Applied Multilevel Modeling: Techniques for Hierarchical Data Analysis
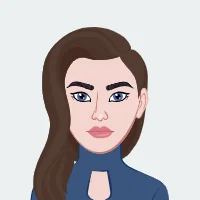
In the realm of statistics and data analysis, understanding how to handle hierarchical data is crucial for drawing meaningful insights. One powerful tool in this regard is Applied Multilevel Modeling. This blog aims to provide students with a comprehensive guide to the techniques involved in Hierarchical Data Analysis using Multilevel Modeling. Whether you are a novice or looking to deepen your knowledge, this exploration into multilevel modeling will equip you with the skills needed to tackle assignments effectively.
As we embark on this journey, it's essential to recognize the significance of hierarchical data. In many real-world scenarios, data is inherently structured in a hierarchical manner, with observations nested within larger groups. This could be seen in educational settings with students nested within classrooms, or in organizational contexts with employees grouped within different departments. The hierarchical nature of such data poses challenges to traditional statistical methods, and this is where Applied Multilevel Modeling comes to the rescue.
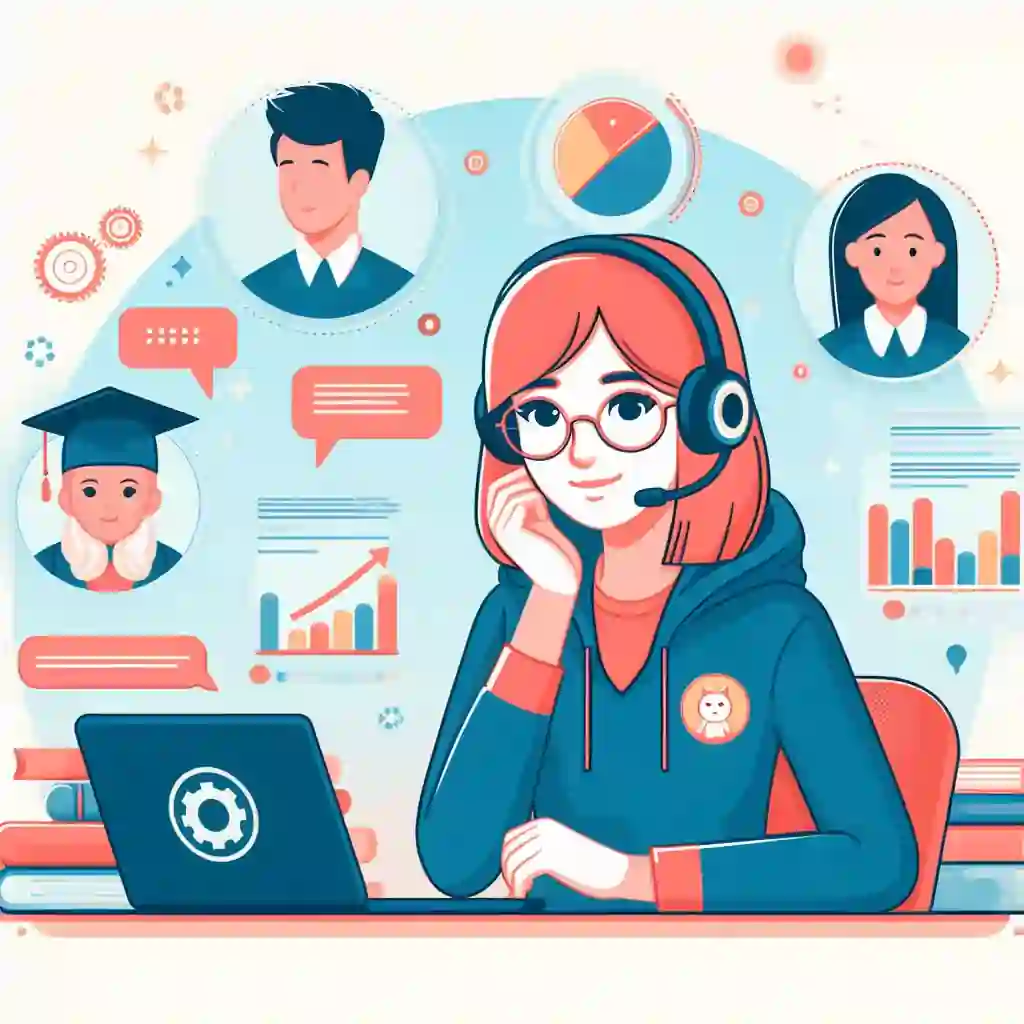
Multilevel Modeling, also known as hierarchical linear modeling or mixed-effects modeling, offers a nuanced approach to data analysis by considering variations both within and between groups. This technique acknowledges the interdependence of observations within the same group while allowing for the exploration of broader trends across groups. The result is a more accurate and insightful analysis that goes beyond the limitations of conventional statistical methods.
For students embarking on their journey into the world of multilevel modeling, acquiring a solid understanding of the foundational concepts is crucial, especially when seeking assistance with statistics assignment. At its core, multilevel modeling discerns between different levels of variation in the data. Some factors exhibit variation within groups, often referred to as random effects, and those that remain consistent across all groups, known as fixed effects. Grasping the intricate interplay between these effects is fundamental to constructing robust multilevel models.
Understanding the Basics of Multilevel Modeling
As we delve into the foundational aspects of multilevel modeling, it's imperative to grasp the conceptual underpinnings. Hierarchical data structures are prevalent in various fields, ranging from educational settings with students nested within classrooms to organizational contexts where employees are grouped within departments. Understanding the nuances of multilevel modeling becomes paramount as it allows researchers to account for the inherent complexities that arise from these hierarchical structures.
Concepts such as within-group and between-group variations form the bedrock of multilevel modeling. Within-group variations capture the variability at the individual level, acknowledging that observations within the same group are likely to be more similar than those across different groups. On the other hand, between-group variations recognize the differences at the group level, highlighting the uniqueness of each cluster within the dataset.
Conceptual Foundation
Before delving into the technical aspects, it's essential to grasp the conceptual foundation of multilevel modeling. At its core, multilevel modeling deals with data that has a hierarchical structure, where observations are nested within groups. This hierarchical arrangement could manifest in various contexts, such as students within schools, employees within companies, or any other organizational structure. The modeling technique considers both within-group and between-group variations, providing a nuanced perspective on data analysis that goes beyond conventional single-level models.
Moreover, the conceptual foundation extends to the acknowledgment of the interdependence among observations within the same group. Understanding this interdependence is crucial for capturing the intricacies of relationships within groups, offering a more holistic view of the data.
The Need for Multilevel Modeling
Hierarchical data poses challenges for traditional statistical methods, primarily because it violates the assumption of independence among observations. This violation arises due to the inherent structure of hierarchical data, where observations are naturally grouped. Conventional statistical methods often assume that data points are independent, leading to biased estimates when applied to hierarchical data.
Multilevel modeling addresses this challenge by recognizing and accommodating the inherent structure of the data. By acknowledging the interdependence among observations within groups, multilevel modeling provides a more accurate representation of the underlying dynamics. Whether it's accounting for variability at different levels or understanding the impact of group-level characteristics, multilevel modeling allows for a more accurate and realistic analysis that aligns with the complex nature of hierarchical data structures.
Key Components of Multilevel Modeling
Building upon the foundational understanding, this section extensively explores the fundamental components that constitute multilevel modeling, thereby enhancing your comprehension of this intricate statistical technique. Distinguishing between random effects and fixed effects is pivotal; random effects encapsulate variations unique to each group, while fixed effects represent constants across all groups. Proper model specification, another critical aspect, involves informed decisions on including variables as fixed or random effects, ensuring the model aligns with the data's hierarchical nature. Understanding the assumptions is equally crucial; thorough insights into these assumptions lay the groundwork for robust analyses. Throughout this section, students will gain profound insights into the indispensable role each component plays in constructing an effective multilevel model. Clear explanations accompanied by illustrative examples will empower students to make judicious decisions when confronted with hierarchical datasets, fostering a comprehensive grasp of the essential elements within the realm of multilevel modeling.
Random Effects and Fixed Effects
In the intricate landscape of multilevel modeling, the nuanced distinction between random effects and fixed effects plays a pivotal role in unraveling the intricacies of hierarchical data. Random effects, akin to statistical chameleons, deftly capture variations that are inherently unique to each group within the hierarchical structure. On the other hand, fixed effects stoically represent factors that stand unwaveringly constant across all groups, providing a baseline of stability against the dynamic backdrop of variability. Grasping the subtleties of these effects is not merely a technicality but a fundamental key to unlocking the true potential of multilevel modeling.
In the realm of random effects, it's crucial to recognize their role as the guardians of group-specific idiosyncrasies. These effects acknowledge and encapsulate the inherent diversity that might exist between different groups, whether it be students in different schools or employees in distinct departments. The beauty lies in their adaptability, dynamically adjusting to the unique characteristics of each group and thereby enriching the model with a depth that would be lost with a more rigid approach.
Fixed effects, in their constancy, provide a stable platform for comparison. Imagine them as the unchanging backdrop against which the random effects paint their variegated portraits. By identifying and incorporating factors that remain consistent across all groups, fixed effects enable a clearer understanding of the commonalities that transcend the intricacies of individual variation.
Understanding how to artfully weave these effects into your model is not a mere technicality but a strategic decision that shapes the narrative your data tells. The art lies in discerning which factors deserve the distinction of fixed effects, contributing to the overarching storyline, and which should be entrusted to the capricious yet revealing nature of random effects.
Model Specification and Assumptions
As we navigate the multifaceted world of multilevel modeling, the importance of proper model specification emerges as the bedrock of analytical success. Beyond the realm of equations and coefficients, it involves a thoughtful and informed decision-making process, akin to choosing the right ingredients for a culinary masterpiece. This decision-making extends to selecting variables that find their place as either fixed or random effects, shaping the very core of your analytical recipe.
The process of model specification demands a meticulous consideration of the assumptions that underpin the chosen model. These assumptions serve as the silent architects, laying the foundation upon which the entire analytical edifice stands. To overlook or dismiss these assumptions is akin to building a house on shaky ground – a structure destined for instability.
In the intricate dance of model specification, variables must be chosen with a discerning eye. Each variable introduced into the model should contribute meaningfully to the narrative, aligning with the research question at hand. A thoughtful exploration of the data's hierarchical structure is necessary, ensuring that the chosen variables resonate with the inherent levels within the dataset.
Equally critical is an awareness of the assumptions that accompany the chosen model. These assumptions, whether explicit or implicit, form the contract between the analyst and the statistical methods employed. Violating this contract can lead to biased estimates, distorting the very insights we seek to gain.
Practical Steps in Applied Multilevel Modeling
Moving from theory to practice, this section serves as a comprehensive guide, outlining the essential practical steps involved in applied multilevel modeling. The transition from theoretical understanding to hands-on application is pivotal for students seeking proficiency in hierarchical data analysis.
Data preparation and exploration take center stage in this practical journey. The emphasis is not only on recognizing hierarchical structures within the data but also on a meticulous examination of variable distributions. As students navigate this process, they gain valuable insights into the nuances of handling missing data, a common challenge in real-world datasets.
Data Preparation and Exploration
Before embarking on modeling, it's essential to prepare and explore your data thoroughly. Identify the hierarchical structure, examine the distribution of variables at different levels, and address any missing or outliers. A solid foundation in data preparation sets the stage for a robust multilevel analysis.
Choosing the Right Software
Several software options are available for conducting multilevel modeling, such as R, Python (with packages like Statsmodels or PyMC3), and specialized software like MLwiN. Choosing the right tool depends on your familiarity, preferences, and the specific requirements of your assignment. Familiarizing yourself with one or more of these tools is a valuable investment in your statistical skill set.
Advanced Techniques and Applications
As students progress in their multilevel modeling journey, this section introduces advanced techniques and their applications. Cross-level interactions, which delve into the variability of relationships across different groups, add depth to the analysis. Understanding how the relationship between variables evolves within distinct groups provides a nuanced perspective, enriching the interpretative capacity of the analysis. This not only enhances the comprehensiveness of the findings but also allows for targeted interventions based on specific group dynamics.
Furthermore, Multilevel Structural Equation Modeling (MSEM) is explored as a sophisticated approach, combining structural equation modeling with multilevel analysis. This integration enables a more intricate examination of latent variables and their relationships within hierarchical structures. Students are not only exposed to the theoretical underpinnings of MSEM but are also provided with practical insights on its implementation. This empowers them to navigate the complexities of real-world data scenarios with confidence, as MSEM offers a comprehensive framework for understanding and modeling intricate relationships at multiple levels.
As students delve into the intricacies of these advanced techniques, they expand their toolkit for tackling complex hierarchical data scenarios in both research and practical applications. The ability to apply cross-level interactions and MSEM equips them with a deeper understanding of the intricate dynamics present in hierarchical data. This expanded skill set not only enhances their analytical capabilities but also positions them as adept researchers capable of addressing the multifaceted challenges posed by hierarchical data structures.
Cross-Level Interactions
One of the distinctive strengths inherent in multilevel modeling lies in its unparalleled ability to delve into the intricate realm of cross-level interactions. This sophisticated analytical facet involves a meticulous examination of how the relationship between variables at one level dynamically evolves across diverse groups within the hierarchical structure. By mastering this intricate aspect of multilevel modeling, researchers and students unlock a trove of opportunities to reveal nuanced insights. The potential to tailor recommendations based on the specific characteristics of each group becomes not just a possibility but a powerful reality, enhancing the depth and applicability of hierarchical data analysis.
Multilevel Structural Equation Modeling (MSEM)
For those eager to broaden and fortify their multilevel modeling toolkit, delving into the advanced domain of Multilevel Structural Equation Modeling (MSEM) emerges as a logical and rewarding next step. MSEM seamlessly integrates the robust analytical capabilities of structural equation modeling with the nuanced intricacies of multilevel analysis. This synergy empowers researchers and students alike to conduct a more comprehensive examination of the complex relationships embedded within hierarchical data structures. The fusion of these two analytical approaches adds a layer of sophistication to the modeling process, allowing for a deeper understanding of the intricate interplay of variables and contributing to a more holistic interpretation of hierarchical datasets. As you embark on the journey of mastering MSEM, you open doors to a realm where the depth of analysis matches the complexity of the data you aim to decipher.
Conclusion
In conclusion, mastering Applied Multilevel Modeling emerges as an indispensable skill for students navigating the intricate landscape of hierarchical data analysis. This blog, serving as an insightful guide, not only elucidates foundational concepts but also delves into the application of advanced techniques, furnishing students with a robust roadmap to approach assignments with unwavering confidence. As you embark on your journey into the realm of multilevel modeling, it is imperative to underscore the pivotal role of practice. Consistent application of these techniques to real-world scenarios not only reinforces your comprehension but also furnishes you with a potent tool for adeptly unraveling the multifaceted intricacies inherent in hierarchical data structures. Happy modeling, and may your statistical endeavors be both rewarding and enlightening!