A Comprehensive Guide to Statistical Analysis for SAS Assignments
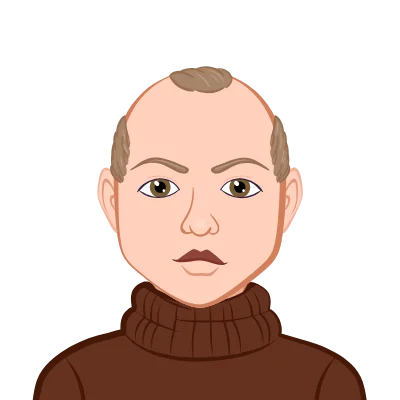
Statistical analysis plays a pivotal role in various fields, ranging from business and finance to healthcare and social sciences. It provides valuable insights into data, helping us make informed decisions and draw meaningful conclusions. For university students, particularly those working on SAS assignments, understanding statistical analysis is essential. In this comprehensive guide, we will delve into the core aspects of statistical analysis, including descriptive and inferential statistics, regression analysis, analysis of variance (ANOVA), multivariate analysis, and non-parametric statistical methods. By the end of this blog, you'll have the knowledge needed to complete your Statistical Analysis assignment using SAS with confidence.
Descriptive Statistics
Descriptive statistics are the foundation of data analysis. They provide a clear snapshot of data characteristics, making complex information more understandable. Measures like the mean, median, and variance offer insights into central tendencies and data variability. These statistics are essential tools for summarizing and presenting data effectively, helping us uncover patterns and trends in our datasets. Here are some key concepts:

Measures of Central Tendency
Measures of central tendency, such as the mean, median, and mode, are crucial in statistics. The mean represents the average of a dataset, providing a central reference point. The median identifies the middle value, which is resistant to extreme outliers. These statistics help us grasp the typical or central value in a set of data points, aiding in data interpretation.
- Mean: The mean, often referred to as the average, is a fundamental measure of central tendency in statistics. It's calculated by summing up all data points and dividing by the number of observations. The mean represents a typical value within a dataset and is sensitive to all data points. It's widely used for summarizing data and forming a basis for various statistical analyses.
- Median: The median, a key measure of central tendency, is the middle value in a dataset when arranged in ascending or descending order. Unlike the mean, it's not influenced by extreme outliers, making it a robust statistic for representing central data tendencies. This makes the median particularly useful when dealing with skewed data distributions or when you want to find a typical value that's resistant to outliers.
- Mode: The mode is a fundamental measure of central tendency in statistics. It identifies the most frequently occurring value in a dataset. Unlike the mean and median, the mode is particularly useful for categorical or discrete data, where identifying the most common category or value is essential. It provides valuable insights into the data's most prevalent characteristic, aiding in decision-making and analysis.
Measures of Dispersion
Measures of dispersion, including variance and standard deviation, complement central tendency measures. Variance quantifies how data points spread out from the mean, while standard deviation offers a more interpretable value as the square root of variance. These measures provide critical insights into data variability, helping us understand the data's spread and distribution.
- Variance: Variance is a vital statistical measure that quantifies the extent of data dispersion from the mean. A high variance indicates that data points are spread out widely, while a low variance suggests that they are clustered closely around the mean. It's a fundamental tool for understanding data variability, assisting in decision-making, risk assessment, and making informed comparisons between datasets or populations.
- Standard Deviation: Standard deviation is a key measure of dispersion in statistics. It quantifies the extent to which data points deviate from the mean. A high standard deviation indicates greater variability, while a low one suggests data points are closer to the mean. This valuable statistic provides a clear picture of data's spread and helps assess the reliability and consistency of data sets, aiding in decision-making and analysis.
- Range: The range is a straightforward yet informative measure of dispersion in statistics. It simply calculates the difference between the maximum and minimum values in a dataset. This measure offers a quick glimpse into the extent of data spread, making it a valuable tool for initial data exploration. However, it doesn't capture the nuances of data distribution, making it essential to combine with other measures for a comprehensive analysis.
Measures of Shape
Measures of shape, such as skewness and kurtosis, provide insights into the distribution of data. Skewness indicates whether the data is skewed to the left or right, revealing asymmetry. Kurtosis measures the concentration of data in the tails, showing if the distribution is more or less peaked than a normal distribution. These measures help characterize the shape and nature of data distributions.
- Skewness: Skewness is a vital statistical measure that reveals the asymmetry in the distribution of data. A positive skew indicates that the tail on the right side of the distribution is longer or fatter, while a negative skew implies the opposite. Understanding skewness aids in identifying potential outliers and assessing the overall shape of data, which is crucial for making accurate inferences and decisions in statistical analysis.
- Kurtosis: Kurtosis is a statistical measure that assesses the distribution's peakedness or flatness relative to a normal distribution. A high kurtosis indicates a more peaked distribution with heavy tails, while low kurtosis suggests a flatter distribution with lighter tails. It helps us understand the shape of data, detect outliers, and make assumptions about the data's underlying characteristics in statistical analysis.
Inferential Statistics
Inferential statistics, represented by hypothesis testing and confidence intervals, serve as the backbone of data-driven decision-making. Hypothesis testing involves making predictions and drawing conclusions about populations from samples, while confidence intervals provide a range of plausible values for population parameters. These tools help researchers make informed judgments and validate their hypotheses with statistical evidence. Key topics include:
Hypothesis Testing
Hypothesis testing is a pivotal component of inferential statistics, enabling researchers to evaluate assumptions about populations using sample data. It involves formulating a null hypothesis (H0) and an alternative hypothesis (H1), then assessing the evidence against the null hypothesis using p-values. This process empowers researchers to make data-driven decisions, determine statistical significance, and draw meaningful conclusions from their analyses.
- Null Hypothesis (H0): The null hypothesis, denoted as H0, is a foundational concept in hypothesis testing. It represents a statement of no effect or no difference, assuming that any observed variations in data are due to chance. Researchers use the null hypothesis as a starting point to assess whether there's sufficient statistical evidence to reject it in favor of an alternative hypothesis (H1).
- Alternative Hypothesis (H1): The alternative hypothesis, denoted as H1, is a core element of hypothesis testing in statistics. It represents the researcher's assertion or claim that there is a significant effect or relationship in the population. It stands in contrast to the null hypothesis (H0), which posits no effect or difference. Evaluating the evidence in favor of H1 is central to hypothesis testing and informs decisions in statistical analysis.
- p-value: The p-value is a crucial statistical measure in hypothesis testing. It quantifies the strength of evidence against the null hypothesis (H0). A small p-value (typically ≤ 0.05) suggests strong evidence to reject H0 in favor of the alternative hypothesis, indicating statistical significance. Conversely, a large p-value implies weak evidence against H0, suggesting no significant effect. P-values are essential for making informed decisions based on hypothesis testing results.
- Type I and Type II Errors: Understanding Type I and Type II errors is critical in hypothesis testing. Type I error occurs when a true null hypothesis is incorrectly rejected, leading to a false positive conclusion. Conversely, Type II error arises when a false null hypothesis is accepted, resulting in a false negative outcome. Researchers must strike a balance between these errors to ensure the validity of their statistical conclusions.
Confidence Intervals
Confidence intervals provide a range of likely values for population parameters, offering insights into the precision of sample estimates. The confidence level represents the probability that the interval captures the true parameter value. Widening the interval increases confidence but reduces precision. These intervals aid researchers in quantifying uncertainty and making robust inferences from sample data.
- Confidence Level: The confidence level is a crucial concept in inferential statistics, representing the probability that a calculated confidence interval contains the true population parameter. Common confidence levels are 95% or 99%, indicating the level of certainty in the interval's accuracy. Researchers use confidence levels to express the precision of their estimates and the associated margin of error.
- Margin of Error: The margin of error is a crucial concept in statistics, particularly in constructing confidence intervals. It quantifies the potential variability between a sample statistic and the true population parameter. A smaller margin of error indicates higher precision, while a larger margin indicates more uncertainty in the estimation process. Researchers use it to gauge the reliability of their results.
Regression Analysis
Regression analysis, a key component of statistical modeling, investigates relationships between variables. Linear regression predicts a continuous outcome based on one or more predictors, while logistic regression is used for binary outcomes. It's an indispensable tool in understanding, predicting, and modeling complex real-world scenarios, providing valuable insights for decision-making and research. Key types of regression include:
Linear Regression
Linear regression assumes a linear relationship and aims to find the best-fitting line (the regression equation) that explains the variance in the dependent variable. This method helps researchers make predictions and understand how changes in independent variables impact the outcome, making it an essential tool in data analysis and decision-making.
Logistic Regression
Logistic regression is a powerful statistical technique used when the outcome variable is binary or categorical. It models the probability of an event occurring, making it invaluable in fields like healthcare, finance, and marketing. By estimating the odds ratio, logistic regression helps us understand the impact of predictors on the probability of success. Its versatility makes it a fundamental tool for predictive modeling and risk assessment.
Analysis of Variance (ANOVA) and Multivariate Analysis
Analysis of Variance (ANOVA) and multivariate analysis are advanced statistical techniques. ANOVA compares means between multiple groups, providing insights into group differences. Multivariate analysis encompasses techniques like PCA, factor analysis, and cluster analysis, revealing patterns, relationships, and underlying factors within complex datasets. These tools are essential for understanding the intricacies of multivariable data in various fields.
Analysis of Variance (ANOVA)
Analysis of Variance (ANOVA) assesses whether the group variances are significantly different from one another, aiding in understanding the impact of categorical variables on a continuous outcome. ANOVA can be one-way for single factors or two-way for interactions, providing valuable insights into group differences and guiding further post-hoc analyses when needed.
Multivariate Analysis
Multivariate analysis is a sophisticated statistical approach that explores relationships among multiple variables simultaneously. Principal Component Analysis (PCA) reduces data dimensionality, simplifying interpretation. Factor analysis identifies latent variables influencing observed variables. Cluster analysis groups similar data points based on shared characteristics. These techniques are invaluable for uncovering hidden patterns, reducing data complexity, and aiding decision-making in fields like psychology, biology, and market research.
Non-parametric Statistical Methods
Non-parametric statistical methods offer alternatives when data doesn't meet parametric assumptions. The Mann-Whitney U Test, akin to a t-test, assesses differences between two independent groups. The Wilcoxon Signed-Rank Test is a non-parametric counterpart for paired samples. Kruskal-Wallis Test extends the analysis to more groups. These methods provide robust solutions for various research scenarios and help maintain statistical validity.
Conclusion
Statistical analysis is a vital skill for university students working on SAS assignments. In this comprehensive guide, we've covered essential topics, including descriptive and inferential statistics, regression analysis, ANOVA, multivariate analysis, and non-parametric methods. Mastering these concepts will empower you to analyze data effectively, make informed decisions, and excel in your SAS assignments. Remember to practice and apply these techniques to real-world data to solidify your understanding and become a proficient SAS user.