An Insight into Data Visualization Techniques
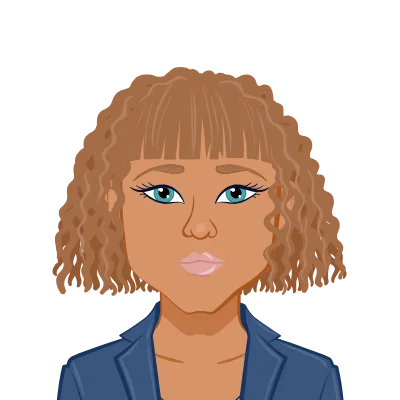
The Importance of Data Visualization
Data visualization is often considered a bridge between raw data and meaningful insights. Our brains are inherently wired to process visual information more effectively than raw numbers or text. Visualizations bring data to life by presenting patterns, trends, and relationships in a way that's easily digestible. Here's why data visualization is so important:
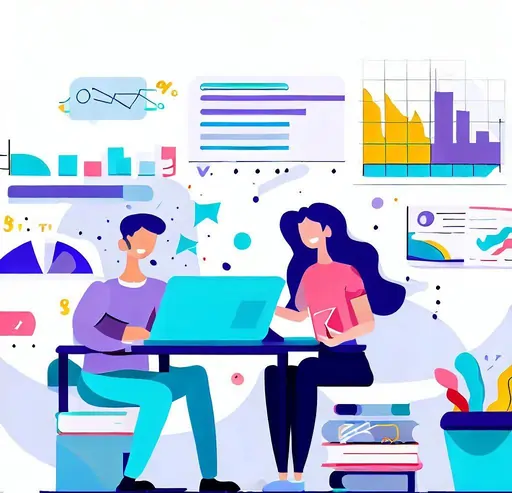
- Clarity and Understanding: Complex datasets can be challenging to comprehend. Visualizations simplify complex concepts and highlight key points, making it easier for individuals to understand the information.
- Identifying Patterns and Trends: Visualizations can reveal patterns and trends that might go unnoticed when looking at raw data. This insight is invaluable for making predictions and informed decisions.
- Storytelling: Visualizations have the power to tell a compelling story about the data. They help convey information in a way that captures the audience's attention and makes the narrative more memorable.
- Effective Communication: When working in teams or presenting to stakeholders, visualizations facilitate clear communication by presenting data in a format that's easily shareable and understood.
- Quick Decision-Making: Well-designed visualizations allow decision-makers to grasp information quickly, enabling them to make faster and more accurate decisions.
Types of Data Visualization Techniques
Data visualization is a powerful way to transform raw data into meaningful insights. Different types of data visualization techniques serve specific purposes and are tailored to different types of data. Here's an in-depth exploration of some of the most common and effective data visualization techniques:
- Bar Charts and Column Charts
- Line Charts
- Pie Charts
- Scatter Plots
- Heatmaps
- Histograms
- Bubble Charts
- Choropleth Maps
- Tree Maps
- Network Diagrams
Bar charts and column charts are simple yet effective ways to compare data across different categories or groups. In these charts, the length of the bars or columns represents the values they correspond to. These visualizations are particularly useful for displaying categorical data and illustrating relationships between variables. For instance, a bar chart could be used to compare sales figures across different regions or the popularity of different movie genres.
Line charts are ideal for visualizing trends over time. They use lines to connect data points, allowing viewers to easily identify fluctuations and patterns in the data. Line charts are commonly used in stock market analysis to track the performance of stocks over time or in tracking temperature changes over seasons.
Pie charts are used to represent parts of a whole. The circular chart is divided into segments, with each segment's size proportional to the value it represents. Pie charts are useful for showing the distribution of percentages within a dataset, such as the composition of expenses in a budget or the distribution of different types of crimes in a city.
Scatter plots are excellent for revealing relationships and correlations between two variables. Each data point is represented as a dot on the graph, with one variable plotted along the x-axis and the other along the y-axis. Scatter plots are valuable for identifying patterns, outliers, and clusters within data, making them popular in scientific research and data analysis.
Heatmaps use color gradients to represent values within a matrix. They are particularly effective for visualizing large datasets and identifying patterns or trends in tabular data. Heatmaps are frequently used in fields like biology (gene expression analysis), finance (stock market data analysis), and social sciences (survey responses analysis).
Histograms display the distribution of continuous data by dividing it into bins and representing the frequency or count of data points within each bin. They're valuable for understanding the underlying distribution of data and identifying trends, gaps, or outliers. Histograms are commonly used in fields such as demographics (age distribution) and quality control (measurement variability).
Bubble charts extend the concept of scatter plots by introducing a third variable represented by the size of the dots (bubbles). The x and y positions of the bubbles represent two variables, while the size of the bubbles represents a third dimension. Bubble charts are useful for visualizing complex relationships involving multiple variables.
Choropleth maps use color variations to represent data values in different geographic regions. These maps are widely used to visualize data related to population density, election results, or any data that can be tied to specific geographic areas. The varying colors provide an immediate visual comparison across regions.
Tree maps are hierarchical visualizations that use nested rectangles to represent data. They're particularly suitable for displaying proportions and relationships within hierarchical structures. Tree maps are often used in financial analysis to represent portfolio compositions or in file systems to show storage usage.
Network diagrams visualize relationships between entities in a network. Nodes represent entities, and edges represent the connections between them. Network diagrams are commonly used in social network analysis, biology (protein-protein interactions), and transportation (airline route networks).
Best Practices for Effective Data Visualization
Creating meaningful and impactful data visualizations requires adhering to certain best practices. Here are some guidelines to keep in mind:
- Know Your Audience:Understand who will be viewing your visualizations and tailor them to their level of expertise and interests. A chart meant for executives might differ from one intended for data analysts.
- Simplicity is Key: Keep your visualizations simple and focused. Avoid clutter and unnecessary elements that can distract from the main message.
- Choose the Right Chart Type: Select a chart type that best represents your data and supports the insights you want to convey. Using an inappropriate chart can lead to confusion and misinterpretation.
- Use Appropriate Colors: Colors should enhance, not detract from, the visualization. Choose a color palette that's easy on the eyes and conveys information effectively. Use color to highlight important points.
- Label Clearly: Ensure that axes, data points, and any other relevant elements are properly labeled. Labels provide context and prevent misunderstandings.
- Provide Context: Add titles, subtitles, and descriptions to your visualizations to provide context and help viewers understand the significance of the data presented.
- Avoid Misleading Visualizations: Be honest and accurate in representing data. Avoid distorting the scale, using 3D effects that can distort perception, or truncating axes to exaggerate differences.
- Tell a Story: Arrange your visualizations in a logical sequence to tell a coherent story. Guide your audience through the data, highlighting key points along the way.
- Interactive Elements: When appropriate, add interactive elements to your visualizations. Interactive features allow viewers to explore the data on their own and extract insights that are relevant to them.
- Regular Updates: If your data changes over time, update your visualizations accordingly. Stale or outdated visualizations can lead to incorrect conclusions.
Tools for Data Visualization
In the dynamic landscape of data visualization, having access to the right tools can significantly impact the quality and impact of your visualizations. Here, we'll delve deeper into some of the prominent tools used for data visualization, each catering to different user needs and expertise levels.
- Tableau
- Power BI
- D3.js
- Matplotlib and Seaborn
- Google Data Studio
- Plotly
- QlikView
Tableau stands out as one of the most popular and user-friendly data visualization tools. It offers an intuitive drag-and-drop interface that allows users to create visually appealing and interactive dashboards without requiring extensive coding skills. Tableau supports a wide variety of chart types, from simple bar charts to complex geographical maps. One of its standout features is its ability to handle large datasets and quickly generate insights from them. Its interactive dashboards enable users to explore data from multiple angles and gain deeper insights through filters, drill-downs, and tooltips.
Microsoft Power BI is another powerful tool that's widely used for creating interactive data visualizations. Integrated seamlessly with other Microsoft products, Power BI is especially beneficial for users already familiar with the Microsoft ecosystem. It offers robust data transformation capabilities, allowing users to clean, shape, and model data before visualizing it. Power BI's strength lies in its ability to connect to various data sources, making it a preferred choice for businesses that deal with diverse datasets. Additionally, its sharing and collaboration features make it ideal for teams working together on data-driven projects.
D3.js (Data-Driven Documents) is a JavaScript library that empowers developers and advanced users to create custom data visualizations with unparalleled flexibility and control. While it demands coding skills, D3.js offers complete control over the creation process, enabling users to craft unique and intricate visualizations that suit their specific needs. This library provides the building blocks for creating interactive and dynamic visuals by binding data to the Document Object Model (DOM). D3.js's extensive community and documentation support make it a go-to choice for those seeking to push the boundaries of data visualization.
For Python enthusiasts, matplotlib and Seaborn are powerful libraries for crafting static and interactive visualizations. Matplotlib is a foundational library that offers a wide range of plotting functionalities and can be used to create basic visualizations quickly. Seaborn, on the other hand, builds on top of matplotlib and provides a higher-level interface with stylish pre-defined themes, making it easy to generate aesthetically pleasing visualizations. Both libraries are particularly suitable for data exploration and analysis, making them valuable tools for researchers, analysts, and data scientists.
Google Data Studio is a free tool that allows users to create customizable and shareable dashboards using data from various sources, including Google Analytics, Google Sheets, and other third-party databases. Its web-based interface simplifies the process of creating interactive reports and dashboards, making it accessible even to users with limited technical skills. With real-time collaboration features, users can work together on visualizations, enhancing the team's ability to analyze and communicate insights effectively.
Plotly is a versatile visualization library available in Python, R, and JavaScript. Known for its ability to create interactive visualizations, Plotly is suitable for both static and dynamic data presentations. Its interactive features, such as hover effects and zooming, allow users to explore data points in detail. With Plotly, you can create a variety of chart types, including line charts, scatter plots, bar charts, and 3D visualizations. It's a valuable tool for data scientists, researchers, and analysts who want to create engaging and exploratory visualizations.
QlikView is a comprehensive business intelligence platform that provides data visualization and discovery tools. While it's more oriented towards business users and analysts, it offers robust capabilities for creating dynamic dashboards and reports. QlikView's associative data model allows users to explore data relationships and uncover insights through interactive selections. Its intuitive interface enables users to build visualizations and dashboards without extensive technical knowledge.
Conclusion
Data visualization is a powerful tool that transcends industries and roles. By transforming complex data into meaningful visual representations, we can unlock insights, tell compelling stories, and make informed decisions. Understanding different types of visualizations, adhering to best practices, and utilizing appropriate tools are essential steps toward becoming proficient in the art of data visualization. As technology continues to advance, data visualization will remain a cornerstone of effective communication in the data-driven landscape. So, whether you're a data scientist, a business analyst, or a researcher, mastering data visualization techniques will undoubtedly enhance your ability to extract valuable insights from the data at hand.