Mastering Advanced Modeling Techniques in SPSS for University Assignments

- Factor Analysis for Dimension Reduction
- Data Preparation: Start by importing your dataset into SPSS. Ensure that your variables are continuous and have no missing values.
- Choosing the Factors: Decide on the number of factors you want to extract. This can be determined through methods like the Kaiser-Guttman rule, scree plot analysis, or theoretical reasoning.
- Factor Extraction: Use one of the common methods for factor extraction, such as Principal Component Analysis (PCA) or Maximum Likelihood. SPSS provides easy-to-use options for these methods.
- Factor Rotation: Rotate the factors to achieve a more interpretable and meaningful solution. Popular rotation methods include Varimax and Promax.
- Interpretation: Interpret the factors based on the loadings of variables on each factor. This step is crucial for understanding the underlying dimensions in your data.
- Reporting: In your assignment, present the results, including factor loadings and the interpretation of factors. Explain how factor analysis helped in dimension reduction and simplifying the data.
- Cluster Analysis for Grouping Similar Cases
- Data Preparation: Similar to factor analysis, begin by importing your dataset into SPSS and ensuring data cleanliness.
- Choosing the Number of Clusters: Decide on the number of clusters you want to create. You can use methods like the Elbow Method or Dendrogram to determine an appropriate number.
- Cluster Algorithm Selection: SPSS offers various clustering algorithms such as K-Means, Hierarchical, and Two-Step Cluster.
- Running the Analysis: Execute the cluster analysis, and SPSS will create clusters based on the variables you specified.
- Interpretation: Examine the characteristics of each cluster and understand what makes them similar. This step is crucial for drawing meaningful insights from your data.
- Reporting: In your assignment, report the number of clusters, their characteristics, and any significant findings. Discuss how cluster analysis aided in grouping similar cases in your research.
- Discriminant Analysis for Predicting Group Membership
- Data Preparation: Ensure your dataset is ready, with a categorical dependent variable (the group you want to predict) and one or more continuous independent variables.
- Selecting Variables: Choose the independent variables that you believe are relevant for predicting group membership.
- Running the Analysis: Execute the discriminant analysis in SPSS, specifying the dependent and independent variables.
- Interpretation: Examine the output, which will include discriminant functions and their coefficients. These coefficients indicate the importance of each independent variable in predicting group membership.
- Validation: Assess the accuracy of the discriminant model using techniques like cross-validation or split-sample validation.
- Reporting: In your assignment, report the results, including the discriminant functions and the variables that significantly contribute to group prediction. Discuss the implications of your findings in the context of your research question.
- Time Series Forecasting
- Data Preparation: Import your time series data into SPSS, ensuring that the time variable is correctly formatted.
- Exploratory Data Analysis: Conduct an initial exploration of your time series data to identify trends, seasonality, and potential outliers.
- Choosing the Forecasting Model: Select an appropriate forecasting model based on the characteristics of your data. Common models include ARIMA (AutoRegressive Integrated Moving Average) and Exponential Smoothing.
- Model Estimation: Use SPSS to estimate the parameters of your chosen model.
- Model Evaluation: Assess the accuracy of your forecasting model using techniques like Mean Absolute Error (MAE) or Mean Squared Error (MSE).
- Generating Forecasts: Use the estimated model to generate forecasts for future time periods.
- Reporting: In your assignment, present the results of your time series forecasting, including the accuracy of your predictions and any insights gained from the analysis. Discuss how this information can be applied in a real-world context.
- Understanding the Assignment Requirements
- What is the specific research question or problem you need to address?
- Which advanced modeling technique(s) are you expected to apply?
- What are the data sources and variables involved?
- Are there any constraints or guidelines for the analysis?
- Data Preparation and Cleaning
- Factor Analysis: Use this technique when you need to reduce the dimensionality of your data while retaining essential information.
- Cluster Analysis: Opt for cluster analysis when your goal is to group similar cases together based on specific characteristics.
- Discriminant Analysis: Employ discriminant analysis for classification tasks, predicting group membership based on independent variables.
- Time Series Forecasting: Utilize time series forecasting when working with temporal data and making predictions about future values.
- Exploratory Data Analysis (EDA)
- SPSS Tools and Functions
- Interpreting Results
- Factor Analysis: Interpret factor loadings and their implications.
- Cluster Analysis: Examine cluster profiles and identify characteristics of each group.
- Discriminant Analysis: Analyze discriminant functions and variable contributions.
- Time Series Forecasting: Evaluate forecast accuracy and trends in time series data.
- Reporting and Documentation
- Clear explanation of the research question or problem.
- Comprehensive presentation of the advanced modeling technique applied.
- Interpretation of results, including insights and implications.
- Discussion of limitations and potential areas for further research.
- Validation and Sensitivity Analysis
- Seeking Help and Resources
When it comes to tackling university assignments involving data analysis and statistics, mastering advanced modeling techniques is the key to success. In the realm of statistical analysis, IBM's Statistical Package for the Social Sciences (SPSS) stands out as a powerful tool that can empower students to excel in their coursework. In this comprehensive blog post, we will delve deep into four advanced modeling techniques in SPSS that are specifically designed to help you solve your SPSS assignment with confidence and precision. These techniques include Factor Analysis for dimension reduction, Cluster Analysis for grouping similar cases, Discriminant Analysis for predicting group membership, and Time Series Forecasting for making data-driven predictions. So, let's embark on this journey to equip you with the knowledge and skills needed to conquer even the most complex SPSS assignments.
Factor Analysis is a data reduction technique that helps in identifying underlying factors or dimensions in a dataset. It is particularly useful when dealing with a large number of variables, making it easier to interpret and work with the data. Students often encounter assignments where they need to reduce the number of variables while preserving as much relevant information as possible.
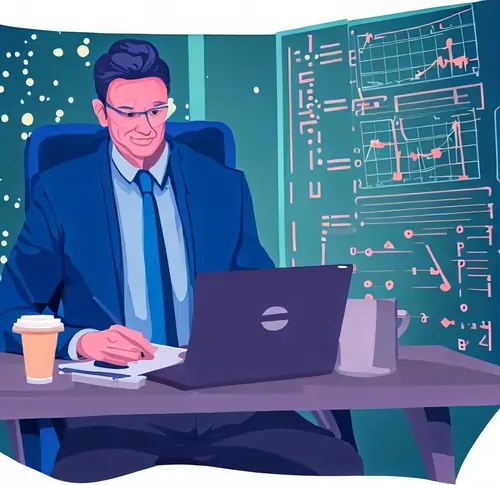
Key Steps in Factor Analysis
Cluster Analysis is a technique used to group similar cases together based on their characteristics or attributes. This method is often used in research and assignments to identify patterns and relationships within data.
Key Steps in Cluster Analysis
Discriminant Analysis is a statistical technique used to predict group membership based on a set of independent variables. This method is particularly valuable when dealing with classification problems and understanding what factors influence group assignments.
Key Steps in Discriminant Analysis
Time Series Forecasting is a critical skill in data analysis, especially when dealing with data that varies over time. This technique is used to make predictions or forecasts about future values based on historical data patterns.
Key Steps in Time Series Forecasting
How to Navigate SPSS Assignments Involving Advanced Modeling
Embarking on SPSS assignments that involve advanced modeling can be a challenging yet rewarding experience. To solve your SPSS assignment with proficiency, it's essential to navigate these tasks systematically and with a clear understanding of the underlying processes. In this section, we will guide you through the fundamental steps and strategies to effectively tackle assignments that require advanced modeling techniques in SPSS.
Before diving into any SPSS assignment, it's crucial to thoroughly comprehend the assignment's requirements and objectives. Consider the following:
Having a clear grasp of these details will serve as a solid foundation for your work.
Data Preparation and Cleaning is the cornerstone of any successful SPSS assignment. Ensuring your dataset is pristine and well-organized is paramount. By meticulously addressing missing values, outliers, and inconsistencies, you lay a solid foundation for meaningful analysis. SPSS offers intuitive tools to streamline this process, enabling you to focus on the core of your research. Clean data not only enhances the accuracy of your results but also simplifies the application of advanced modeling techniques, making your journey to solve your SPSS assignment smoother and more efficient.
3. Selecting the Appropriate Advanced Modeling Technique
Choose the advanced modeling technique that best aligns with your research question and dataset. Consider the following:
Exploratory Data Analysis (EDA) is an essential phase in any data analysis process. It involves delving into your dataset without preconceived notions, allowing patterns, anomalies, and relationships to emerge organically. By creating visualizations, such as scatter plots or histograms, and calculating summary statistics, EDA helps you gain a deep understanding of your data's structure. This understanding is pivotal for informed decision-making in subsequent analysis steps. EDA can unveil hidden insights, guide variable selection, and identify potential outliers or data quality issues, making it an indispensable tool in the data analyst's toolbox.
In the realm of advanced modeling within SPSS, mastering the specific tools and functions available is paramount. These tools streamline the process of applying techniques like Factor Analysis, Cluster Analysis, Discriminant Analysis, and Time Series Forecasting. SPSS provides an intuitive interface, allowing students to define variables, specify criteria, and generate insightful results effortlessly. Understanding these tools and functions ensures that your analysis is not only accurate but also efficient, saving valuable time while working on assignments that demand complex statistical modeling.
Once your analysis is complete, interpret the results in the context of your research question. Pay close attention to the output generated by SPSS, including tables, charts, and statistical values. For each advanced modeling technique, focus on understanding key outcomes:
A well-structured and comprehensive report is essential to solve your SPSS assignment effectively. Include the following:
Validation and sensitivity analysis play a crucial role in ensuring the robustness and reliability of your advanced modeling results. Validation techniques help confirm that your model's predictions or classifications hold up under different scenarios and are not the result of overfitting. Sensitivity analysis explores how variations in input data or model parameters impact the outcomes, providing insights into the model's stability. By addressing these aspects in your SPSS assignments, you demonstrate a commitment to producing accurate and trustworthy results, bolstering the credibility of your findings. These practices are essential in real-world applications, where the ability to validate and assess model sensitivity can lead to more informed decision-making.
When navigating the complexities of SPSS assignments involving advanced modeling, seeking help and utilizing available resources can significantly enhance your success. SPSS has a vibrant user community, and online platforms offer an array of tutorials and forums for assistance. Engaging in discussions and seeking guidance from experienced users can unravel intricacies and provide fresh insights. Additionally, your academic institution likely offers resources such as research mentors, professors, and specialized workshops. Embracing these resources fosters a collaborative learning environment, where you can exchange ideas, troubleshoot issues, and refine your skills, ultimately ensuring that you can confidently solve your SPSS assignment with expert proficiency.
Conclusion
Mastering advanced modeling techniques in SPSS is an indispensable skill for university students. Factor analysis, cluster analysis, discriminant analysis, and time series forecasting provide powerful tools to effectively solve your SPSS assignment. Understanding these methods, coupled with meticulous data preparation, comprehensive interpretation, and effective utilization of SPSS tools, equips students to tackle complex data analysis tasks with confidence. Moreover, leveraging online resources and seeking assistance when needed ensures a supportive learning environment. Armed with these skills and strategies, students are well-prepared to excel in their academic journey and beyond.