Multicollinearity Demystified: A Student's Guide to Conquer Statistics Assignments

In the intricate world of statistics, the term "multicollinearity" often strikes fear into the hearts of students. However, fear not, for this phenomenon is not an insurmountable challenge but a puzzle waiting to be solved. If you need help with your Multicollinearity assignment, "Multicollinearity Demystified: A Student's Guide to Conquer Statistics Assignments" serves as a beacon of light, illuminating the path for students to navigate this complex terrain with confidence and clarity. This comprehensive guide is meticulously crafted to unravel the mysteries of multicollinearity, offering students a robust toolkit of analytical strategies and problem-solving techniques. Through in-depth explanations and real-world examples, this guide empowers students to understand the nuances of multicollinearity, equipping them with the knowledge and skills needed to conquer even the most daunting statistics assignments.
With a focus on demystification, this guide goes beyond the surface, delving deep into the core concepts of multicollinearity. By breaking down intricate statistical jargon into accessible language and offering step-by-step approaches, students are not only equipped to identify multicollinearity but also adept at resolving it effectively. Whether it's through correlation matrix analysis, variance inflation factor assessments, or advanced techniques like regularization, this guide provides students with a holistic understanding of multicollinearity. Armed with this knowledge, students can approach their statistics assignments with confidence, transforming what was once a daunting challenge into a rewarding learning experience.
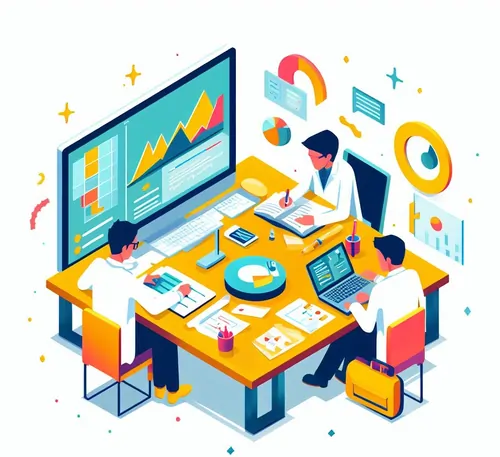
Identifying Multicollinearity: A Critical Analysis
In the intricate realm of statistical analysis, identifying multicollinearity stands as a critical challenge demanding meticulous scrutiny. Through a thorough Correlation Matrix Analysis, researchers can unveil the underlying relationships between variables, discerning the nuances of their interconnections. Variance Inflation Factor (VIF) Analysis, on the other hand, offers a quantitative lens, providing precise measurements of correlation intensity. As students delve into Tolerance Analysis, they gain insights into the unique contribution of each variable, unraveling the layers of multicollinearity's complexity. Eigenvalue Analysis acts as the final piece of this diagnostic puzzle, illuminating subtle patterns within the data. Together, these analyses equip students with the discerning eye needed to identify multicollinearity, laying the foundation for effective problem-solving in their statistics assignments.
Correlation Matrix Analysis
Correlation Matrix Analysis, a fundamental technique in the realm of statistics, provides students with valuable insights into the relationships between variables. By meticulously examining the correlation coefficients within a matrix, students can discern the strength and direction of these relationships. This method enables them to identify highly correlated variables, a crucial step in diagnosing multicollinearity. Moreover, understanding the context of these correlations is equally essential; it aids students in making informed decisions about which variables to retain, modify, or eliminate in their regression models. Through Correlation Matrix Analysis, students gain a deep understanding of the intricate web of interconnections within their data, empowering them to navigate the complexities of multicollinearity effectively in their statistics assignments.
Variance Inflation Factor (VIF) Analysis
Variance Inflation Factor (VIF) analysis stands as a pivotal technique for students aiming to unravel the complexities of multicollinearity. VIF quantifies the extent to which an independent variable is correlated with other variables in a regression model, indicating the presence of multicollinearity. By calculating VIF values for each predictor, students gain profound insights into the interrelationships among variables. A high VIF, typically exceeding 10, signifies a problematic level of multicollinearity, demanding immediate attention. Students are thereby equipped with a precise measure to identify which variables are contributing significantly to the multicollinearity issue, enabling them to make informed decisions such as variable elimination or applying advanced statistical methods to refine their regression models. Mastering VIF analysis empowers students to enhance the accuracy and reliability of their statistical analyses, making it an indispensable tool in their academic journey.
Tolerance Analysis
Tolerance analysis, a vital diagnostic tool in the realm of statistics, plays a pivotal role in assessing multicollinearity among independent variables. Unlike other methods, tolerance provides a unique perspective by indicating the proportion of variance in an independent variable that is not explained by other predictors. When tolerance values are low, typically below 0.1, it signals a high degree of multicollinearity. This means that the variable in question is heavily influenced by other variables in the model, compromising the reliability of regression coefficients. By understanding tolerance values, students can pinpoint specific variables contributing significantly to multicollinearity, allowing them to make informed decisions such as removing redundant variables or exploring more advanced techniques to improve the robustness of their statistical models.
Eigenvalue Analysis
Eigenvalue analysis, a sophisticated technique in multivariate statistics, offers profound insights into the intricate relationships among variables. In the context of multicollinearity, eigenvalues serve as the numeric indicators of the correlation structure. By evaluating eigenvalues derived from the correlation matrix, students can discern the presence and magnitude of multicollinearity within their data. Eigenvalues greater than one signify the existence of correlated variables, prompting students to reconsider their model’s composition. This analytical approach not only highlights problematic variables but also aids in reshaping regression models, fostering a deeper understanding of the intricate web of relationships and empowering students to construct more robust statistical analyses. Mastering eigenvalue analysis equips students with the ability to dissect complex datasets, ensuring their statistical assignments stand on a foundation of rigorous analysis and accurate interpretations.
Overcoming Multicollinearity Challenges: Strategies and Techniques
Navigating the intricate landscape of multicollinearity demands strategic finesse and adept problem-solving. One of the most effective strategies lies in the realm of feature selection and dimensionality reduction. By employing techniques such as backward elimination, forward selection, or the powerful tool of stepwise regression, students can meticulously curate their variables, ensuring only the most significant ones find their place in the model. Additionally, regularization techniques like Lasso and Ridge regression offer a robust shield against multicollinearity, introducing penalty terms that discourage the undue influence of correlated variables. For those facing limited datasets, data augmentation and resampling techniques emerge as saviors, injecting diversity into the data pool and mitigating multicollinearity's impact. Furthermore, fostering a spirit of collaboration and communication within the academic community proves invaluable; shared insights and innovative solutions from peers and professionals often illuminate the path to overcoming these challenges effectively.
Feature Selection and Dimensionality Reduction
In the realm of statistics, mastering the art of feature selection and dimensionality reduction is akin to sculpting a masterpiece from a block of marble. Feature selection, a meticulous process, involves choosing the most relevant variables that significantly impact the model's outcomes. By employing techniques like backward elimination, forward selection, or stepwise regression, students meticulously refine their models, discarding variables that add noise rather than clarity. On the other hand, dimensionality reduction techniques such as Principal Component Analysis (PCA) transform high-dimensional datasets into a condensed, manageable form. This process not only mitigates multicollinearity but also simplifies the complexity of the analysis, allowing students to focus on the core variables driving meaningful insights. As students adeptly navigate these techniques, they not only conquer multicollinearity but also elevate their statistical prowess, sculpting nuanced and accurate analyses in their assignments.
Regularization Techniques
In the realm of statistics, Regularization Techniques stand as formidable guardians against the challenges posed by multicollinearity. Lasso and Ridge regression, two prominent regularization methods, introduce penalty terms into the regression equation, effectively constraining the impact of highly correlated variables. Lasso, with its ability to shrink coefficients to zero, acts as a powerful variable selection tool, encouraging sparsity and simplifying models. On the other hand, Ridge regression mitigates multicollinearity by penalizing large coefficients, ensuring a balance between bias and variance. By incorporating these techniques, students can fine-tune their regression models, preventing multicollinearity-induced errors and enhancing the predictive accuracy of their statistical analyses. Regularization not only refines the precision of predictions but also equips students with advanced tools to confidently navigate the intricate landscape of statistics assignments.
Data Augmentation and Resampling
data augmentation and resampling techniques emerge as indispensable strategies for students seeking to bolster the depth of their insights. Data augmentation involves the strategic expansion of the existing dataset through techniques like mirroring, rotation, or adding noise, thereby diversifying the dataset and providing a more comprehensive view of the underlying patterns. On the other hand, resampling methods, such as bootstrapping, empower students to create multiple datasets by drawing repeated samples from the original data. These diversified datasets not only fortify statistical models but also allow students to explore the nuances of multicollinearity across various data subsets. By embracing these techniques, students gain a nuanced understanding of the impact of multicollinearity on different data scenarios, equipping themselves with a robust analytical arsenal for tackling complex statistics assignments.
Communication and Collaboration
Effective communication and collaboration are the cornerstones of academic success in statistics. Engaging in open dialogue with fellow students, professors, and online communities provides a platform for sharing insights, discussing challenges, and exploring innovative solutions. By actively participating in discussions and seeking guidance from peers and professionals, students can broaden their perspectives and gain fresh insights into the complexities of multicollinearity. Collaborative efforts foster an environment of mutual learning, allowing students to exchange ideas, strategies, and real-world experiences. Embracing the power of communication and collaboration not only enhances students’ problem-solving skills but also nurtures a supportive network that empowers them to tackle even the most daunting statistics assignments with confidence and proficiency.
Conclusion
In the realm of statistics, mastering the art of diagnosing and addressing multicollinearity is indispensable for students striving for excellence in their assignments. Armed with a deep understanding of correlation matrix analysis, VIF, tolerance, and eigenvalue analysis, students can pinpoint multicollinearity issues with precision. Implementing strategic solutions such as feature selection, regularization techniques, and data augmentation empowers students to overcome these challenges effectively.
As students navigate the intricate landscape of statistics assignments, embracing collaboration and seeking guidance from the broader academic community proves invaluable. By harnessing the collective wisdom of peers and professionals, students can refine their diagnostic skills, paving the way for accurate, reliable, and insightful statistical analyses. Multicollinearity, once a daunting obstacle, becomes an opportunity for growth and learning, shaping students into adept statisticians capable of tackling real-world data challenges with confidence and expertise.