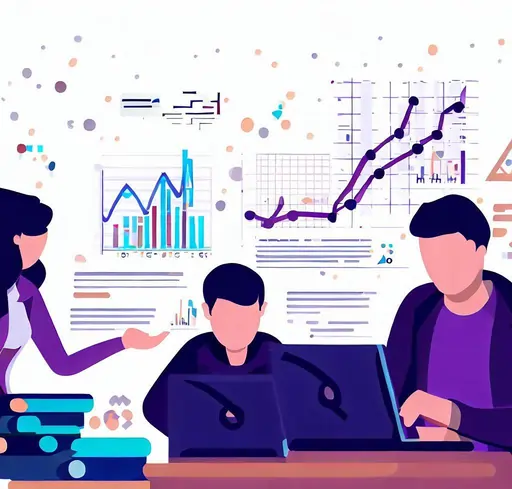
Homoscedasticity
Homoscedasticity implies that the variance of the dependent variable is constant across all levels of the independent variable. When this assumption is violated, the results of regression analyses may be biased. Data transformation can help stabilize the variance, leading to more accurate regression results.
Linearity
Linear regression assumes a linear relationship between the dependent and independent variables. If this assumption is not met, data transformation can help establish a linear relationship and improve the regression model's performance.
Removing Skewness and Outliers
Skewed data and outliers can significantly influence statistical analyses and distort results. Transformation methods such as log transformation or Winsorization can reduce the impact of extreme values and make the data more suitable for analysis.
Common Data Transformation Techniques
Data transformation techniques like log transformation, square root transformation, and Box-Cox transformation are essential for stabilizing variance, reducing skewness, and normalizing data. These methods play a vital role in preparing data for accurate statistical analyses in SPSS assignments.
Log Transformation
Log transformation is a powerful data manipulation technique widely used in SPSS assignments. By taking the logarithm of data values, it compresses large values while expanding small ones, effectively reducing the impact of extreme values and improving the symmetry of the distribution. This transformation is particularly valuable when dealing with positively skewed data or when the variance increases with the mean. Log transformation helps stabilize variance, make data more approximately normal, and ensures the validity of parametric tests like t-tests and ANOVA. It is a valuable tool for researchers seeking accurate and reliable results when analyzing real-world datasets in SPSS.
Square Root Transformation
The square root transformation is a valuable data conversion method used in SPSS assignments to address moderate right-skewed data. By taking the square root of each data point, this technique compresses higher values while expanding smaller ones, effectively reducing the impact of extreme values. This transformation is particularly useful when the data exhibits a nonlinear relationship, making it closer to a normal distribution and meeting the assumptions of parametric tests. However, it is essential to note that the square root transformation might not be suitable for highly skewed data. As part of a comprehensive data transformation approach, understanding when to apply the square root transformation can significantly enhance the accuracy of statistical analyses.
Reciprocal Transformation
The reciprocal transformation, involving the computation of the reciprocal (1/x) of data points, is a valuable technique in data preparation for SPSS assignments. Particularly useful when dealing with negatively skewed data, this transformation enhances symmetrical distribution properties. By reciprocating the values, extreme data points are effectively compressed, reducing their influence on statistical analyses. The resulting transformed data offers improved compatibility with assumptions of certain tests, promoting accurate inferential outcomes. When encountering non-linear relationships, the reciprocal transformation can aid in establishing a linear relationship between variables, contributing to the efficacy of regression models. Proper application of this method in SPSS empowers analysts to derive meaningful insights from complex datasets.
Box-Cox Transformation
Box-Cox transformation is a powerful data conversion technique used to address skewed data and heteroscedasticity. It offers a family of power transformations that cater to various data distributions. By identifying the optimal λ value, the data can be normalized, making it more suitable for parametric statistical tests. In SPSS, researchers can either let the software automatically determine the λ value or manually specify it. The versatility of Box-Cox transformation makes it an invaluable tool in handling complex datasets, ensuring statistical assumptions are met, and ultimately leading to more reliable and accurate results in SPSS assignments.
Winsorization
Winsorization is a robust data transformation technique used to address the influence of outliers in statistical analyses. By capping extreme values, Winsorization effectively reduces the impact of outliers without entirely removing them from the dataset. This method ensures that the overall distribution of the data remains intact while mitigating the potential distortion caused by extreme values. In SPSS assignments, Winsorization offers a practical solution to handle outliers, enabling researchers to obtain more accurate results and make reliable inferences. Its flexibility allows for customized percentile thresholds, tailoring the transformation to suit specific dataset characteristics and analysis requirements.
Applying Data Transformation in SPSS
Applying data transformation in SPSS involves calculating appropriate functions like log, square root, or Box-Cox for variables to meet statistical assumptions. This process enhances the validity of analyses and facilitates more accurate interpretations of results.
Descriptive Statistics
Descriptive statistics play a fundamental role in data exploration and preparation within SPSS assignments. By calculating measures such as mean, standard deviation, skewness, and kurtosis, researchers gain valuable insights into the distribution and characteristics of the variables. Understanding the data's central tendency, variability, and shape allows researchers to identify potential issues, such as skewness or extreme values, which may require data transformation. Descriptive statistics also aid in making informed decisions regarding the choice of appropriate data transformation techniques. Overall, the analysis of descriptive statistics empowers researchers to effectively cleanse and transform the data, paving the way for accurate and reliable statistical analyses in SPSS.
Data Screening
Data screening is a crucial preliminary step in the data transformation process within SPSS. It involves identifying and managing missing data, outliers, and extreme values. By addressing these data issues early on, researchers can ensure data integrity and prevent potential biases in subsequent analyses. SPSS provides powerful tools for data screening, including imputation techniques for missing data and identification of outliers through graphical representations and statistical tests. Proper data screening allows researchers to make informed decisions on whether to remove or transform outliers, improving the overall quality of the dataset and the reliability of the results obtained from subsequent data transformation and analysis.
Data Transformation Procedure
The data transformation procedure in SPSS is a systematic process to prepare data for analysis. After importing the dataset, researchers can access the "Transform" menu and choose "Compute Variable." Here, they can specify the new variable's name and select the appropriate transformation function, such as log or square root. The transformation is then applied to the desired variable, creating a new transformed variable. Researchers must assess the normality and compare it to the original variable. This step-by-step process empowers users to handle skewed, heteroscedastic, or non-normally distributed data effectively, ensuring the accuracy and reliability of their statistical analyses in SPSS.
Conclusion
Data transformation plays a crucial role in ensuring the accuracy and reliability of statistical analyses in SPSS assignments. By addressing issues such as non-normality, heteroscedasticity, and outliers, data transformation enhances the validity of results and facilitates meaningful interpretations. This guide has provided an overview of data transformation techniques and their application in SPSS, as well as assignment help tips to ensure a successful analysis. Mastering data transformation will empower you to handle complex datasets effectively and make informed decisions in your research and analyses using SPSS.
You Might Also Like
Explore a wealth of knowledge and insights through our engaging blogs. From expert tips and industry trends to practical advice and innovative solutions, our blog is your go-to resource for staying informed and inspired. Dive into our latest posts and unlock new perspectives on topics that matter to you.