Mastering Biostatistics Assignment Topics with Effective Problem-Solving Strategies
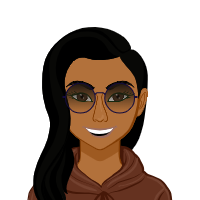
If you're facing a biostatistics assignment, understanding essential topics is vital. Mastering probability, hypothesis testing, study design, descriptive statistics, regression analysis, and survival analysis will empower you to approach your biostatistics assignment with confidence. Carefully organizing data, applying appropriate statistical tests, and interpreting results accurately are key aspects. By following these problem-solving strategies, you'll be better equipped to tackle the complexities of biostatistics assignments and produce meaningful insights for medical and biological studies, potentially seeking assistance with your biostatistics assignment if needed.
Essential Topics for Biostatistics Assignments
Biostatistics assignments demand a solid grasp of crucial topics. Probability and distributions lay the foundation, while hypothesis testing enables meaningful conclusions. Understanding study design and sampling methods helps analyze data accurately. Descriptive statistics summarize data effectively, and regression analysis models relationships between variables. Survival analysis tackles time-to-event data, vital for medical and biological studies.
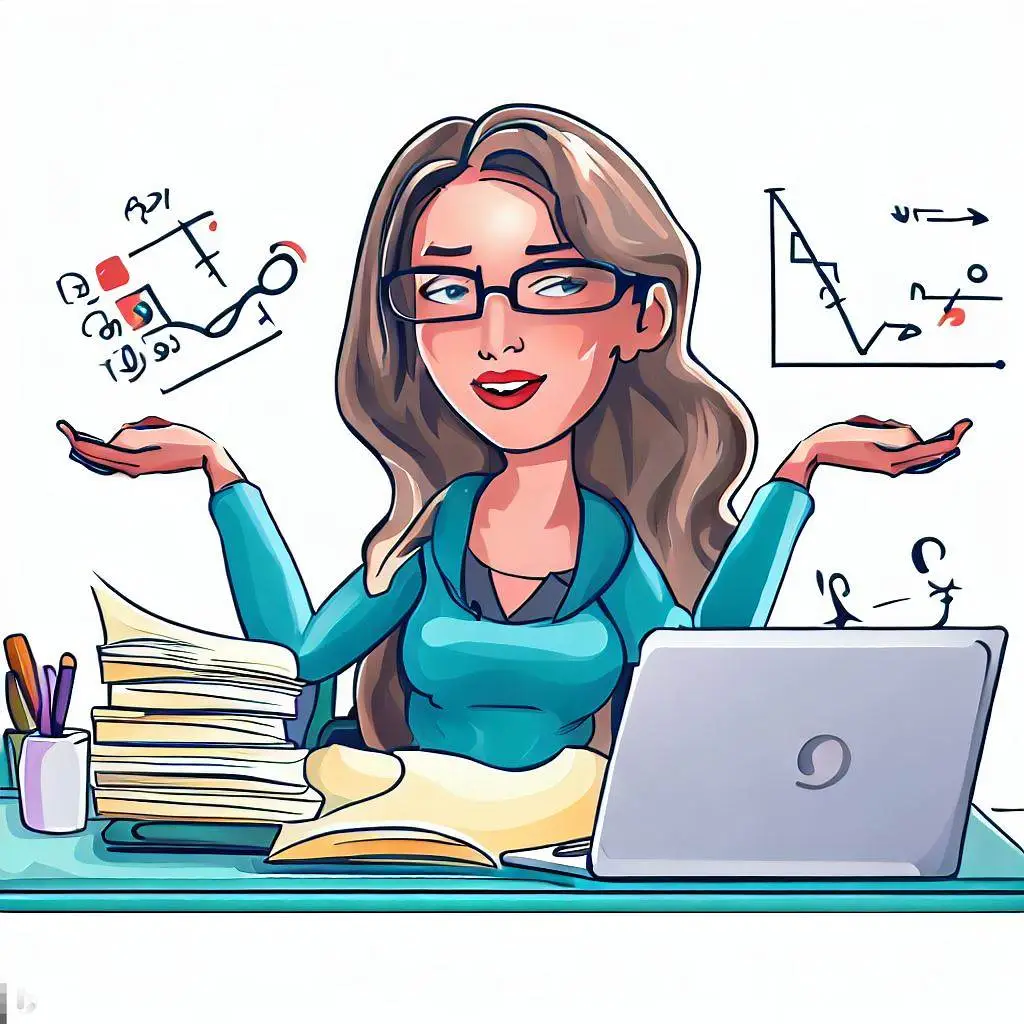
1. Probability and Distributions
Probability and distributions form the bedrock of biostatistics, allowing researchers to quantify uncertainty and make informed decisions based on data. Probability measures the likelihood of events occurring, while distributions describe how data is spread across different values.
In biostatistics assignments, understanding probability enables the assessment of the likelihood of specific outcomes in biological experiments or medical studies. It aids in predicting disease occurrences, treatment responses, and potential risks in patient populations.
Probability distributions, such as the normal distribution, are frequently encountered in biostatistical analyses. The normal distribution is particularly useful for approximating various biological measurements like height, weight, and blood pressure. Other distributions, such as the binomial distribution (useful for binary outcomes) and the Poisson distribution (useful for count data), are essential in analyzing discrete data commonly found in health-related research.
Having a firm grasp of probability and distributions enables the selection of appropriate statistical tests and modeling techniques, providing valuable insights into biological phenomena. It empowers researchers to make evidence-based decisions, ultimately contributing to advancements in medical science and public health.
2. Hypothesis Testing
Hypothesis testing involves formulating two opposing hypotheses: the null hypothesis (H0) and the alternative hypothesis (H1). The null hypothesis assumes no significant difference or effect, while the alternative hypothesis proposes a specific effect or relationship.
In biostatistics assignments, hypothesis testing is crucial for evaluating the effectiveness of new treatments, assessing the impact of interventions, and drawing conclusions from experimental data. By calculating p-values and comparing them to a pre-defined significance level (often 0.05), researchers can determine if the results are statistically significant.
Understanding hypothesis testing helps researchers avoid drawing erroneous conclusions from their data. They can confidently determine whether observed differences or associations are likely due to chance or represent true effects. Furthermore, researchers can adjust the significance level to control for Type I and Type II errors, ensuring the reliability of their findings. Through hypothesis testing, biostatisticians contribute to evidence-based decision-making in medical research and healthcare practices.
3. Study Design and Sampling Techniques
Study design and sampling techniques are critical aspects of biostatistics that influence the quality and validity of research findings. A well-designed study ensures that data collected is representative of the target population and minimizes biases.
In biostatistics assignments, understanding study design is essential for assessing the appropriateness of the data and drawing accurate conclusions. Different study designs, such as cross-sectional, cohort, and case-control studies, have distinct advantages and limitations. Researchers must consider factors like time, cost, and ethical considerations when selecting the most suitable design for their research question.
Sampling techniques are equally important, as they impact the generalizability of results. Random sampling, stratified sampling, and cluster sampling are commonly used methods. Biostatisticians need to be mindful of potential sampling biases and account for them in their analyses.
A well-designed study with appropriate sampling techniques enhances the credibility of research findings, contributing to evidence-based healthcare practices. In biostatistics assignments, students should critically evaluate study designs and sampling methods to ensure accurate interpretation and meaningful contributions to the field of medical research.
4. Descriptive Statistics
Descriptive statistics play a pivotal role in biostatistics assignments by providing a concise summary and visual representation of data. They enable researchers to grasp essential features of a dataset, identify patterns, and draw preliminary insights before conducting further analyses.
Measures of central tendency, such as the mean, median, and mode, help researchers understand the typical value or the center of the data. For instance, calculating the mean height of a group of patients in a clinical trial provides valuable information about their average stature.
Measures of variability, including the standard deviation and range, quantify the spread or dispersion of the data points. This information is critical for assessing the consistency and reliability of the measurements. For instance, a small standard deviation in drug trial results indicates that patients' responses are relatively consistent.
Graphical representations, like histograms, box plots, and scatter plots, enhance the understanding of data distributions and relationships between variables. Descriptive statistics offer researchers an initial glimpse into the data's characteristics, facilitating the decision-making process regarding suitable statistical methods for analysis. By effectively employing descriptive statistics, researchers can present clear and meaningful findings in their biostatistics assignments, contributing to the advancement of medical knowledge and patient care.
5. Regression Analysis
Regression analysis is a powerful statistical tool widely used in biostatistics to explore and model relationships between variables. It allows researchers to understand how one or more independent variables influence a dependent variable. In biostatistics assignments, regression analysis plays a critical role in identifying and quantifying associations between key factors and health outcomes.
Linear regression is frequently employed when dealing with continuous dependent variables, enabling researchers to predict outcomes based on the values of independent variables. Logistic regression, on the other hand, is used for binary or categorical dependent variables, often utilized to analyze the likelihood of disease occurrence or treatment success.
By applying regression analysis in biostatistics, researchers can identify risk factors, assess the impact of interventions, and make predictions about future health trends. Additionally, regression models provide valuable insights into the strength and direction of relationships, enabling evidence-based decision-making in healthcare and medical research. Understanding regression analysis equips biostatisticians with a powerful toolset to contribute to advancements in public health and medicine.
6. Survival Analysis
Survival analysis is a specialized statistical technique used in biostatistics to analyze time-to-event data, commonly observed in medical and biological studies. The "event" in this context can be anything of interest, such as the onset of a disease, patient recovery, or time until relapse.
In biostatistics assignments, survival analysis is indispensable for assessing the effectiveness of medical treatments and interventions, as it allows researchers to study the time it takes for specific outcomes to occur. The Kaplan-Meier curve is a vital tool in survival analysis, visualizing the probability of an event happening over time. Additionally, the Cox proportional hazards model is commonly employed to analyze the effects of multiple factors on survival times.
Survival analysis accounts for censored data, where the event of interest may not have occurred for all subjects by the end of the study. This makes it particularly useful in longitudinal studies with varying follow-up times.
By mastering survival analysis, researchers can unravel critical insights into disease progression, treatment outcomes, and patient survival rates, ultimately advancing medical knowledge and enhancing patient care.
How to Solve Biostatistics Assignments
To excel in biostatistics assignments, begin by thoroughly understanding the problem statement and organizing the data. Choose appropriate statistical tests based on the research question and interpret the results accurately. Validate your findings and communicate them effectively in a well-structured report or presentation for a successful assignment completion.
a) Understand the Problem Statement
Understanding the problem statement is the first crucial step in tackling biostatistics assignments. Carefully read and analyze the given instructions to identify the research question, data type, and required analysis. Seek clarifications if needed to ensure a clear grasp of the task. A solid understanding of the problem will guide subsequent data handling, analysis, and interpretation, leading to accurate and meaningful results.
b) Organize the Data
Organizing data systematically is crucial in biostatistics assignments. Ensure all variables and observations are correctly labeled and input into a spreadsheet or statistical software. Handle missing values appropriately, and consider data transformations if necessary. A well-organized dataset streamlines the analysis process, reduces errors, and facilitates a clear understanding of the data, leading to more accurate and reliable conclusions.
c) Perform Descriptive Statistics
Descriptive statistics play a crucial role in biostatistics assignments by summarizing and presenting data in a meaningful manner. Calculating measures of central tendency, such as the mean and median, helps understand the data's typical values, while measures of variability, such as the standard deviation, provide insights into its spread. Visualization techniques, like histograms and box plots, aid in identifying patterns and outliers, facilitating a comprehensive data analysis process.
d) Apply the Appropriate Statistical Tests
Selecting the right statistical test is paramount in biostatistics assignments. Analyze the nature of the data and the research question to determine which test best suits the scenario. For comparing means, employ t-tests or ANOVA; for categorical data, use chi-square or Fisher's exact test. Logistic regression is valuable for predicting binary outcomes, while survival analysis examines time-to-event data. Accurate test selection ensures reliable and meaningful conclusions.
e) Interpret the Results
After conducting the statistical analysis, the interpretation of results is critical to draw meaningful conclusions. Analyze the p-values and confidence intervals to determine the significance of findings. Present the outcomes in the context of the research question and avoid overinterpretation. Acknowledge any limitations in the study and discuss potential implications of the results. A clear and concise interpretation enhances the value of biostatistics assignments.
f) Validate Your Results
Validating your results is a crucial step in biostatistics assignments to ensure accuracy and reliability. Recheck all calculations and verify that statistical tests are appropriately applied. Conduct sensitivity analyses or additional tests to test the robustness of your findings. By validating your results, you can confidently present your conclusions, knowing that they are based on sound statistical analysis and contribute meaningfully to the field of biostatistics.
g) Communicate Your Findings Clearly
Effective communication of findings is crucial in biostatistics assignments. Clearly present the research question, methods used, and the results obtained in a concise and coherent manner. Utilize visual aids like graphs, tables, and charts to enhance understanding. Interpret the statistical outcomes in the context of the research objectives and acknowledge any limitations. A well-structured and articulate presentation ensures your work is easily comprehensible and impactful.
Conclusion
Biostatistics assignments can be challenging, but with a solid understanding of key topics and a systematic problem-solving approach, you can tackle them effectively. By mastering probability, hypothesis testing, study design, descriptive statistics, regression analysis, and survival analysis, you will be well-equipped to analyze biological and health-related data. Remember to approach each assignment with a clear understanding of the problem statement, organize the data meticulously, and choose the appropriate statistical tests. Through diligent analysis and interpretation, you can draw meaningful conclusions and contribute to the field of biostatistics.