Claim Your Offer
Unlock a fantastic deal at www.statisticsassignmenthelp.com with our latest offer. Get an incredible 10% off on all statistics assignment, ensuring quality help at a cheap price. Our expert team is ready to assist you, making your academic journey smoother and more affordable. Don't miss out on this opportunity to enhance your skills and save on your studies. Take advantage of our offer now and secure top-notch help for your statistics assignments.
We Accept
- Essential Topics to Master Before Starting RapidMiner Assignments
- 1. Data Preprocessing
- 2. Exploratory Data Analysis (EDA)
- 3. Machine Learning Algorithms
- 4. Model Evaluation and Selection
- 5. Feature Engineering
- 6. Time Series Analysis (if applicable)
- 7. RapidMiner Basics
- Approaching RapidMiner Assignments: Strategies for Success
- Conclusion
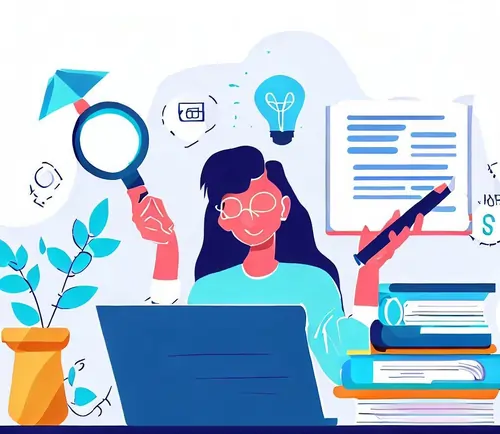
In the realm of data science and machine learning, RapidMiner stands as a versatile and powerful tool that empowers analysts to extract valuable insights from raw data. As you embark on your journey to master RapidMiner, you'll likely encounter assignments that test your understanding and proficiency. To ace these assignments, it's essential to grasp a range of foundational topics and develop effective problem-solving strategies. This blog will guide you through the crucial topics you need to know before tackling RapidMiner assignments and provide you with strategies to approach and conquer these assignments.
Essential Topics to Master Before Starting RapidMiner Assignments
RapidMiner is a powerful data science platform that empowers analysts to extract insights from data through a user-friendly interface. To complete your RapidMiner assignment effectively, you need to be well-versed in fundamental concepts that underpin the tool's functionality. Here are the essential topics you should know before diving into RapidMiner assignments:
1. Data Preprocessing
Data preprocessing is the cornerstone of effective analysis. By addressing missing values, and outliers, and transforming data, you ensure its quality and accuracy. Proper preprocessing enhances the reliability of your results and prevents skewed conclusions. Furthermore, feature selection plays a pivotal role in simplifying models and improving their performance. Mastering these preprocessing techniques is essential for a successful RapidMiner assignment. Understand key techniques like:
- Data Cleaning: Data cleaning is the initial step towards reliable insights. Identifying and handling missing values, duplicates, and outliers ensures the accuracy of your analysis. In RapidMiner assignments, proficiency in data cleaning guarantees that your models are built on sound foundations, leading to more robust and meaningful conclusions. Clean data reduces bias and enhances the effectiveness of subsequent preprocessing and modeling stages.
- Data Transformation: Data transformation is a critical step in data preprocessing. It involves normalizing, scaling, and encoding categorical variables, ensuring data consistency and comparability. Normalization brings all features to a uniform scale, preventing bias towards certain attributes. Scaling maintains relationships between variables while accommodating different measurement units. Categorical encoding transforms categorical data into numerical values for analysis. Skillfully applying these techniques empowers you to harness the full potential of your data in RapidMiner assignments.
- Feature Selection: Feature selection is a critical step in refining your analysis. By selecting the most relevant features, you simplify models, reduce overfitting, and enhance predictive accuracy. Through careful selection, you focus on the variables that truly influence the outcome, streamlining the complexity of your analysis. Skillful feature selection ensures that your RapidMiner assignments are efficient, interpretable, and yield meaningful insights from the data.
2. Exploratory Data Analysis (EDA)
Exploratory Data Analysis (EDA) is the compass that guides your analysis journey. Through descriptive statistics and visualizations, you unveil hidden patterns and relationships within your data. EDA provides insights into data distribution, outliers, and potential correlations, offering a roadmap for subsequent analysis steps. By mastering EDA, you equip yourself to make informed decisions when approaching RapidMiner assignments and extract the full value from your data. Familiarize yourself with:
- Descriptive Statistics: Descriptive statistics are the storytellers of your data. They offer a snapshot of central tendencies, dispersions, and distributions, painting a clear picture of your dataset's characteristics. By understanding measures like mean, median, and standard deviation, you gain insights into the data's overall behavior. These statistics serve as the foundation for effective decision-making during data analysis, ensuring your RapidMiner assignments are grounded in accurate information.
- Data Visualization: Data visualization is the bridge between raw data and insightful understanding. Through graphs and plots, you transform complex datasets into visual narratives that are easy to grasp. Visualizations aid in identifying trends, anomalies, and patterns that might go unnoticed in raw data. Proficiency in creating and interpreting visualizations empowers you to communicate findings effectively and make informed decisions during your RapidMiner assignments.
- Correlation Analysis: Correlation analysis is a lens that uncovers connections between variables. By understanding the degree and direction of relationships, you gain insights into potential dependencies that impact your analysis. RapidMiner's correlation analysis tools enable you to pinpoint variables that influence each other, guiding your feature selection and model-building processes. Adept correlation analysis enhances the precision of your RapidMiner assignments and strengthens the foundation of your data-driven decisions.
3. Machine Learning Algorithms
Machine Learning Algorithms are the toolkit for turning data into predictions and insights. By mastering algorithms like classification, regression, and clustering, you unlock the potential to solve diverse problems. Understanding how these algorithms work, their strengths, and when to apply them is crucial for selecting the right approach in your RapidMiner assignments, leading to accurate and meaningful results. Understand the core machine learning algorithms and their applications:
- Classification Algorithms: Classification algorithms are data science's classifiers, sorting data into predefined categories. By learning algorithms like Decision Trees, SVMs, and Random Forests, you gain the ability to classify data points accurately. RapidMiner's classification tools empower you to predict outcomes and make informed decisions. A strong grasp of classification algorithms equips you to tackle diverse tasks in RapidMiner assignments, from sentiment analysis to fraud detection, with confidence and precision.
- Regression Algorithms: Regression algorithms are the compass for predicting continuous outcomes. Whether linear or more advanced like Lasso and Ridge, they model relationships between variables. In RapidMiner, understanding regression algorithms empowers you to make accurate predictions and quantify the impact of different factors on your target variable. Proficiency in regression algorithms allows you to handle diverse assignment scenarios, from price predictions to risk assessments, with confidence and precision.
- Clustering Algorithms: Clustering algorithms are the compass guiding you through complex data landscapes. By categorizing data points into meaningful groups, you uncover hidden structures and patterns. RapidMiner's clustering algorithms allow you to segment data based on similarities, aiding in customer segmentation, anomaly detection, and more. Proficiency in clustering equips you to navigate intricate data sets, enhancing the quality and depth of insights in your RapidMiner assignments.
- Dimensionality Reduction: Dimensionality reduction is the compass through high-dimensional data landscapes. By condensing variables while retaining meaningful information, you alleviate the curse of dimensionality and enhance model performance. RapidMiner's dimensionality reduction techniques like PCA and t-SNE empower you to navigate intricate datasets effectively. Skillful application of dimensionality reduction ensures your RapidMiner assignments are efficient, accurate, and maintain the integrity of your insights.
4. Model Evaluation and Selection
Model Evaluation and Selection is the compass guiding your analytical journey. By assessing models through metrics like precision, recall, and cross-validation, you ensure their reliability and generalization. This step aids in distinguishing between underperforming and robust models, allowing you to fine-tune your approach for optimal results in your RapidMiner assignments. Learn how to assess model performance and choose the right metrics:
- Accuracy Metrics: Accuracy metrics provide a clear view of your model's performance. Metrics like precision, recall, and F1-score reveal how well your model classifies instances, especially in imbalanced datasets. These insights guide your model refinement, ensuring it meets the desired standards. Utilizing RapidMiner's array of accuracy metrics equips you to confidently gauge and enhance the effectiveness of your models in various contexts during your assignments.
- Cross-Validation: Cross-validation is the sentinel of robust model assessment. By partitioning data into subsets, training and testing models iteratively, you guard against overfitting and validate generalization. RapidMiner's cross-validation tools empower you to evaluate model performance realistically, enhancing your confidence in the results of your assignments. Skillful cross-validation ensures that your models are dependable, making your RapidMiner analysis a beacon of accuracy.
- Hyperparameter Tuning: Hyperparameter tuning is the tuning fork for model optimization. By adjusting parameters that aren't learned from data, you fine-tune model performance. In RapidMiner, meticulous hyperparameter tuning can transform a good model into an exceptional one. Learning how to navigate the trade-offs and intricacies of hyperparameter tuning equips you to extract maximum value from your models and excel in your RapidMiner assignments.
5. Feature Engineering
Feature engineering is the creative brushstroke that enhances your analysis. By crafting new features or modifying existing ones, you capture hidden insights within the data. In RapidMiner assignments, skillful feature engineering amplifies the predictive power of your models, allowing you to uncover nuanced relationships and elevate your data-driven decisions. Feature engineering enhances model predictive power:
- Creating New Features: Crafting new features breathes life into your data. By combining existing attributes or generating novel ones, you amplify the potential for uncovering hidden patterns. This creative process enables your RapidMiner assignments to capture nuanced relationships that might otherwise go unnoticed, enriching your analyses with depth and insight.
- Feature Scaling: Scaling features creates harmony in your data. By ensuring variables are on comparable scales, you facilitate smoother model convergence. In your RapidMiner assignments, mastering techniques like normalization and standardization helps prevent certain features from overshadowing others, leading to more balanced and accurate results.
- One-Hot Encoding: One-hot encoding liberates categorical data. By converting categorical variables into binary vectors, you enable models to comprehend and incorporate this information effectively. In RapidMiner assignments, proficient one-hot encoding equips your models to handle categorical variables seamlessly, enhancing their predictive capabilities and enriching your analytical outcomes.
6. Time Series Analysis (if applicable)
Time Series Analysis adds a temporal dimension to your insights. By understanding trends, seasonality, and cyclic patterns within time-dependent data, you can make accurate predictions and strategic decisions. If your RapidMiner assignments involve time series data, mastering these techniques empowers you to unravel the dynamics of time and harness its predictive power effectively. If your assignments involve time-dependent data, grasp the basics of time series analysis:
- Time Series Components: Understanding trends, seasonality, and noise in time series data.
- Time Series Modeling: Applying techniques like ARIMA, Exponential Smoothing, etc.
7. RapidMiner Basics
RapidMiner Basics lay the groundwork for your data exploration journey. Navigating operators, constructing processes, and interpreting visualizations are essential skills. By mastering these fundamentals, you gain the ability to construct efficient workflows for data manipulation, analysis, and model building. RapidMiner's user-friendly interface becomes your canvas, allowing you to craft insightful analyses and confidently tackle assignments in your data science endeavors. Ensure you are comfortable navigating the RapidMiner interface:
- Operators: Understanding various operators for data loading, preprocessing, modeling, and evaluation.
- Processes: Creating and connecting operators to form a workflow for analysis.
- Results and Visualizations: Interpreting and presenting outcomes from RapidMiner processes.
Approaching RapidMiner Assignments: Strategies for Success
With a solid grasp of these foundational topics, you're now equipped to tackle RapidMiner assignments with confidence. Steps to follow:
- Read the Assignment Prompt Carefully: Before diving into the data, thoroughly understand the assignment requirements. Identify the goals, tasks, and specific questions you need to address.
- Plan Your Workflow: Outline the sequence of operators and steps you'll need to execute in RapidMiner to complete the assignment. This preliminary planning will save you time and prevent confusion during the process.
- Data Preprocessing is Key: Spend a significant portion of your time on data preprocessing. Clean, transform, and prepare your data meticulously. Ensure you handle missing values, outliers, and data inconsistencies appropriately.
- Document Your Process: As you build your RapidMiner process, document each step. This documentation will not only help you keep track of your progress but also serve as a valuable reference for explaining your methodology later.
- Iterative Approach to Model Selection: Don't hesitate to try multiple algorithms to find the best fit for your assignment. Experiment with different models, adjusting parameters as needed. Use evaluation metrics to compare and contrast their performance.
- Validate and Tune: Implement proper validation techniques, such as cross-validation, to ensure your models generalize well. Tweak parameters based on validation results to achieve optimal performance.
- Interpret and Communicate Results: Once you've obtained results, interpret them in the context of the assignment's objectives. Prepare clear visualizations and explanations to convey your findings effectively.
- Reflect and Learn: After completing the assignment, take time to reflect on your approach. Learning from each assignment will contribute to your overall growth.
Conclusion
Mastering RapidMiner assignments requires a solid understanding of foundational topics and a strategic problem-solving approach. By familiarizing yourself with RapidMiner's basics, data preprocessing, EDA, machine learning algorithms, model evaluation, feature engineering, and potentially time series analysis, you'll be well-prepared to tackle a variety of assignments. Remember to approach assignments systematically, from careful planning to thorough documentation and effective communication of results. With persistence and practice, you'll become adept at navigating RapidMiner's intricacies and excel in your data science journey.