Data Relationships: A Student's Ultimate Guide to Multiple Correlation in Statistics
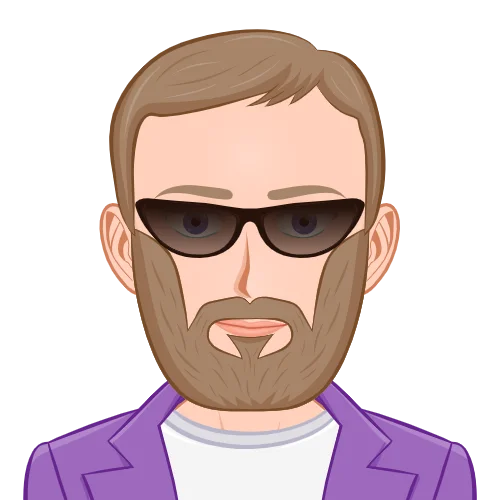
Understanding the intricate web of relationships within data sets is paramount, and Multiple Correlation stands as a formidable tool in unraveling this complexity. Welcome to "Data Relationships: A Student's Ultimate Guide to Multiple Correlation in Statistics" the perfect resource to help you write your correlation assignment. This comprehensive guide is meticulously crafted to empower students with the knowledge and skills necessary to navigate the intricate pathways of statistical relationships. Whether you're a novice venturing into the world of data analysis or a seasoned learner seeking to deepen your understanding, this guide is your compass.
Within these pages, you will embark on a journey that demystifies the concept of Multiple Correlation. From grasping the fundamental principles to exploring advanced applications, this guide provides a holistic perspective. Delving into the very essence of Multiple Correlation, you will uncover not only its theoretical underpinnings but also its practical implications in various fields. Through clear explanations, real-world examples, and practical tips, this guide equips you to discern patterns, make predictions, and draw meaningful conclusions from complex data sets. Emphasizing hands-on practice, critical thinking, and the utilization of cutting-edge statistical tools, this guide serves as a steadfast companion in your quest to master the art and science of data relationships. Join us as we unravel the mysteries hidden within the numbers, empowering you to decode the language of data and become a proficient statistician.
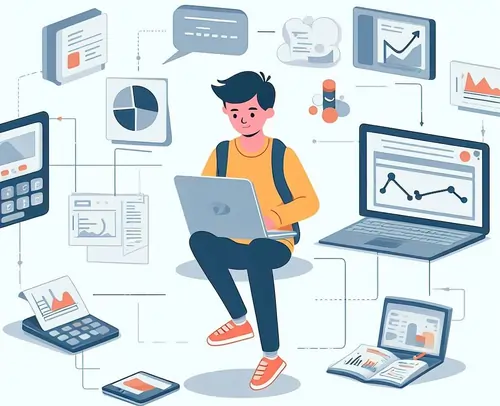
Exploring the Basics of Multiple Correlation
the fundamental concept of Multiple Correlation is essential for any student diving into the world of statistics. At its core, Multiple Correlation delves into the intricate relationships between a single dependent variable and multiple independent variables. Unlike simple correlation, which examines connections between two variables, Multiple Correlation takes a broader perspective, allowing researchers to unravel the complex web of influences that several factors can have on an outcome. Represented by an "R" value, Multiple Correlation provides insights into both the strength and directionality of these relationships. By comprehending the nuances of this statistical tool, students gain the ability to decipher multifaceted datasets, make accurate predictions, and draw meaningful conclusions about real-world phenomena. This foundational knowledge forms the basis for more advanced statistical analyses, making it a cornerstone for students navigating the intricacies of statistical assignments.
What is Multiple Correlation?
Multiple Correlation, also known as multiple regression correlation, is a statistical method used to explore the relationship between a dependent variable and two or more independent variables. Unlike simple correlation, which examines the relationship between two variables, multiple correlation analyzes how multiple independent variables jointly influence a single dependent variable.
Multiple Correlation is expressed as an "R" value, ranging from -1 to 1. An R-value closer to 1 indicates a strong positive correlation, while a value closer to -1 indicates a strong negative correlation. A value near 0 suggests a weak or no correlation between the variables.
Why is Multiple Correlation Important?
Multiple Correlation is a powerful tool in various fields such as economics, psychology, biology, and social sciences. Researchers and analysts use it to predict outcomes, identify significant variables, and understand complex relationships within datasets. By examining multiple factors simultaneously, Multiple Correlation provides a more nuanced understanding of real-world phenomena.
Assumptions and Requirements for Multiple Correlation
Before applying Multiple Correlations, it's essential to ensure that the data meets certain assumptions. These include linearity, independence of observations, homoscedasticity (constant variance), and normality of residuals. Violation of these assumptions can lead to inaccurate results, emphasizing the importance of thorough data preparation and analysis.
Steps to Perform Multiple Correlation Analysis
Performing Multiple Correlation analysis involves several steps:
- Data Collection and Preparation: Gather the relevant data for the dependent and independent variables. Clean and preprocess the data to ensure accuracy and consistency.
- Variable Selection: Choose the independent variables that you believe have an impact on the dependent variable. Consider factors such as theoretical relevance and prior research.
- Regression Model: Build a regression equation using the selected independent variables. The equation will help predict the values of the dependent variable based on the chosen predictors.
- Analysis and Interpretation: Analyze the regression output, paying attention to coefficients, R-squared value, and p-values. Interpret the results in the context of the research question and draw meaningful conclusions.
Advanced Applications of Multiple Correlation
Now that we have covered the basics, let's explore some advanced applications of Multiple Correlation that can enhance your statistical analysis skills. Multiple Correlation proves indispensable in various fields. One significant area is predictive modeling, which serves as the cornerstone for creating robust and accurate models. By delving into the relationships among multiple variables, analysts can develop sophisticated predictive algorithms. Additionally, Multiple Correlation plays a pivotal role in experimental design, guiding researchers as they dissect the effects of numerous independent variables on an experiment's outcome. Its ability to unravel complex relationships shines brightly in this context, enabling scientists to draw nuanced conclusions. Furthermore, in the domain of business analytics, Multiple Correlation is instrumental in market research, helping companies understand the intricate web of factors influencing consumer behavior. By leveraging Multiple Correlation techniques, businesses gain insights that drive strategic decisions, ultimately enhancing their competitive edge in the market.
Multicollinearity and its Impact on Multiple Correlations
Multicollinearity occurs when independent variables in a regression model are highly correlated. This can lead to unreliable coefficient estimates and difficulties in interpreting the model. Identifying and addressing multicollinearity is crucial for accurate Multiple Correlation analysis. Techniques like variance inflation factor (VIF) help assess the severity of multicollinearity, enabling researchers to make informed decisions about variable inclusion.
Multiple Correlation in Predictive Modeling
Multiple Correlation plays a pivotal role in predictive modeling. By understanding the relationships between multiple variables, analysts can create robust models for prediction and forecasting. Techniques like stepwise regression and cross-validation enhance the accuracy of predictive models, ensuring they generalize well to new, unseen data.
Multiple Correlation in Experimental Design
In experimental design, Multiple Correlation helps researchers assess the impact of multiple independent variables on the outcome of an experiment. By controlling and manipulating different factors simultaneously, scientists can gain valuable insights into complex phenomena. Multiple Correlation guides the analysis of such experiments, enabling researchers to draw meaningful conclusions about the effects of various variables on the dependent variable.
Limitations and Challenges in Multiple Correlation Analysis
While Multiple Correlation is a powerful tool, it is not without limitations. One common challenge is the potential for overfitting, where the model fits the training data too closely and performs poorly on new data. Regularization techniques, such as ridge regression and Lasso regression, mitigate overfitting by penalizing overly complex models. Additionally, the quality of results heavily depends on the choice of independent variables. Including irrelevant or redundant variables can lead to misleading conclusions, emphasizing the importance of careful variable selection.
Practical Tips for Mastering Multiple Correlation
Mastering Multiple Correlations requires a combination of practical skills and analytical thinking. One effective strategy is to immerse yourself in hands-on practice. Engage with real-world datasets, apply regression models, and meticulously interpret the outcomes. This hands-on experience not only reinforces your theoretical knowledge but also hones your ability to discern meaningful patterns within data. Additionally, seeking guidance and resources from professors, classmates, and online platforms can provide valuable insights and alternative perspectives on solving complex problems. Utilizing statistical software like R, Python, or specialized packages streamlines the analysis process, allowing you to focus on understanding the results. Furthermore, cultivate critical thinking skills by approaching assignments with a discerning eye. Understand the context, identify variables, and question assumptions, enabling you to approach Multiple Correlation problems strategically. By combining practical application, guidance, software proficiency, and critical thinking, you'll develop a robust foundation for mastering Multiple Correlations and excelling in your statistics assignments. To excel in solving statistics assignments involving Multiple Correlations, consider the following practical tips:
Embrace Hands-on Practice
Practice is key to mastering Multiple Correlations. Work on real-world datasets, apply regression models and interpret the results. The more hands-on experience you gain, the more confident you'll become in tackling complex assignments.
Seek Guidance and Resources
Don't hesitate to seek help from your professors, classmates, or online resources. There are numerous tutorials, textbooks, and online forums dedicated to statistics and data analysis. Engage with these resources to enhance your understanding of Multiple Correlations.
Utilize Statistical Software
Familiarize yourself with statistical software like R, Python (with libraries such as NumPy, pandas, and scikit-learn), or software packages like SPSS and SAS. These tools simplify the process of performing Multiple Correlation analysis and interpreting the results.
Practice Critical Thinking
Approach statistics assignments with a critical mindset. Understand the context of the problem, identify the variables at play, and think critically about the relationships between them. Developing a logical approach to problem-solving will significantly enhance your ability to analyze and interpret Multiple Correlation results accurately.
Conclusion
In conclusion, Multiple Correlation is a potent statistical technique that unlocks deeper insights into complex relationships within datasets. By mastering its principles, applications, and best practices, you can confidently tackle statistics assignments and contribute meaningfully to various fields that rely on data-driven decision-making. Embrace the challenges, practice diligently, and remember that with a solid understanding of Multiple Correlations, you're equipped to unravel the mysteries hidden within the numbers.