Claim Your Offer
Unlock a fantastic deal at www.statisticsassignmenthelp.com with our latest offer. Get an incredible 20% off on your second statistics assignment, ensuring quality help at a cheap price. Our expert team is ready to assist you, making your academic journey smoother and more affordable. Don't miss out on this opportunity to enhance your skills and save on your studies. Take advantage of our offer now and secure top-notch help for your statistics assignments.
We Accept
- Importance of Simulation
- Modeling for Deeper Insight
- Witness: A Simulation Software
- Understanding Witness Models
- Advantages of Witness for Statistics Assignments
- Monte Carlo Simulations
- Applications of Monte Carlo Simulations
- Monte Carlo Simulations for Statistics Assignments
- Conclusion
In the realm of statistics and data analysis, the ability to simulate and model real-world scenarios is an invaluable skill. Simulation and modeling techniques help us gain insights, make predictions, and solve complex problems in various fields, from business and engineering to healthcare and finance. In this comprehensive blog, we will explore the concepts of simulation and modeling, focusing on the use of Witness software and Monte Carlo simulations. These tools are not only powerful aids for understanding real-world phenomena but are also indispensable for students tackling statistics assignments.
When students embark on their statistical journey, they often encounter challenging assignments that require a deep understanding of statistical concepts and the ability to apply them to practical situations. This is where Witness software and Monte Carlo simulations come to their aid. Whether you need assistance with your simulation assignment using witness or seek to enhance your statistical modeling skills, this blog will provide valuable insights and guidance to help you excel in your academic endeavors.
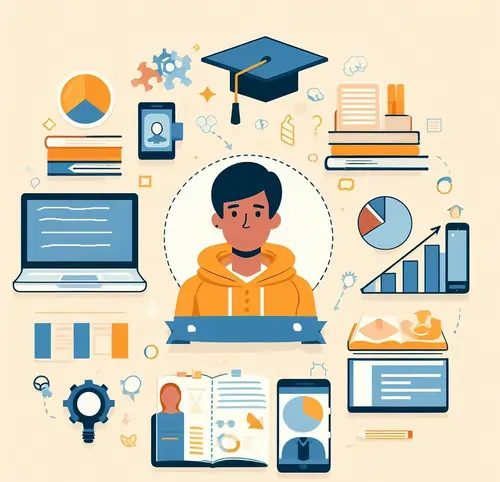
Now, let's delve into the world of simulation and modeling, exploring how these tools can empower you to tackle complex statistical problems with confidence and precision.
What is Simulation and Modeling?
Before we dive into the specifics of Witness and Monte Carlo simulations, let's establish a foundational understanding of simulation and modeling.
- Simulation
- Modeling
Simulation involves creating a computerized or mathematical model of a system or process to imitate its behavior over time. It allows us to study the system's performance, evaluate different scenarios, and make informed decisions without directly manipulating the real-world system. In essence, simulation is a virtual experiment.
Modeling is the process of constructing a simplified representation of a system or phenomenon. Models can be mathematical equations, diagrams, or computer programs. These representations help us analyze and understand complex real-world situations by breaking them down into manageable components.
Now, let's explore how Witness and Monte Carlo simulations fit into this framework.
Now, let's delve deeper into the fundamental concepts of simulation and modeling to gain a more comprehensive understanding of their significance in various fields.
Importance of Simulation
Simulation is a powerful tool used extensively across diverse domains, including engineering, healthcare, finance, and manufacturing. Its importance lies in its ability to provide a safe and controlled environment for experimentation and analysis. Here, we'll highlight a few key reasons why simulation is indispensable:
- Risk Reduction: In scenarios where real-world experimentation is costly, dangerous, or impractical, simulation steps in as a safer alternative. For instance, testing the impact of a new drug on patients can be risky, but simulating patient responses in a controlled digital environment can mitigate those risks.
- Cost Savings: Developing and fine-tuning real-world systems can be expensive. Simulation allows organizations to test multiple configurations and scenarios without incurring substantial costs. This is especially beneficial in manufacturing and product development.
- Performance Optimization: Simulation enables the optimization of complex systems. Engineers can use simulations to refine designs, make adjustments, and improve the efficiency of processes, ultimately leading to better performance outcomes.
- Scenario Analysis: Whether in finance, emergency response, or urban planning, simulation empowers decision-makers to explore a range of possible outcomes and scenarios. This aids in strategic planning, risk assessment, and resource allocation.
Modeling for Deeper Insight
Modeling, on the other hand, is a fundamental aspect of simulation. It's the art and science of creating simplified representations of complex systems. Let's delve into why modeling is crucial:
- Abstraction: Complex real-world systems often involve numerous variables and interactions. Modeling simplifies these complexities by focusing on the most relevant aspects. This abstraction makes it easier to analyze and understand the system.
- Predictive Power: Models serve as tools for prediction. By representing a system mathematically or visually, we can use these models to forecast outcomes under different conditions. This predictive power is invaluable in fields like weather forecasting, where models help anticipate storms and temperature changes.
- Exploration: Models encourage exploration and experimentation. They allow us to manipulate variables, test hypotheses, and explore "what if" scenarios. For instance, climate models help scientists study the potential effects of various emissions scenarios on global temperatures.
- Communication: Models facilitate communication among experts and stakeholders. They provide a common language to discuss complex systems and convey ideas. Architects, for instance, use architectural models to convey design concepts to clients and builders.
Incorporating these expanded insights into the concepts of simulation and modeling underscores their significance in solving real-world problems. Witness and Monte Carlo simulations, as we will explore, leverage these principles to address complex challenges and provide valuable insights.
Witness: A Simulation Software
Witness is a popular simulation software used in various industries for modeling and analyzing complex systems. It provides a platform to create dynamic models, simulate real-world processes, and assess system performance. For students tackling statistics assignments, Witness offers a versatile tool to apply statistical concepts to practical problems.
Understanding Witness Models
Witness models are built using a visual interface, making it accessible to users with varying levels of programming experience. The software allows you to design intricate systems by defining entities, resources, and interactions. Here's a brief overview of key components in a Witness model:
- Entities
- Resources
- Queues
- Processes
Entities represent objects or individuals in the system. They move through the simulation based on predefined rules, mimicking real-world behavior.
Resources are assets or constraints that entities require or interact with during the simulation. Managing resources efficiently is critical for optimizing system performance.
Queues are where entities wait for their turn to access resources or undergo processing. Understanding queue dynamics is vital for improving system efficiency.
Processes define the actions entities take within the system. These can include tasks, activities, or any other actions relevant to the simulation.
Advantages of Witness for Statistics Assignments
Witness offers several advantages when it comes to statistics assignments:
- Realistic Scenarios
- Experimentation
- Data Analysis
- Decision Support
- Flexibility and Versatility
Witness allows students to create realistic scenarios and simulate data generation processes. This is invaluable for understanding statistical concepts like probability distributions and sampling.
Students can design experiments within Witness to test hypotheses and explore the impact of different variables on system performance. This aligns with statistical experimentation principles.
Witness provides tools for collecting and analyzing data generated during simulations. Students can apply statistical techniques to draw meaningful conclusions from the simulated data.
Using Witness, students can model decision-making processes under uncertainty. This is particularly useful for assignments related to decision analysis and risk assessment.
Witness can be applied to a wide range of scenarios, from manufacturing processes to supply chain management. This flexibility allows students to adapt their simulations to specific assignments.
Now that we've covered the basics of Witness, let's delve into another powerful tool: Monte Carlo simulations.
Monte Carlo Simulations
Monte Carlo simulations are a statistical technique used to model the behavior of complex systems by generating random samples from known probability distributions. Named after the famous Monte Carlo Casino, this approach relies on randomness to approximate solutions to problems that may be deterministic in nature.
Key Elements of Monte Carlo Simulations
Monte Carlo simulations involve the following key elements:
- Random Sampling
- Probability Distributions
- Simulation Runs
- Statistical Analysis
The heart of Monte Carlo simulations lies in random sampling. It involves repeatedly drawing random samples from probability distributions to estimate outcomes.
To perform Monte Carlo simulations, you need a good understanding of probability distributions. Common distributions include the normal distribution, uniform distribution, and exponential distribution.
Monte Carlo simulations typically involve running thousands or even millions of iterations to obtain reliable results. The law of large numbers ensures that as the number of iterations increases, the simulation's outcomes converge to the true values.
After running the simulations, statistical analysis is performed on the results to calculate means, variances, and other relevant statistics.
Applications of Monte Carlo Simulations
Monte Carlo simulations are incredibly versatile and find applications in various fields:
- Finance
- Engineering
- Healthcare
- Project Management
- Environmental Science
In finance, Monte Carlo simulations are used to model stock price movements, estimate portfolio risks, and assess options pricing.
Engineers use Monte Carlo simulations to analyze the reliability of systems, such as bridges, aircraft, and manufacturing processes.
In healthcare, Monte Carlo simulations help optimize treatment plans, assess patient outcomes, and simulate disease spread.
Project managers use Monte Carlo simulations to estimate project durations and costs, accounting for uncertainties and risks.
Environmental scientists employ Monte Carlo simulations to model environmental processes, including climate change scenarios and pollutant dispersion.
Now, let's see how Monte Carlo simulations can be a valuable tool for students working on statistics assignments.
Monte Carlo Simulations for Statistics Assignments
Monte Carlo simulations offer several benefits for students studying statistics:
- Hands-On Learning
- Visual Representation
- Problem Solving
- Real-World Applications
- Risk Assessment
- Sensitivity Analysis
- Hypothesis Testing
Students can gain a deep understanding of probability distributions and statistical concepts by actively designing and running simulations.
Simulations provide visual representations of data, making it easier for students to grasp abstract statistical concepts.
Statistics assignments often involve complex problems. Monte Carlo simulations can help break down these problems into manageable parts, allowing students to tackle them step by step.
Monte Carlo simulations bridge the gap between theory and practice, as students can apply statistical knowledge to real-world scenarios.
Many statistics assignments involve risk assessment and decision-making. Monte Carlo simulations are well-suited for modeling uncertainties and providing insights into optimal decision paths.
Students can explore how changes in input parameters impact the outcomes, a crucial aspect of understanding system behavior.
Monte Carlo simulations can be used for hypothesis testing, enabling students to assess the significance of their findings.
Conclusion
Simulation and modeling, as facilitated by Witness and Monte Carlo simulations, are invaluable tools for students studying statistics. Witness provides a user-friendly platform to simulate and analyze complex systems, while Monte Carlo simulations offer a statistical approach to modeling uncertainty and randomness.
By incorporating these tools into their studies, students can develop practical skills, gain a deeper understanding of statistical concepts, and confidently tackle assignments that involve real-world data and scenarios. Whether you're exploring the intricacies of a manufacturing process or estimating the financial risks of an investment portfolio, Witness and Monte Carlo simulations are your trusted companions in the world of statistics.
So, whether you're a student embarking on a statistics assignment or a professional seeking to enhance your analytical toolkit, remember that simulation and modeling are your keys to unlocking insights and solving complex problems in a data-driven world. Embrace the power of Witness and Monte Carlo simulations to excel in the fascinating realm of statistics and data analysis.