Understanding Moments, Skewness, and Kurtosis: A Key to Solving Statistics Assignments
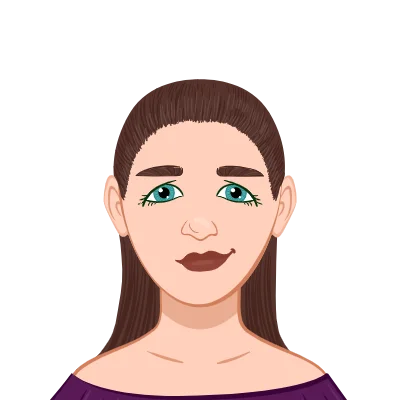
To complete your dispersion assignment using Statistics, it's important to recognize that Statistics, often considered the backbone of data-driven decision-making, encompasses a multitude of complex concepts. Among these, moments, skewness, and kurtosis stand out as fundamental tools that illuminate the characteristics of datasets. Moments, including the mean, variance, skewness, and kurtosis, offer a comprehensive framework for describing the central tendency, dispersion, and shape of data distributions. These measures provide invaluable insights for students navigating the intricate terrain of statistics assignments. From determining the stability of financial investments to analyzing social phenomena, moments serve as the foundation upon which statistical analyses are built.
Skewness, the measure of a distribution's asymmetry, reveals essential details about the shape of data, indicating whether it leans towards larger or smaller values. Kurtosis, on the other hand, gauges the tailedness of a distribution, shedding light on the likelihood of extreme values. Together, these concepts empower students to not only comprehend the complexities of data but also to solve intricate statistical problems. By delving into the depths of moments, skewness, and kurtosis, students embark on a journey that equips them with the analytical prowess needed to decipher real-world phenomena, ensuring that their statistical assignments are not just completed, but mastered with a deep understanding of the underlying principles.

Moments – The Foundation of Statistical Analysis
Moments serve as the bedrock of statistical analysis, providing a comprehensive framework for understanding the fundamental characteristics of a dataset. Within the realm of moments, the mean stands as the pivotal measure of central tendency, offering a glimpse into the average value around which the data points revolve. Variance, another vital moment, quantifies the extent of data dispersion, revealing how much individual data points deviate from the mean. Skewness, the third moment, delves into the asymmetry of the distribution, determining whether the data leans towards higher or lower values. Lastly, kurtosis, the fourth moment, unveils the shape of the tails of the distribution, indicating the likelihood of extreme values. These measures not only summarize the data but also empower statisticians and students alike to draw meaningful comparisons, identify outliers, and conduct hypothesis testing, making moments an indispensable foundation upon which statistical analyses are built.
What are Moments?
Moments are fundamental statistical measures that provide crucial insights into the shape and characteristics of a dataset. They offer a systematic way to summarize data, helping statisticians and researchers make meaningful interpretations. The concept of moments encompasses various statistical parameters, including the mean, variance, skewness, and kurtosis. The first moment, the mean, represents the central tendency of the data, indicating its average value. The second moment, variance, quantifies the spread or dispersion of the data points around the mean. Skewness, the third moment, measures the asymmetry of the distribution, indicating whether the data is skewed to the left or right. Lastly, kurtosis, the fourth moment, characterizes the tails of the distribution, providing insights into the presence of extreme values. Understanding these moments is fundamental for analyzing data, making comparisons, detecting outliers, and drawing meaningful conclusions in various statistical assignments and real-world applications.
Mean – The First Moment
The mean, often regarded as the cornerstone of statistical analysis, is a fundamental concept representing the central tendency of a dataset. It embodies the essence of a set of values, calculated by summing all the data points and dividing the total by the number of observations. In the realm of statistics assignments, understanding and computing the mean is akin to deciphering the heartbeat of a dataset. It serves as a compass, guiding students through a sea of numbers, allowing them to grasp the data's core essence. Whether determining the average income of a population, analyzing exam scores, or evaluating the central performance of a business metric, the mean provides a stable foundation upon which further statistical analyses can be built. Its simplicity belies its importance, for it is the mean that unveils the story hidden within the numbers, making it an indispensable tool for every statistician and student alike.
Variance – The Second Moment
Variance, the second moment in statistics, serves as a crucial measure of a dataset's dispersion and spread. In essence, it quantifies how much individual data points deviate from the mean. A high variance indicates that data points are widely scattered around the mean, suggesting greater diversity, while a low variance implies that the data points are clustered closely around the mean, indicating more uniformity. Calculating variance involves taking the average of the squared differences between each data point and the mean of the dataset. This mathematical concept is fundamental for students grappling with statistical assignments, as it not only provides insight into the data's variability but also forms the basis for various advanced statistical analyses, making it an indispensable tool in the realm of data interpretation and decision-making. Understanding variance equips students with the ability to assess the stability and reliability of datasets, allowing them to draw meaningful conclusions and make informed judgments in diverse fields of study.
Skewness – The Third Moment
Skewness, as the third moment of a distribution, plays a pivotal role in understanding the asymmetry of data. This statistical measure provides crucial insights into the shape of a dataset, indicating whether the distribution leans towards larger values (positive skewness) or smaller values (negative skewness). In essence, skewness helps students unravel the mysteries of data patterns, offering valuable clues about the distribution's behavior. When a dataset exhibits positive skewness, it implies that the tail on the right side is longer or fatter than the left side, indicating a concentration of high values. Conversely, negative skewness suggests a longer or fatter tail on the left side, signifying an abundance of low values. By grappling with problems related to skewness, students refine their analytical skills, enabling them to discern trends and irregularities within datasets, a proficiency invaluable in various statistical assignments and real-world applications.
Kurtosis – The Fourth Moment
Kurtosis, often referred to as the fourth moment, is a pivotal statistical measure that extends our understanding of data distribution beyond the variance. While mean, variance, and skewness provide crucial insights, kurtosis delves deeper into the shape of a dataset, specifically focusing on the tails of the distribution. This measure quantifies the relative weight of the tails compared to the rest of the distribution, indicating the probability of extreme values. A positive kurtosis suggests heavy tails and a sharper peak, indicating a higher likelihood of extreme outcomes, while a negative kurtosis indicates lighter tails and a flatter peak, signifying a decreased probability of outliers. Understanding kurtosis is essential in fields such as finance, where assessing the risk associated with investment portfolios relies heavily on predicting extreme events. Moreover, in data analysis, recognizing the kurtosis of a dataset aids in model selection, ensuring that statistical methods are appropriately tailored to the distribution's characteristics, thus enhancing the accuracy and reliability of analytical outcomes.
Solving Statistical Problems with Moments
Solving statistical problems with moments is akin to unlocking the secrets hidden within data distributions. Moments, encompassing mean, variance, skewness, and kurtosis, serve as powerful tools for students navigating the intricate landscape of statistics assignments. These measures provide a structured approach for data comparison, aiding in the assessment of central tendency, dispersion, asymmetry, and tailedness of datasets. Through moments, students can discern patterns, identify outliers, and make informed decisions in various scenarios. When faced with assignments, understanding how to utilize moments equips students to detect biases, perform hypothesis tests, and analyze time series data effectively. By leveraging moments, students not only unravel the complexities of datasets but also develop critical analytical skills that are invaluable in real-world applications.
Using Moments for Data Comparison
Using moments for data comparison provides a robust framework for students to analyze and contrast different datasets effectively. By calculating the mean and variance of datasets, students can quantify the central tendency and dispersion, respectively, allowing for a precise understanding of each dataset's characteristics. This information is invaluable when making comparisons, enabling students to identify patterns, trends, and anomalies within the data. Moments offer a quantitative basis for assessing the stability and reliability of datasets, aiding in decision-making processes. Whether comparing financial investments, examining exam scores, or evaluating manufacturing processes, moments serve as essential tools, empowering students to draw insightful conclusions and make data-driven recommendations in various real-world scenarios.
Identifying Outliers with Moments
Identifying outliers in a dataset is a crucial aspect of statistical analysis, and moments, particularly skewness and kurtosis, play a significant role in this process. Skewness helps in detecting the asymmetric spread of data, indicating potential outliers on one side of the distribution, while kurtosis helps assess the tails of the distribution, where extreme values might exist. By understanding these moments, students can effectively pinpoint data points that deviate significantly from the rest, allowing them to recognize unusual patterns or errors within the dataset. This skill is invaluable in various fields, enabling students to ensure the accuracy and reliability of their analyses and make informed decisions based on reliable data, which is a fundamental aspect of statistics assignments.
Moments in Hypothesis Testing
In the realm of hypothesis testing, moments serve as the bedrock upon which statistical conclusions are built. The mean and variance, both fundamental moments, play pivotal roles in determining the statistical significance of hypotheses. When conducting hypothesis tests such as t-tests or analysis of variance (ANOVA), understanding the moments of sample data is essential. The mean acts as a measure of central tendency, allowing researchers to compare it with a population parameter and make inferences about the population mean. Meanwhile, the variance, depicting the spread of data points around the mean, informs researchers about the consistency of the data, enabling them to draw conclusions about the variability within the population. By utilizing moments in hypothesis testing, students can critically assess whether observed differences between samples are statistically significant, making these moments indispensable tools for rigorous scientific inquiry and data-driven decision-making.
Moments in Time Series Analysis
In Time Series Analysis, moments serve as essential tools for understanding the patterns and behaviors within sequential data points over time. By calculating moments like mean and variance, analysts can discern the central tendency and dispersion of time series data, providing valuable insights into trends and fluctuations. For instance, understanding the mean of a time series helps identify its long-term average behavior, while variance indicates the extent of deviation from this average, offering crucial information about volatility. Skewness and kurtosis moments are equally important, helping analysts assess the asymmetry of the data's distribution and the presence of extreme values over specific time intervals. Through moments, students studying Time Series Analysis gain the ability to model, forecast, and make informed decisions based on historical data, contributing significantly to fields like finance, economics, and environmental studies.
Skewness – The Measure of Asymmetry
Skewness, a fundamental concept in statistics, serves as a vital tool for understanding the asymmetry within a dataset. Essentially, it paints a clear picture of how the data is distributed, indicating whether it is perfectly symmetrical or exhibits a tilt to one side. In a symmetric distribution, the mean, median, and mode align perfectly. However, when skewness comes into play, these measures diverge, revealing intriguing insights. A positively skewed distribution, also known as right skewness, signifies that the data extends more towards higher values, pulling the mean higher than the median. Conversely, negative skewness, or left skewness, illustrates a scenario where the data clusters more towards lower values, causing the mean to be lower than the median. Mastering the nuances of skewness equips students with the ability to recognize biases, identify outliers, and make informed decisions, enhancing their analytical prowess in various statistical assignments and real-world applications.
What is Skewness?
Skewness is a crucial concept in statistics that describes the shape of a distribution. It provides insights into whether the data is symmetric or asymmetric. In a symmetric distribution, the mean, median, and mode are all the same. Skewness measures how far the distribution's tail extends in one direction, affecting the balance of the mean.
Positive Skewness
Positive skewness, also known as right skewness, occurs when the tail of the distribution extends more to the right (toward larger values). In such cases, the mean is greater than the median and the mode. Problems involving positive skewness often arise in fields like finance, where the distribution of returns on an investment might be positively skewed.
Negative Skewness
Negative skewness, or left skewness, is the opposite of positive skewness. Here, the tail of the distribution extends more to the left (toward smaller values). In negatively skewed distributions, the mean is less than the median and the mode. Negative skewness can be observed in scenarios like analyzing exam scores, where a few students score much lower than the majority.
Calculating Skewness
In statistics assignments, students may be required to calculate skewness. There are different formulas for skewness, but one of the common methods is using the third moment (skewness coefficient). Calculating skewness helps students assess the shape of a distribution and understand how it impacts data analysis.
Solving Statistical Problems with Skewness
Solving statistical problems with skewness is a nuanced process that empowers students to unravel the underlying patterns within data. Skewness, indicating the asymmetry of a distribution, plays a pivotal role in data analysis. When detecting biases in data, understanding the direction and magnitude of skewness is crucial, guiding students in making informed interpretations. Assignments often require students to apply this knowledge practically, especially in fields like market research or social sciences where skewed data is prevalent. Additionally, recognizing the impact of skewness on decision-making processes equips students with the ability to critically assess scenarios where skewed distributions influence real-world choices. Moreover, students learn to transform skewed data effectively, ensuring the accuracy of statistical models and analyses. By mastering the intricacies of skewness, students not only excel in their assignments but also acquire skills essential for making reliable, data-driven decisions in their future careers.
Detecting Biases in Data
Skewness is a useful tool for identifying biases in data. If a dataset is positively skewed, it might indicate an upward bias, suggesting that the mean is being pulled higher due to a few large values. In contrast, negative skewness can point to a downward bias. This knowledge is beneficial for students tackling assignments in fields like political polling or market research.
Impact on Decision-Making
Understanding skewness is crucial when making decisions based on data. Assignments may require students to analyze real-world scenarios where skewness affects the decision-making process. For instance, when assessing the distribution of income levels in a country, a positively skewed distribution could influence policy decisions related to income inequality.
Handling Skewed Data
Sometimes, students might need to transform skewed data to make it more suitable for analysis. For example, in finance, returns data is often positively skewed. Students may be tasked with transforming this data to meet the assumptions of statistical models, ensuring accurate analysis and decision-making.
Kurtosis – The Measure of Tailedness
Kurtosis, a crucial statistical measure, offers deep insights into the tailedness of a distribution. In essence, it evaluates the extent to which a dataset's tails deviate from the tails of a normal distribution. A positive kurtosis, termed leptokurtic, indicates that a dataset has fatter tails and a sharper peak, suggesting a higher likelihood of extreme values. This is of utmost importance in fields such as finance, where investors need to understand the potential risks associated with their portfolios. Conversely, a negative kurtosis, or platykurtic distribution, signifies thinner tails and a flatter peak, suggesting a lesser probability of extreme events. This distinction is invaluable in areas like quality control, ensuring that products or processes remain consistent. Moreover, the mesokurtic distribution, with kurtosis equal to zero, mirrors the tails and peak of a normal distribution, making it a common occurrence in various natural phenomena. Kurtosis, therefore, serves as a critical tool in determining the risk, volatility, and reliability of data, guiding decision-making processes in numerous real-world scenarios.
What is Kurtosis?
Kurtosis measures the tailedness or the thickness of the tails of a distribution. It tells us whether data points are concentrated around the mean or spread out more widely. Understanding kurtosis is vital for comprehending the potential for extreme values in a dataset.
Leptokurtic Distributions
A distribution with positive kurtosis, known as leptokurtic, has fatter tails and a sharper peak compared to a normal distribution. Leptokurtic distributions indicate that the data has a higher probability of extreme values than a normal distribution. Students might encounter leptokurtic distributions in assignments related to risk analysis, where understanding the likelihood of extreme events is crucial.
Platykurtic Distributions
Conversely, a distribution with negative kurtosis, called platykurtic, has thinner tails and a flatter peak than a normal distribution. Platykurtic distributions suggest that data points are less likely to deviate significantly from the mean. Assignments in fields such as quality control might involve analyzing data with platykurtic distributions to ensure consistency and uniformity.
Mesokurtic Distributions
A mesokurtic distribution, with kurtosis equal to zero, has tails and a peak similar to a normal distribution. Such distributions are often encountered in various natural phenomena. Understanding mesokurtic distributions is essential for students analyzing real-world data, as many statistical models assume data to be approximately normal.
Solving Statistical Problems with Kurtosis
Solving statistical problems with kurtosis opens a gateway to understanding the inherent risk and volatility in various datasets. In the realm of finance, kurtosis serves as a vital tool for assessing investment risks, allowing students to evaluate the likelihood of extreme market events. By calculating kurtosis, students can discern whether an investment portfolio is prone to substantial fluctuations, aiding in the development of more robust risk management strategies. Additionally, kurtosis plays a pivotal role in ensuring data accuracy, serving as an indicator for data anomalies and outliers. In assignments, students can utilize kurtosis to identify irregular patterns in datasets, guiding them to investigate further and maintain the integrity of their analyses. Moreover, when making predictions in fields such as predictive modeling and machine learning, understanding kurtosis is essential. It influences the choice of appropriate models, enabling students to build predictive algorithms that can handle datasets with heavy-tailed distributions, ultimately enhancing the accuracy of their forecasts.
Identifying Risk and Volatility
In finance and investment analysis, kurtosis helps assess the risk associated with an investment portfolio. High kurtosis indicates a higher probability of extreme returns, which might be desirable for high-risk, high-reward investments. Assignments in finance may require students to calculate kurtosis to evaluate the volatility of different assets and make informed investment decisions.
Ensuring Data Accuracy
Understanding kurtosis is vital when working with datasets in various fields. Students might need to identify errors in data entry or collection by analyzing kurtosis. Unusual kurtosis values can indicate data discrepancies, prompting further investigation. Assignments related to data quality assurance often involve assessing kurtosis to ensure the accuracy and reliability of the dataset.
Making Predictions with Kurtosis
In predictive modeling, kurtosis can influence the choice of appropriate statistical models. For example, a dataset with high kurtosis might require models that can handle heavy-tailed distributions, such as certain types of regression models or machine learning algorithms. Students working on predictive modeling assignments must consider kurtosis to build accurate and reliable models for making predictions.
Conclusion
In conclusion, moments, skewness, and kurtosis are indispensable tools in the world of statistics. Mastering these concepts empowers students to solve a wide range of statistical problems effectively. From comparing datasets and detecting outliers to making informed decisions and predictions, moments, skewness, and kurtosis provide valuable insights that are applicable across diverse fields. As students engage with assignments related to these concepts, they gain a deeper understanding of the data they analyze, enhancing their analytical skills and problem-solving abilities. By embracing the complexities of moments, skewness, and kurtosis, students pave the way for more accurate and meaningful statistical analysis, contributing significantly to the advancement of knowledge and decision-making in their respective fields.